Pattern-wise Transparent Sequential Recommendation
CoRR(2024)
摘要
A transparent decision-making process is essential for developing reliable
and trustworthy recommender systems. For sequential recommendation, it means
that the model can identify critical items asthe justifications for its
recommendation results. However, achieving both model transparency and
recommendation performance simultaneously is challenging, especially for models
that take the entire sequence of items as input without screening. In this
paper,we propose an interpretable framework (named PTSR) that enables a
pattern-wise transparent decision-making process. It breaks the sequence of
items into multi-level patterns that serve as atomic units for the entire
recommendation process. The contribution of each pattern to the outcome is
quantified in the probability space. With a carefully designed pattern
weighting correction, the pattern contribution can be learned in the absence of
ground-truth critical patterns. The final recommended items are those items
that most critical patterns strongly endorse. Extensive experiments on four
public datasets demonstrate remarkable recommendation performance, while case
studies validate the model transparency. Our code is available at
https://anonymous.4open.science/r/PTSR-2237.
更多查看译文
AI 理解论文
溯源树
样例
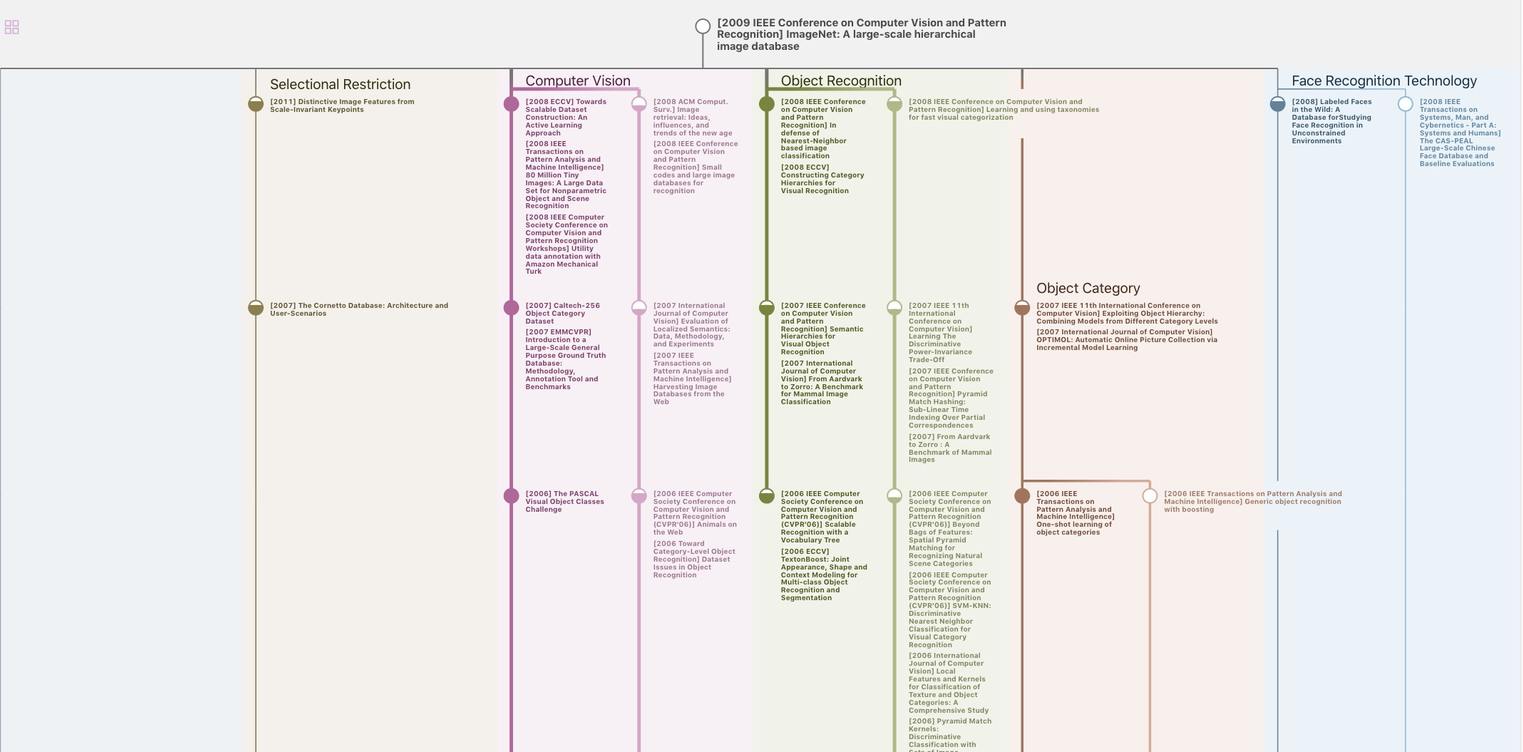
生成溯源树,研究论文发展脉络
Chat Paper
正在生成论文摘要