Metamorphic Testing for Traffic Sign Detection and Recognition.
International Conference on Software Quality, Reliability and Security(2023)
摘要
Traffic sign detection and recognition (TSDR) is a crucial technology to realize vehicle autonomous driving and maintain road safety. Misdetection or misclassification of traffic signs can lead to serious traffic accidents. Therefore, it is essential to ensure the correctness of traffic sign detection and recognition systems. However, most existing methods use labeled data, but it isn't easy to obtain labeled data. The metamorphic testing can reduce the dependence on the labeled data, but the current metamorphic relations do not consider the domain characteristics of traffic signs, so it is difficult to effectively detect traffic sign detection and recognition systems faults. To solve the above problems, this paper introduces metamorphic testing to verify traffic sign detection and recognition systems and designs and implements a tool, MTSDR, which provides a series of metamorphic relations in the traffic domain. It can generate many follow-up test images based on these metamorphic relations and automatically performs tests for evaluation, focusing on practical failure situations. The experimental evaluation was carried out on four advanced traffic sign detection and recognition models on open datasets. The results show that MTSDR can generate natural images and find thousands of traffic sign detection and recognition faults. Compared with the previous methods, our method has a higher fault detection capability, and the highest fault detection rate reaches more than 40%. In addition, it finds that retraining the traffic sign detection and recognition models using the images that found the fault can improve the model's performance, reduce its fault, and reduce the fault rate by 11.77% on average.
更多查看译文
关键词
metamorphic testing,traffic sign detection and recognition,metamorphic relation
AI 理解论文
溯源树
样例
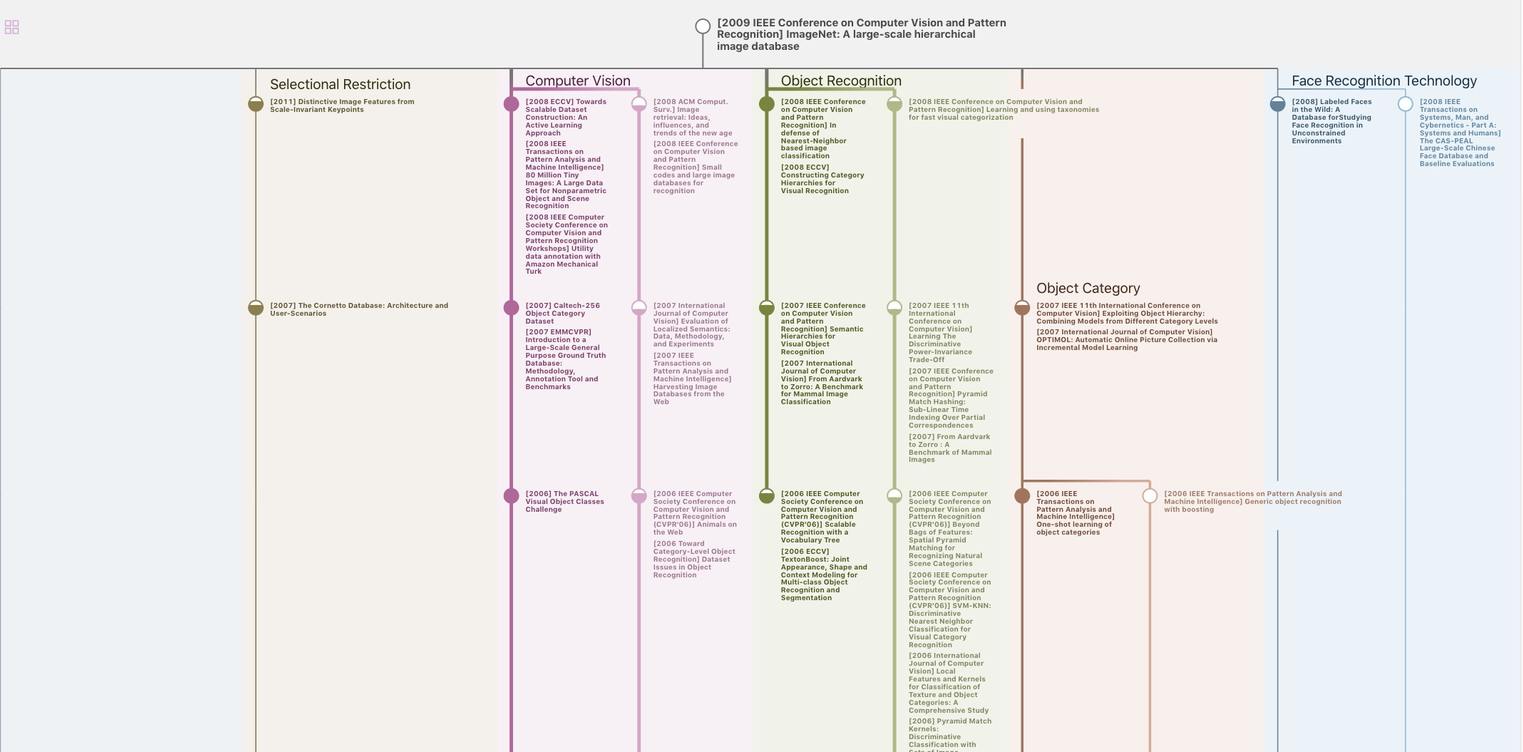
生成溯源树,研究论文发展脉络
Chat Paper
正在生成论文摘要