A novel unsupervised deep transfer learning method based on contrast pre-training for fault diagnosis
2023 IEEE 2ND INDUSTRIAL ELECTRONICS SOCIETY ANNUAL ON-LINE CONFERENCE, ONCON(2023)
摘要
Unsupervised transfer learning has become a research hotspot in the field of mechanical equipment fault diagnosis. It typically assumes a large amount of labeled data is available for constructing a diagnosis model in the source domain, and aims at applying the model in the target domain by adjusting marginal and conditional data distributions using unlabeled data of the target domain. However, the labeling capability is often extremely limited for large data, and automatically balancing the confrontation of adjusting both marginal and conditional distribution is difficult. To this end, this paper proposes a deep transfer adversarial learning method based on contrast pretraining (DTALC). A fault diagnosis model can be contrasted under the few-label constraint in source domain, where a feature extractor is pre-trained unsupervised by contrast coding, based on which a satisfactory classifier can be built using a few labeled data. And an adversarial factor is designed to automatically adapt the weights between the global discriminator and the local discriminator to release the marginal and conditional distribution adaptation problem of unsupervised transfer learning. The proposed DTALC is verified in On publicly available datasets and its diagnosis accuracy outperforms other state-of-the-art methods considering multiple combinations of source and target domains.
更多查看译文
关键词
Fault diagnosis,contrast coding,unsupervised transfer learning
AI 理解论文
溯源树
样例
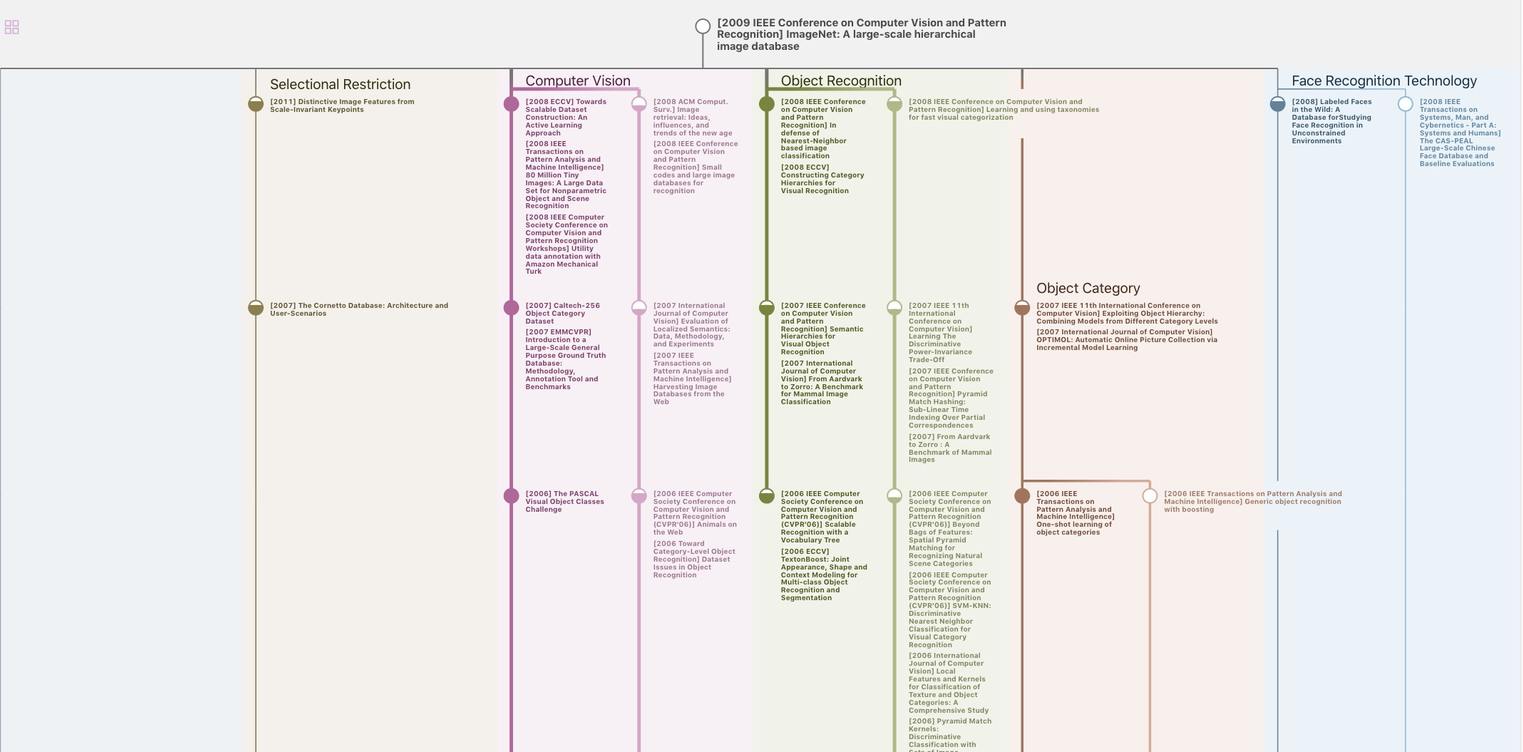
生成溯源树,研究论文发展脉络
Chat Paper
正在生成论文摘要