HunFlair2 in a cross-corpus evaluation of biomedical named entity recognition and normalization tools
CoRR(2024)
摘要
With the exponential growth of the life science literature, biomedical text
mining (BTM) has become an essential technology for accelerating the extraction
of insights from publications. Identifying named entities (e.g., diseases,
drugs, or genes) in texts and their linkage to reference knowledge bases are
crucial steps in BTM pipelines to enable information aggregation from different
documents. However, tools for these two steps are rarely applied in the same
context in which they were developed. Instead, they are applied in the wild,
i.e., on application-dependent text collections different from those used for
the tools' training, varying, e.g., in focus, genre, style, and text type. This
raises the question of whether the reported performance of BTM tools can be
trusted for downstream applications. Here, we report on the results of a
carefully designed cross-corpus benchmark for named entity extraction, where
tools were applied systematically to corpora not used during their training.
Based on a survey of 28 published systems, we selected five for an in-depth
analysis on three publicly available corpora encompassing four different entity
types. Comparison between tools results in a mixed picture and shows that, in a
cross-corpus setting, the performance is significantly lower than the one
reported in an in-corpus setting. HunFlair2 showed the best performance on
average, being closely followed by PubTator. Our results indicate that users of
BTM tools should expect diminishing performances when applying them in the wild
compared to original publications and show that further research is necessary
to make BTM tools more robust.
更多查看译文
AI 理解论文
溯源树
样例
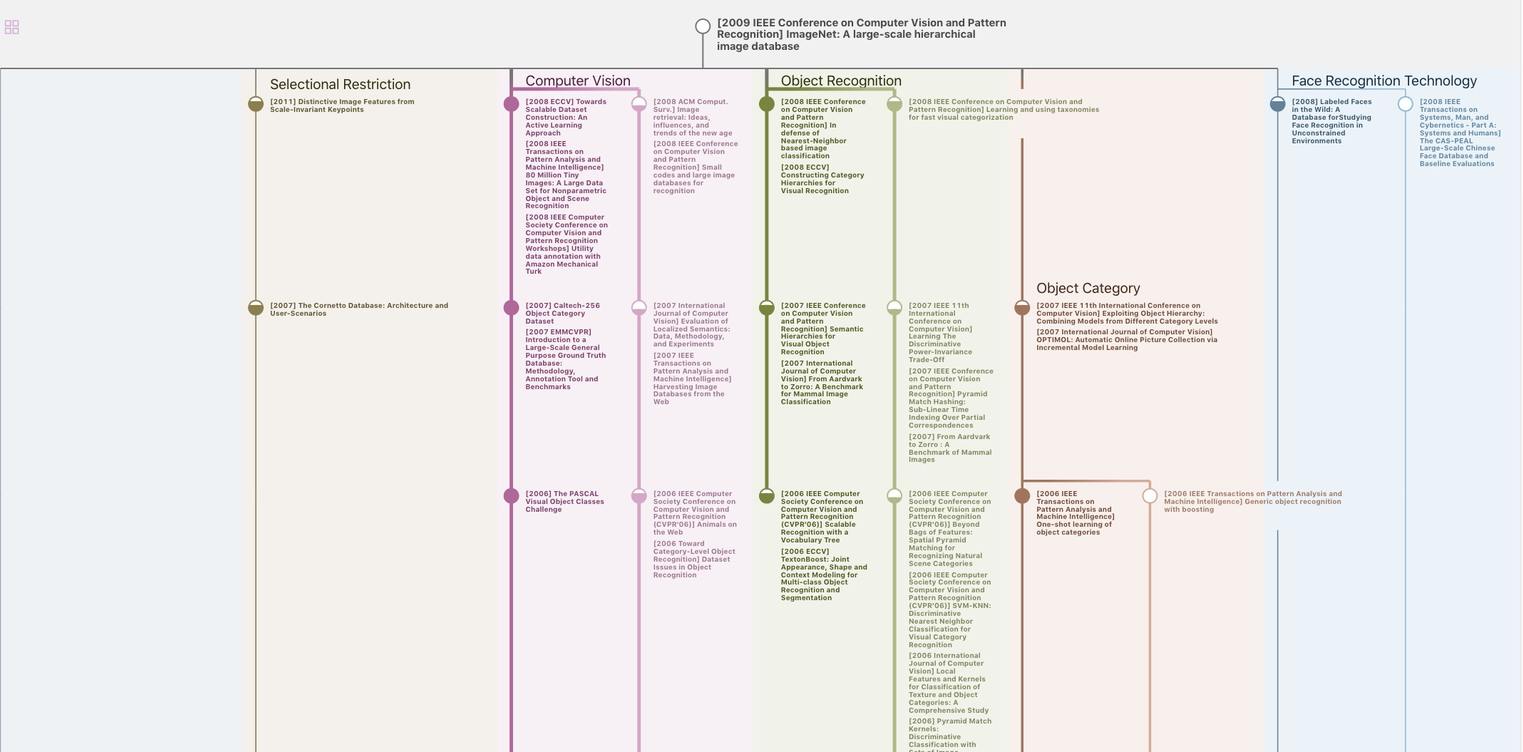
生成溯源树,研究论文发展脉络
Chat Paper
正在生成论文摘要