Doubly Robust Inference in Causal Latent Factor Models
CoRR(2024)
Abstract
This article introduces a new framework for estimating average treatment
effects under unobserved confounding in modern data-rich environments featuring
large numbers of units and outcomes. The proposed estimator is doubly robust,
combining outcome imputation, inverse probability weighting, and a novel
cross-fitting procedure for matrix completion. We derive finite-sample and
asymptotic guarantees, and show that the error of the new estimator converges
to a mean-zero Gaussian distribution at a parametric rate. Simulation results
demonstrate the practical relevance of the formal properties of the estimators
analyzed in this article.
MoreTranslated text
AI Read Science
Must-Reading Tree
Example
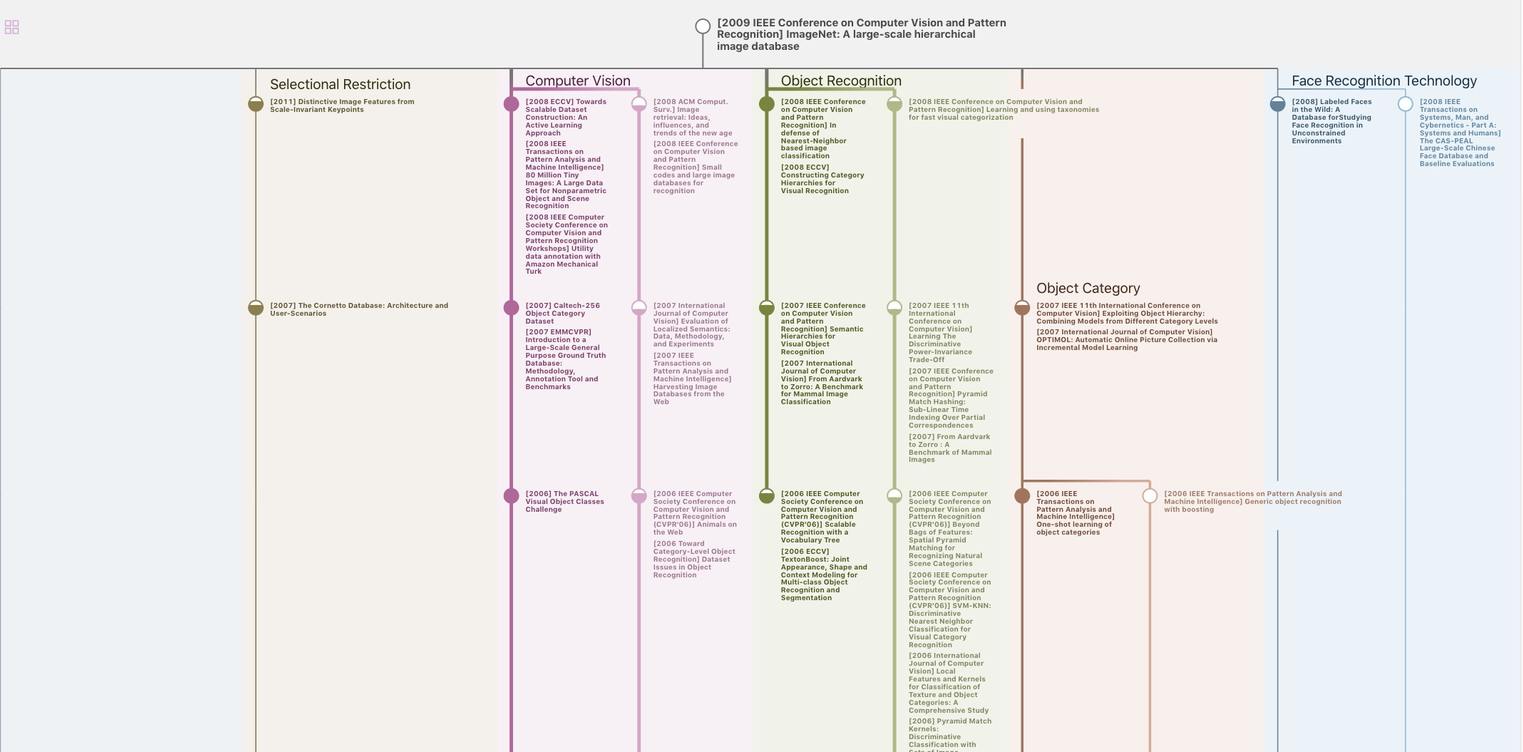
Generate MRT to find the research sequence of this paper
Chat Paper
Summary is being generated by the instructions you defined