Unveiling community structures in static networks through graph variational Bayes with evolution information
NEUROCOMPUTING(2024)
摘要
With the rapid development of graph variational Bayes theory, some representative community detection methods have been proposed. Although these methods are well designed, they are limited by the inherent constraints of static networks. Therefore, how to re-examine the information in static networks from a new perspective becomes a challenge. To this end, in this paper, we propose EG-VGAE and its variant EGC-VGAE, going beyond the constraints of static networks from a new perspective. Specifically, we first demonstrate that the evolution relations on static networks can be simulated reasonably. And then, our EG-VGAE method combines evolution information with static network information to realize a fine-grained propagation of local low -frequency signals to global low -frequency signals, thereby improving the accuracy of community assignment for each node. Building on this progress, our method EGC-VGAE imposes the smoothness constraint on adjacent slices, significantly enhancing the sensitivity of the method to evolution information and mitigating the impact of network noise. The comprehensive experimental results on real static networks well validate that our methods outperform state-of-the-art methods in most cases. The code is available at https://github.com/GDM-SCNU/EG-VGAE.
更多查看译文
关键词
Variational graph autoencoder,Community detection,Evolution information,Mixture of Gaussian distributions,K-core decomposition
AI 理解论文
溯源树
样例
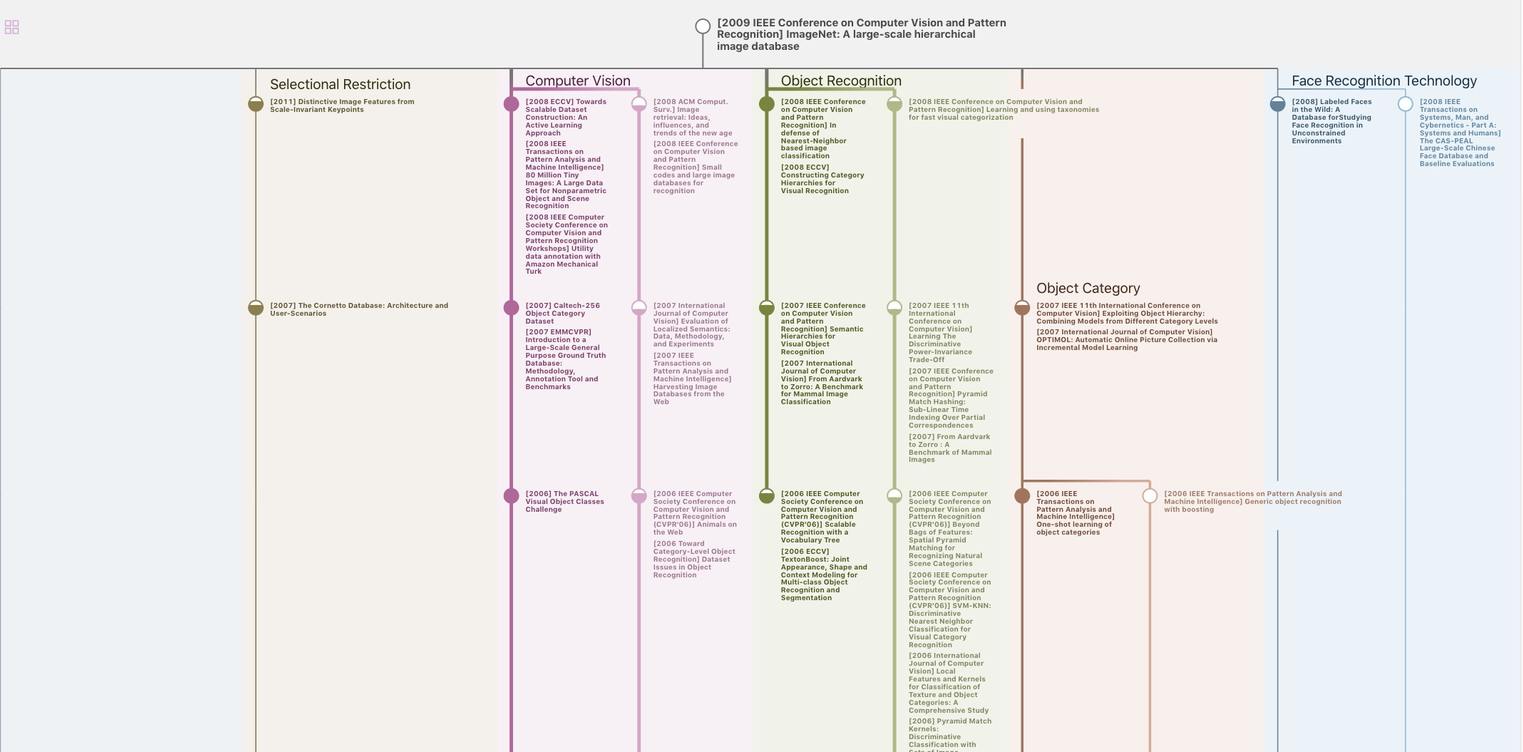
生成溯源树,研究论文发展脉络
Chat Paper
正在生成论文摘要