Is Open-Source There Yet? A Comparative Study on Commercial and Open-Source LLMs in Their Ability to Label Chest X-Ray Reports
CoRR(2024)
摘要
Introduction: With the rapid advances in large language models (LLMs), there
have been numerous new open source as well as commercial models. While recent
publications have explored GPT-4 in its application to extracting information
of interest from radiology reports, there has not been a real-world comparison
of GPT-4 to different leading open-source models.
Materials and Methods: Two different and independent datasets were used. The
first dataset consists of 540 chest x-ray reports that were created at the
Massachusetts General Hospital between July 2019 and July 2021. The second
dataset consists of 500 chest x-ray reports from the ImaGenome dataset. We then
compared the commercial models GPT-3.5 Turbo and GPT-4 from OpenAI to the
open-source models Mistral-7B, Mixtral-8x7B, Llama2-13B, Llama2-70B,
QWEN1.5-72B and CheXbert and CheXpert-labeler in their ability to accurately
label the presence of multiple findings in x-ray text reports using different
prompting techniques.
Results: On the ImaGenome dataset, the best performing open-source model was
Llama2-70B with micro F1-scores of 0.972 and 0.970 for zero- and few-shot
prompts, respectively. GPT-4 achieved micro F1-scores of 0.975 and 0.984,
respectively. On the institutional dataset, the best performing open-source
model was QWEN1.5-72B with micro F1-scores of 0.952 and 0.965 for zero- and
few-shot prompting, respectively. GPT-4 achieved micro F1-scores of 0.975 and
0.973, respectively.
Conclusion: In this paper, we show that while GPT-4 is superior to
open-source models in zero-shot report labeling, the implementation of few-shot
prompting can bring open-source models on par with GPT-4. This shows that
open-source models could be a performant and privacy preserving alternative to
GPT-4 for the task of radiology report classification.
更多查看译文
AI 理解论文
溯源树
样例
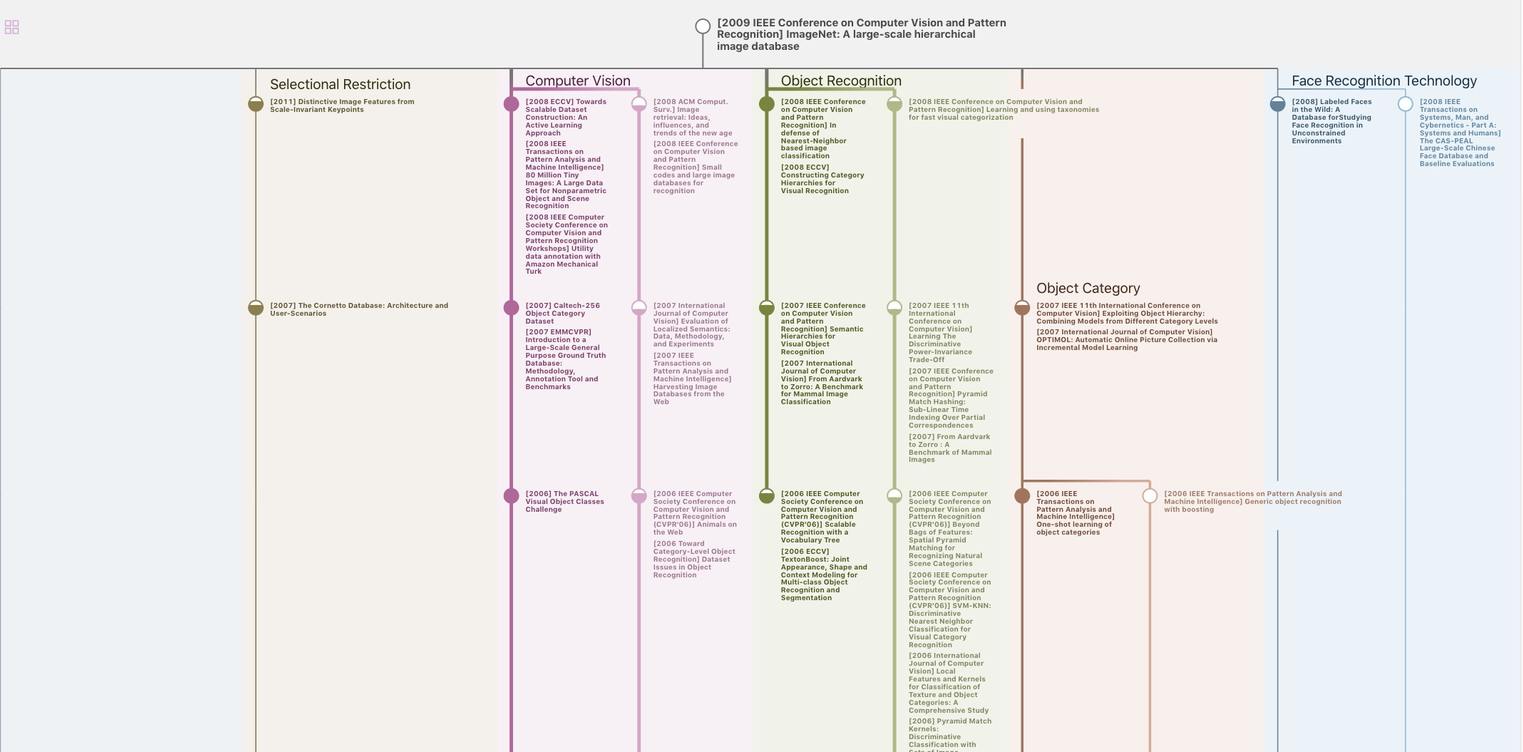
生成溯源树,研究论文发展脉络
Chat Paper
正在生成论文摘要