CiDAR-Former: Cosine-Weighting Deep Abundance Reconstruction Transformer for Fast Unsupervised Hyperspectral Anomaly Detection.
Workshop on Hyperspectral Image and Signal Processing(2023)
摘要
Hyperspectral anomaly detection (HAD) aims to classify those targets with distinctive spectral characteristics compared to the background. Although hyperspectral images (HSIs) have high spectral resolution for material identification, they are usually accompanied by over correlated and redundant information. Moreover, the mixed pixel phenomenon caused by the satellite hardware limitation weakens the distinguishability between abnormal pixels and their surrounding background pixels, making HAD a challenging inverse problem. This paper develops an unsupervised cosine-weighting deep abundance-reconstruction transformer (CiDAR-Former) to address the aforementioned issues. CiDAR-Former is the first work employing deep learning, together with a trickily designed cosine-similarity loss for suppressing anomaly effects, to unsupervisedly and effectively reconstruct clean abundances for the subsequent HAD. Real data experiments will demonstrate the superiority of the proposed CiDAR-Former both qualitatively and quantitatively.
更多查看译文
关键词
Hyperspectral anomaly detection (HAD),hyperspectral unmixing,inverse problem,self-attention,unsupervised transformer
AI 理解论文
溯源树
样例
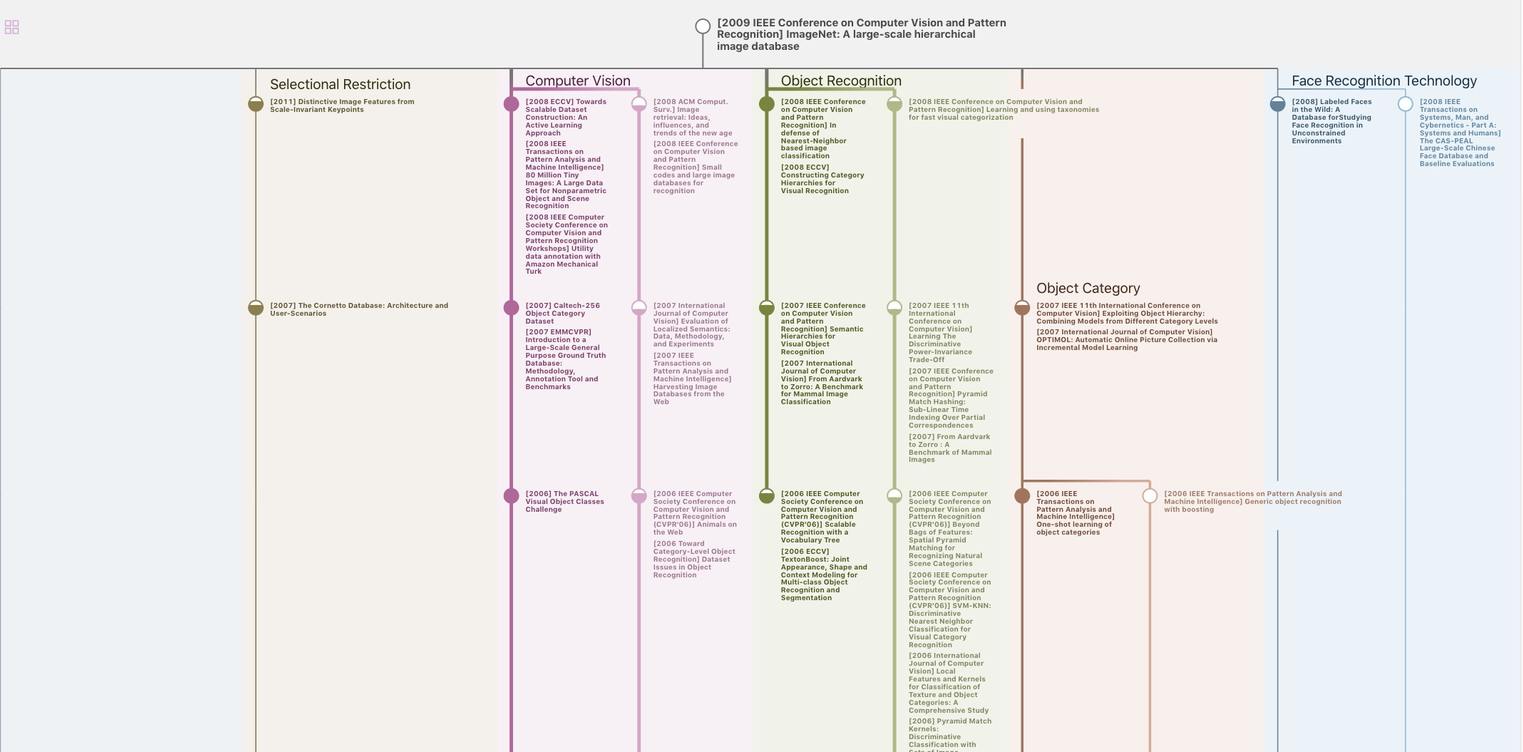
生成溯源树,研究论文发展脉络
Chat Paper
正在生成论文摘要