Mapping 30-m Resolution Bioclimatic Variables During 1991-2020 Climate Normals for Hubei Province, the Yangtze River Middle Reaches
IEEE JOURNAL OF SELECTED TOPICS IN APPLIED EARTH OBSERVATIONS AND REMOTE SENSING(2024)
摘要
High-resolution bioclimatic data are crucial to providing fine-scaled insights into biodiversity assessment, forestry, and agricultural management. Existing global bioclimatic datasets often exhibit kilometer-level coarse resolution or have miss the data in recent decades, potentially resulting in the issues of lower spatial accuracy, limited information, and restricted applicability in fine-scaled studies. Hubei Province in Yangtze River Middle Reaches has sparse meteorological stations in high-altitude mountainous areas to map the high-resolution bioclimatic variables directly. This study developed a 30-year averaged bioclimatic dataset for Hubei Province during 1991-2020 at a 30-m spatial resolution, utilizing monthly temperatures and precipitation derived from a downscaling-calibration framework. The downscaling of 1-km resolution climate variables was achieved by using a random forest model with 30-m resolution terrain and spatial covariates. Then, the geographical differential analysis was applied to improve the accuracy of downscaled products by including additional ground data. The mean absolute errors of calibrated monthly maximum, mean, minimum temperatures, and precipitation based on ordinary kriging decreased from 0.74(degrees)C, 0.47 C-degrees, 0.47 C-degrees, and 28.27 mm to 0.43 C-degrees, 0.28 C-degrees, 0.36 C-degrees, and 21.43 mm, respectively. Finally, calibrated climate variables were employed to calculate 19 annual bioclimatic variables, which were subsequently averaged over the 30-year period. The constructed bioclimatic dataset exhibits high overall consistency with the WorldClim dataset according to pixel-based comparison (Spearman correlation coefficients >0.6), with differences mainly attributed to the superior local accuracy of our dataset and climate changes. The dataset will provide fine-scaled, updated, and reliable data supports for local-related studies and decision making.
更多查看译文
关键词
Bioclimate,geographical differential analysis(GDA),Hubei Province,machine learning (ML),statisticaldownscaling
AI 理解论文
溯源树
样例
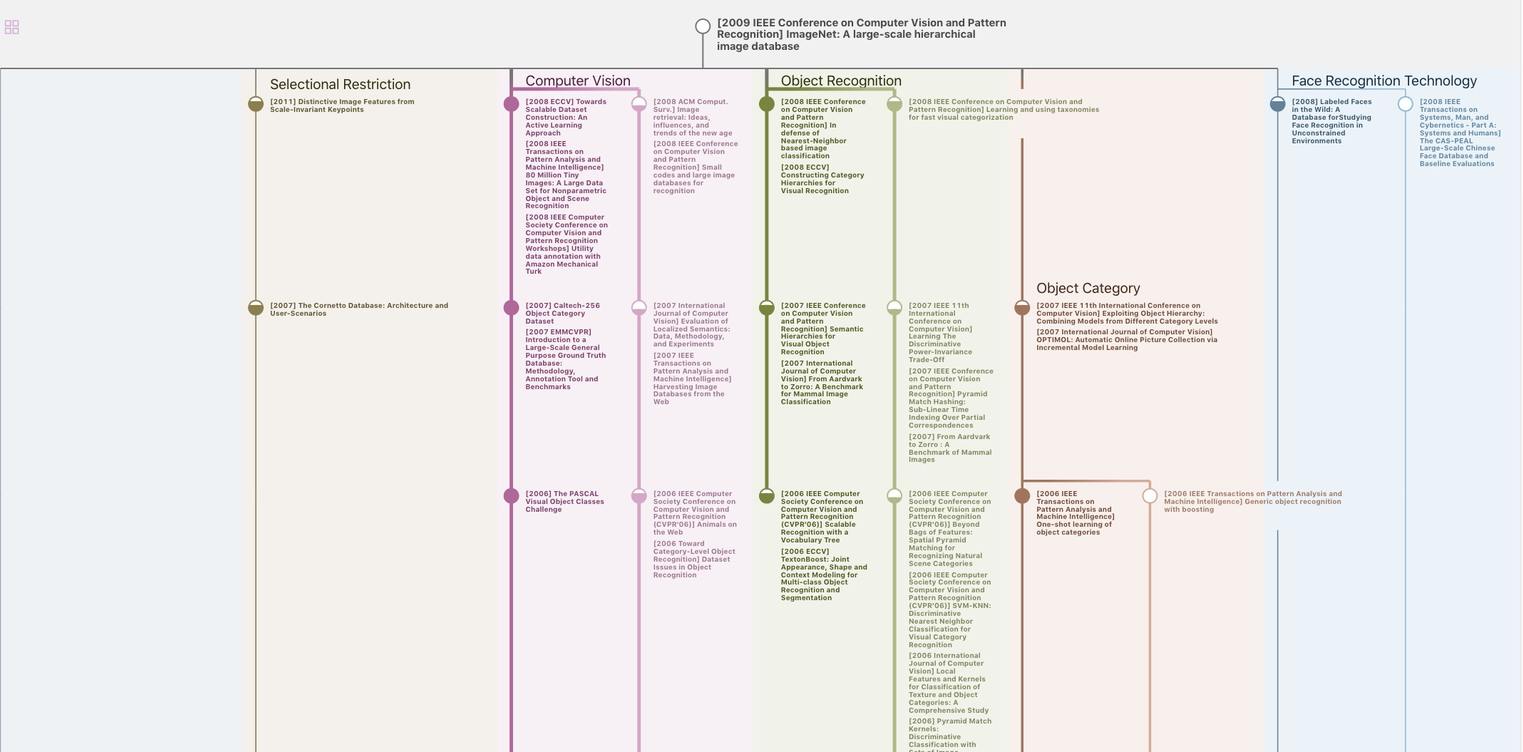
生成溯源树,研究论文发展脉络
Chat Paper
正在生成论文摘要