A Dual-channel Augmented Attentive Dense-convolutional Network for Power Image Splicing Tamper Detection
Neural computing & applications(2024)
摘要
Power image tampering brings certain security risks to the safe operation of power grids, among which splicing tampering is the most common. Although image tampering detection has received much attention in recent years, relatively little research has been conducted on the practical application to power systems, and the detection results are poor due to the difficulty of learning subtle edge features of tampered regions and the lack of power image tampering dataset for training. In order to effectively detect image tampering regions, this paper proposes a splicing tampering detection model with a Dual-channel Augmented Attentive Dense-convolutional Network (DAAD-Net) structure. The model consists of three main parts: backbone network feature extraction, augmented attention feature extraction, and tampered region detection. Firstly, the backbone network feature extraction module fuses the original tampered image features with the image residual features and transfers them into the backbone network to extract the feature map. Secondly, the augmented attention feature extraction module extracts the tampered region features in the higher and lower layers using hierarchical encoding and decoding operations. Finally, the extracted feature maps of each layer are sent to the tampered region detection module, and by combining the loss of each feature map for optimizing the network parameters. Additionally, we produced a power image tampering dataset containing 552 samples. Experiments demonstrate that the proposed method outperforms current state-of-the-art models, with 1% to 31% improvement in evaluation indicators, and has good robustness to noise and JPEG compression attacks.
更多查看译文
关键词
Power image,Splicing tampering detection,Augmented attention mechanism,Dense convolutional network
AI 理解论文
溯源树
样例
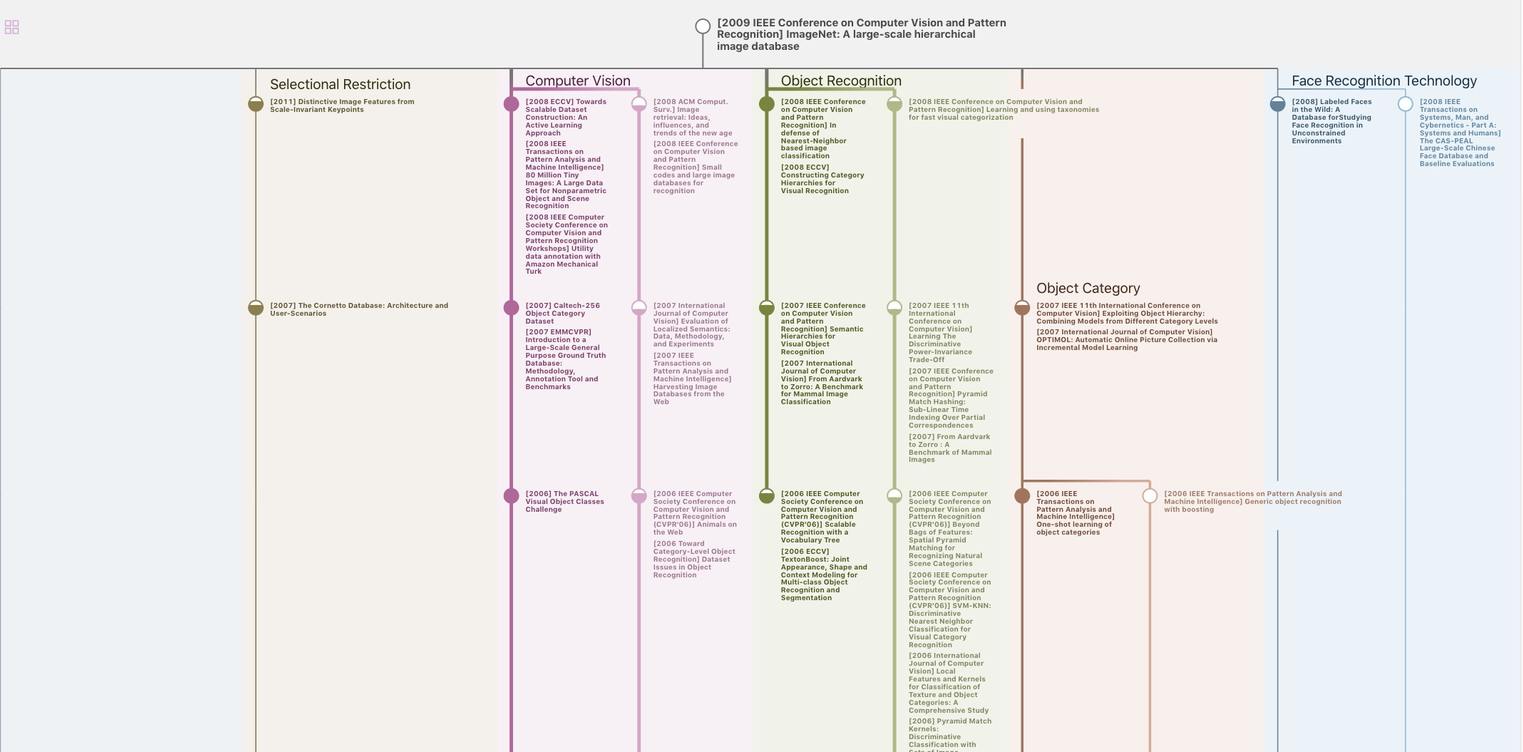
生成溯源树,研究论文发展脉络
Chat Paper
正在生成论文摘要