Prediction of Secondary Structure for Long Non-Coding RNAs using a Recursive Cutting Method based on Deep Learning.
IEEE International Conference on Bioinformatics and Bioengineering(2023)
摘要
Accurately predicting the secondary structure of RNA, particularly for long non-coding RNA, has direct implications in healthcare, where it can be used for diagnostic, therapeutic, and drug discovery purposes. However, the majority of previous approaches are too costly in terms of computation budget to cope with the increasing complexity of long RNAs, and the ones that can scale to long RNAs lack accuracy to reliably predict their structures. We propose a new approach combining recursive cutting and machine learning techniques for predicting the secondary structures of long non-coding RNAs. In comparison, our method proves to be computationally efficient by recursively partitioning a sequence into smaller fragments until they can be easily managed by an existing model. We perform a benchmark of different state-of-the-art models and show that our approach indeed demonstrates better performance for long RNAs and a potential to bring significant improvements in the future, as well as interesting enhancing properties, which we discuss.
更多查看译文
关键词
long non-coding RNA,secondary structure prediction,deep learning
AI 理解论文
溯源树
样例
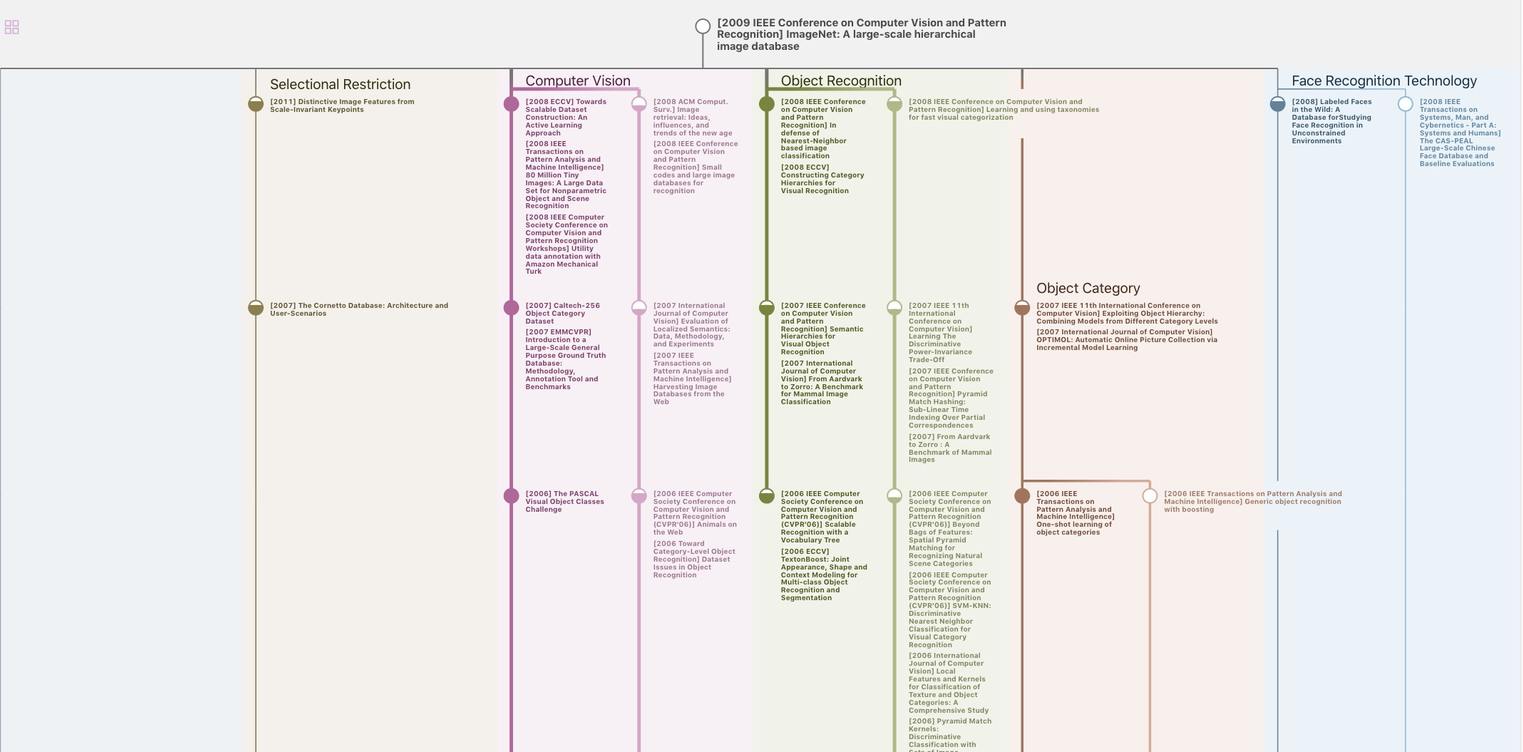
生成溯源树,研究论文发展脉络
Chat Paper
正在生成论文摘要