End-to-end Supervised Prediction of Arbitrary-size Graphs with Partially-Masked Fused Gromov-Wasserstein Matching
CoRR(2024)
摘要
We present a novel end-to-end deep learning-based approach for Supervised
Graph Prediction (SGP). We introduce an original Optimal Transport (OT)-based
loss, the Partially-Masked Fused Gromov-Wasserstein loss (PM-FGW), that allows
to directly leverage graph representations such as adjacency and feature
matrices. PM-FGW exhibits all the desirable properties for SGP: it is node
permutation invariant, sub-differentiable and handles graphs of different sizes
by comparing their padded representations as well as their masking vectors.
Moreover, we present a flexible transformer-based architecture that easily
adapts to different types of input data. In the experimental section, three
different tasks, a novel and challenging synthetic dataset (image2graph) and
two real-world tasks, image2map and fingerprint2molecule - showcase the
efficiency and versatility of the approach compared to competitors.
更多查看译文
AI 理解论文
溯源树
样例
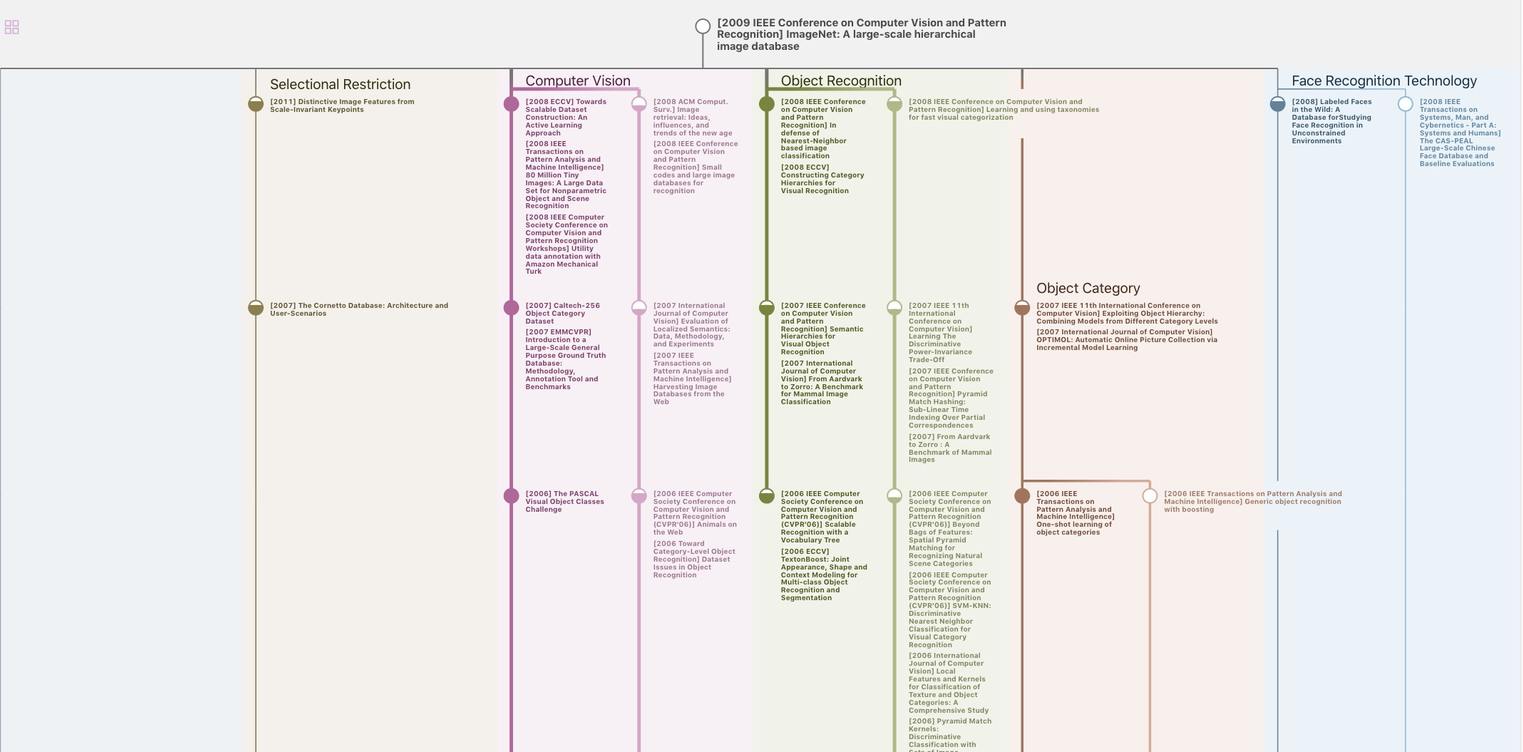
生成溯源树,研究论文发展脉络
Chat Paper
正在生成论文摘要