Evaluating Image Review Ability of Vision Language Models
CoRR(2024)
摘要
Large-scale vision language models (LVLMs) are language models that are
capable of processing images and text inputs by a single model. This paper
explores the use of LVLMs to generate review texts for images. The ability of
LVLMs to review images is not fully understood, highlighting the need for a
methodical evaluation of their review abilities. Unlike image captions, review
texts can be written from various perspectives such as image composition and
exposure. This diversity of review perspectives makes it difficult to uniquely
determine a single correct review for an image. To address this challenge, we
introduce an evaluation method based on rank correlation analysis, in which
review texts are ranked by humans and LVLMs, then, measures the correlation
between these rankings. We further validate this approach by creating a
benchmark dataset aimed at assessing the image review ability of recent LVLMs.
Our experiments with the dataset reveal that LVLMs, particularly those with
proven superiority in other evaluative contexts, excel at distinguishing
between high-quality and substandard image reviews.
更多查看译文
AI 理解论文
溯源树
样例
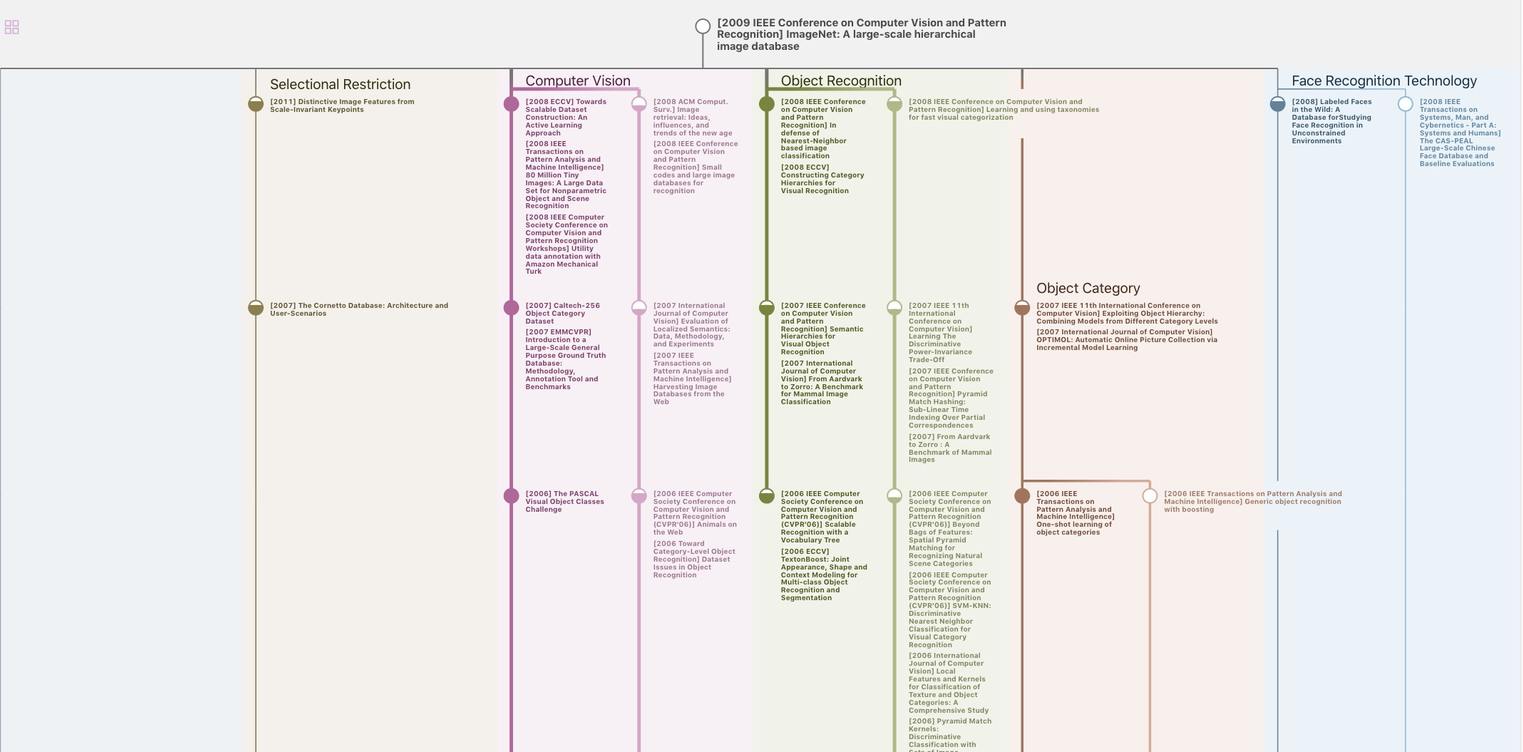
生成溯源树,研究论文发展脉络
Chat Paper
正在生成论文摘要