The Impact of Demonstrations on Multilingual In-Context Learning: A Multidimensional Analysis
CoRR(2024)
摘要
In-context learning is a popular inference strategy where large language
models solve a task using only a few labelled demonstrations without needing
any parameter updates. Compared to work on monolingual (English) in-context
learning, multilingual in-context learning is under-explored, and we lack an
in-depth understanding of the role of demonstrations in this context. To
address this gap, we conduct a multidimensional analysis of multilingual
in-context learning, experimenting with 5 models from different model families,
9 datasets covering classification and generation tasks, and 56 typologically
diverse languages. Our results reveal that the effectiveness of demonstrations
varies significantly across models, tasks, and languages. We also find that
Llama 2-Chat, GPT-3.5, and GPT-4 are largely insensitive to the quality of
demonstrations. Instead, a carefully crafted template often eliminates the
benefits of demonstrations for some tasks and languages altogether. These
findings show that the importance of demonstrations might be overestimated. Our
work highlights the need for granular evaluation across multiple axes towards a
better understanding of in-context learning.
更多查看译文
AI 理解论文
溯源树
样例
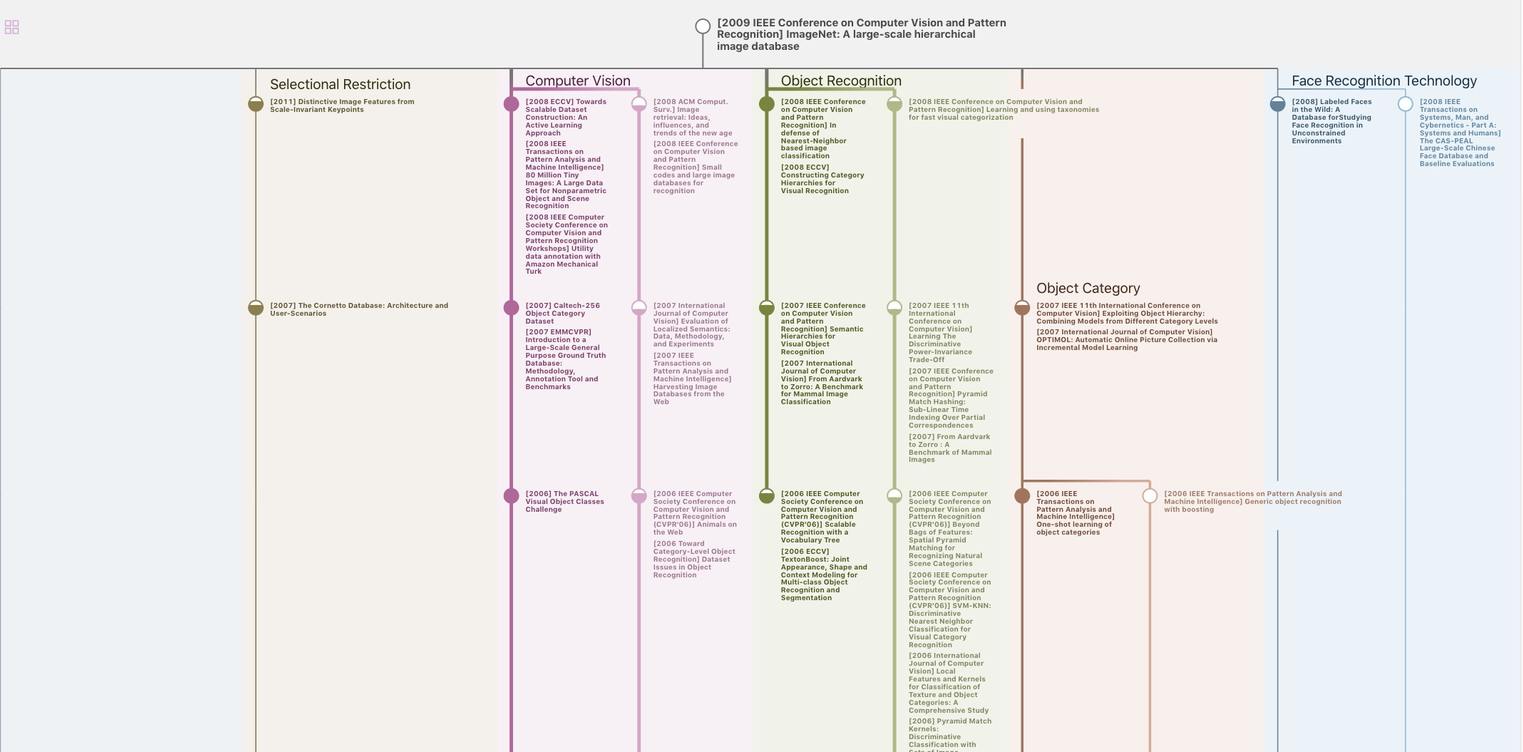
生成溯源树,研究论文发展脉络
Chat Paper
正在生成论文摘要