YOLO-Ant: A Lightweight Detector Via Depthwise Separable Convolutional and Large Kernel Design for Antenna Interference Source Detection
IEEE TRANSACTIONS ON INSTRUMENTATION AND MEASUREMENT(2024)
摘要
In the era of 5G communication, removing interference sources that affectcommunication is a resource-intensive task. The rapid development of computervision has enabled unmanned aerial vehicles to perform various high-altitudedetection tasks. Because the field of object detection for antenna interferencesources has not been fully explored, this industry lacks dedicated learningsamples and detection models for this specific task. In this article, anantenna dataset is created to address important antenna interference sourcedetection issues and serves as the basis for subsequent research. We introduceYOLO-Ant, a lightweight CNN and transformer hybrid detector specificallydesigned for antenna interference source detection. Specifically, we initiallyformulated a lightweight design for the network depth and width, ensuring thatsubsequent investigations were conducted within a lightweight framework. Then,we propose a DSLK-Block module based on depthwise separable convolution andlarge convolution kernels to enhance the network's feature extraction ability,effectively improving small object detection. To address challenges such ascomplex backgrounds and large interclass differences in antenna detection, weconstruct DSLKVit-Block, a powerful feature extraction module that combinesDSLK-Block and transformer structures. Considering both its lightweight designand accuracy, our method not only achieves optimal performance on the antennadataset but also yields competitive results on public datasets.
更多查看译文
关键词
YOLO-Ant,Antenna interference source detection,Small object detection,Lightweight,CNN-transformer fusion
AI 理解论文
溯源树
样例
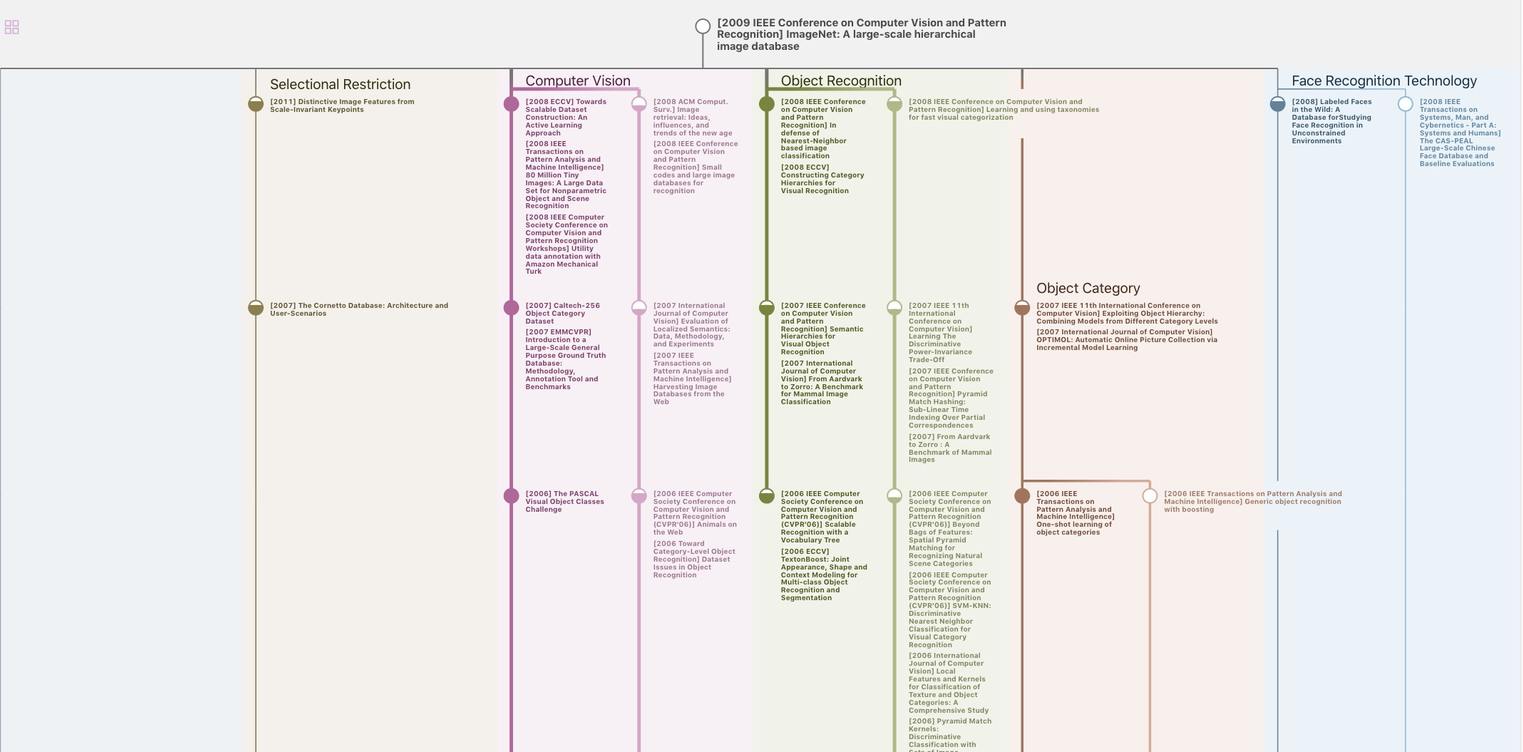
生成溯源树,研究论文发展脉络
Chat Paper
正在生成论文摘要