Location-Adaptive Generative Graph Augmentation for Fraud Detection.
2023 IEEE 5th International Conference on Cognitive Machine Intelligence (CogMI)(2023)
摘要
Class imbalance is a well-recognized challenge in GNN-based fraud detection. Traditional methods like re-sampling and re-weighting address this issue by balancing class distribution. However, node class balancing with simple re-sampling or re-weighting may significantly distort the data distributions and eventually lead to the ineffective performance of GNNs. In this paper, we propose a novel approach named Location-Adaptive Generative Node Augmentation (LAGA), which improves GNN-based fraud detection models by augmenting synthetic nodes of the minority class. To increase the variety for the synthetic nodes, LAGA utilizes the GAN framework to synthesize node features and related edges of fake fraudulent nodes. Specifically, LAGA include feature generation and edge generation modules. In order to make sure the generated features are consistent in terms of representing fraudulent nodes, we also design a location network that uses node features to find the neighborhood of synthetic nodes. Our empirical results on two real-world fraud datasets demonstrate that LAGA improves the performance of GNN-based fraud detection models by a large margin with much fewer nodes than traditional class balance methods, and outperforms recent graph augmentation methods with the same number of synthetic nodes.
更多查看译文
关键词
Graph Neural Networks,Fraud Detection,Node Augmentation,Generative Adversarial Network
AI 理解论文
溯源树
样例
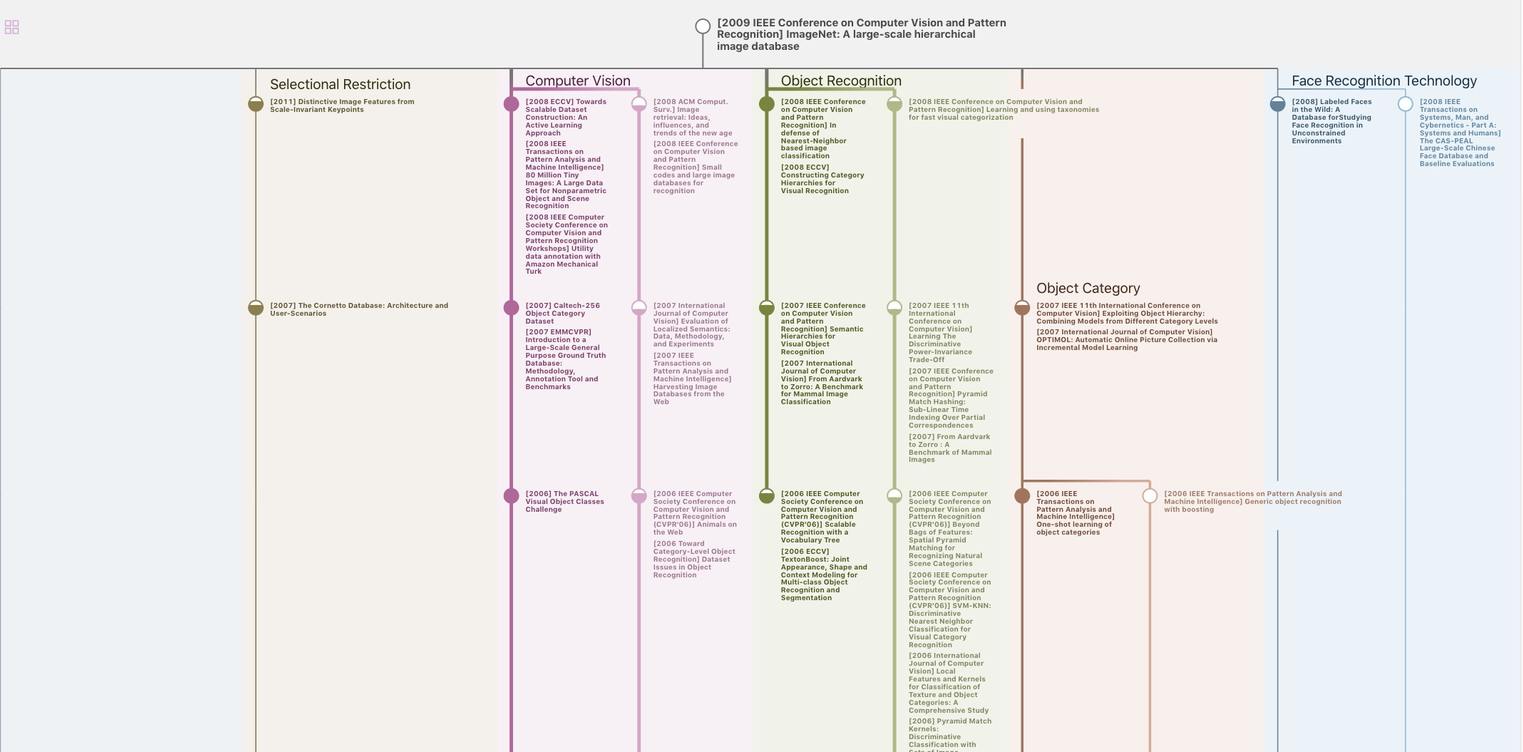
生成溯源树,研究论文发展脉络
Chat Paper
正在生成论文摘要