Bench Marking Tensor-based Imputation Techniques on Astronomical Imagery Data
2023 1st International Conference on Optimization Techniques for Learning (ICOTL)(2023)
摘要
The thriving industrial and societal application of raster data analytics has been hindered by missing pixel values caused primarily by sensor errors and data transmission issues. When confronted with this problem in real-world applications, researchers tried to fill in the missing values using various imputation techniques borrowed from statistics, expert systems, machine learning, and deep learning. Since each imputation technique has a selection bias that justifies the significance of one value over another, no universally acceptable best imputation technique exists to fill up the missing data for any given satellite imagery data. This paper aims to provide a benchmark and valuable insights into the performance, strengths, weaknesses, and suitability of popular imputation techniques for handling missing pixel imputation in satellite imagery data. Through a comprehensive analysis, our study will offer researchers and practitioners a deeper understanding of the effectiveness of these methods, enabling them to make informed decisions when addressing the challenge of missing pixel imputation in satellite imagery. The evaluation was performed using datasets obtained from the Kaguya and Chandrayan satellites, providing concrete insights into the performance of these techniques.
更多查看译文
关键词
Astronomical data,tensor completion,imputation,and raster images
AI 理解论文
溯源树
样例
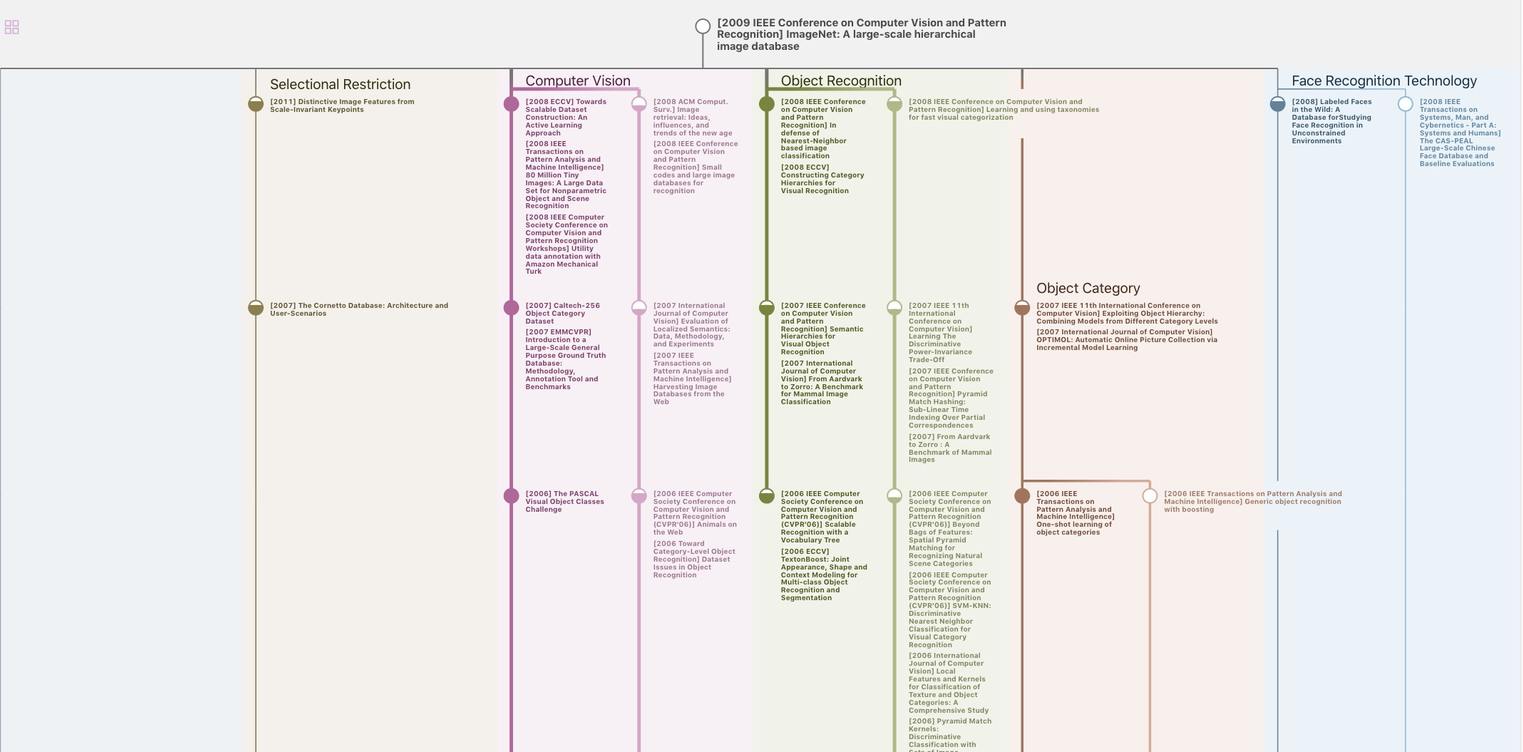
生成溯源树,研究论文发展脉络
Chat Paper
正在生成论文摘要