Target Tracking in 4D US Based on Template Matching and Target Forecasting Using Spatio-Temporal Autoencoders.
IEEE International Conference on Bioinformatics and Bioengineering(2023)
摘要
4D ultrasound (US) imaging is a promising imaging modality for diagnosis as well as therapy guidance. It provides volumetric images of soft-tissue structures in real-time without harming the patient with ionizing radiation. Soft tissue targets in the liver are affected by breathing-induced movements resulting in high dimensional motion patterns including deformations affecting the target appearance. Thus, using 4D US for target tracking in radiation therapy to enhance the treatment accuracy is promising but challenging. In this study, a novel approach for target tracking in 4D US is proposed based on predicting the appearance of the target for the next US frame. A spatio-temporal autoencoder as well as a recurrent neural network are implemented and trained based on a labeled 4D US data set with a mean spatial resolution of
$1.0\times 0.7\times 1.5$
mm
3
for predicting volumetric target patches and target locations. Four template matching-based tracking algorithms are implemented and evaluated in terms of tracking accuracy. The results indicate that using predicted targets for template matching reduce the tracking error by 62 %, on average. By using a naive template matching algorithm, a mean tracking error of
$7.55\pm 12.31$
mm was measured. In contrast, using predicted targets, a mean tracking error of
$2.85\pm 2.04$
mm was determined. Since the proposed spatio-temporal autoencoder is independent of the tracking algorithm, it can be combined with any tracking algorithm, making it a promising approach for improving target tracking accuracy.
更多查看译文
关键词
deep representation learning,radiation therapy,ultrasound guidance
AI 理解论文
溯源树
样例
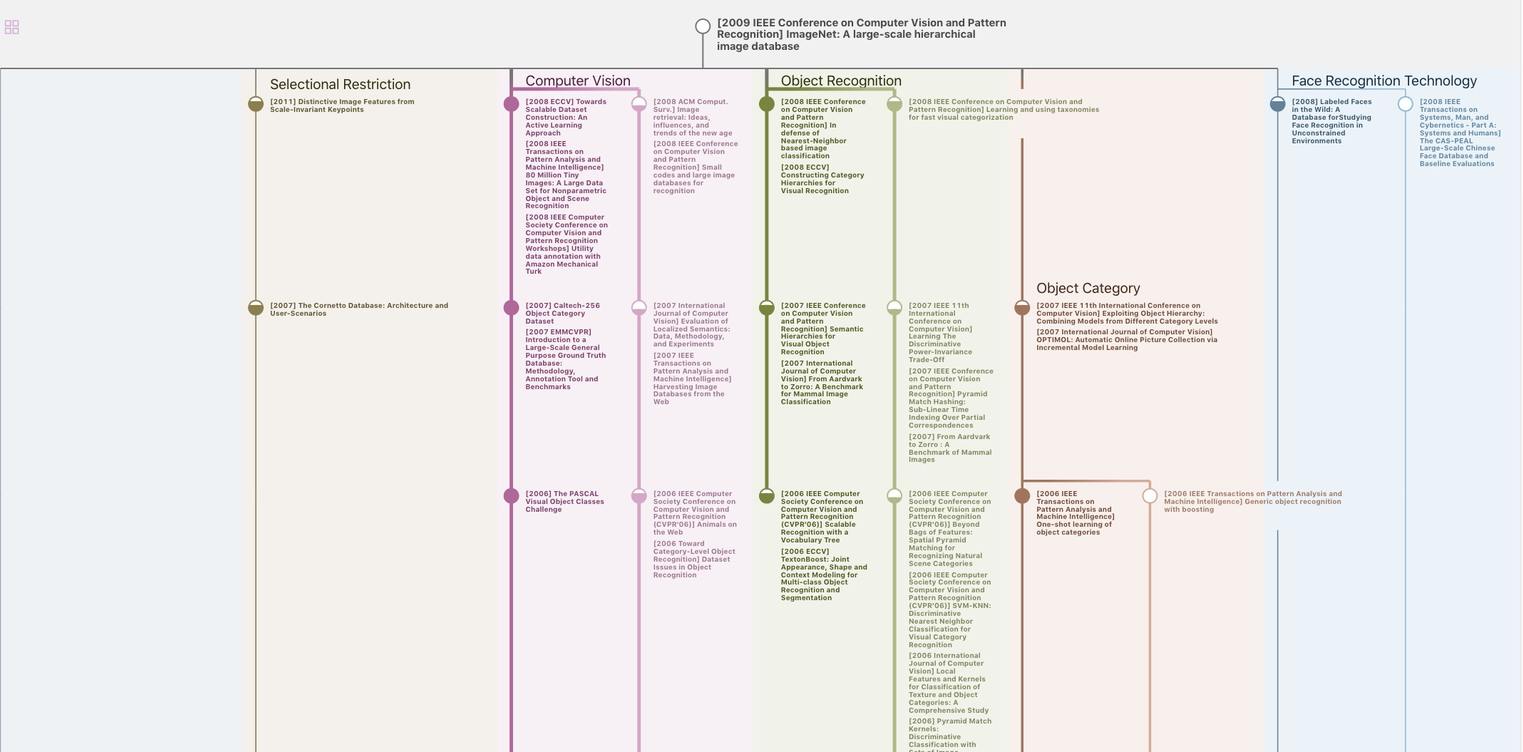
生成溯源树,研究论文发展脉络
Chat Paper
正在生成论文摘要