Heterogeneous Graph Reasoning for Fact Checking over Texts and Tables
Proceedings of the AAAI Conference on Artificial Intelligence(2024)
摘要
Fact checking aims to predict claim veracity by reasoning over multipleevidence pieces. It usually involves evidence retrieval and veracity reasoning.In this paper, we focus on the latter, reasoning over unstructured text andstructured table information. Previous works have primarily relied onfine-tuning pretrained language models or training homogeneous-graph-basedmodels. Despite their effectiveness, we argue that they fail to explore therich semantic information underlying the evidence with different structures. Toaddress this, we propose a novel word-level Heterogeneous-graph-based model forFact Checking over unstructured and structured information, namely HeterFC. Ourapproach leverages a heterogeneous evidence graph, with words as nodes andthoughtfully designed edges representing different evidence properties. Weperform information propagation via a relational graph neural network,facilitating interactions between claims and evidence. An attention-basedmethod is utilized to integrate information, combined with a language model forgenerating predictions. We introduce a multitask loss function to account forpotential inaccuracies in evidence retrieval. Comprehensive experiments on thelarge fact checking dataset FEVEROUS demonstrate the effectiveness of HeterFC.Code will be released at: https://github.com/Deno-V/HeterFC.
更多查看译文
AI 理解论文
溯源树
样例
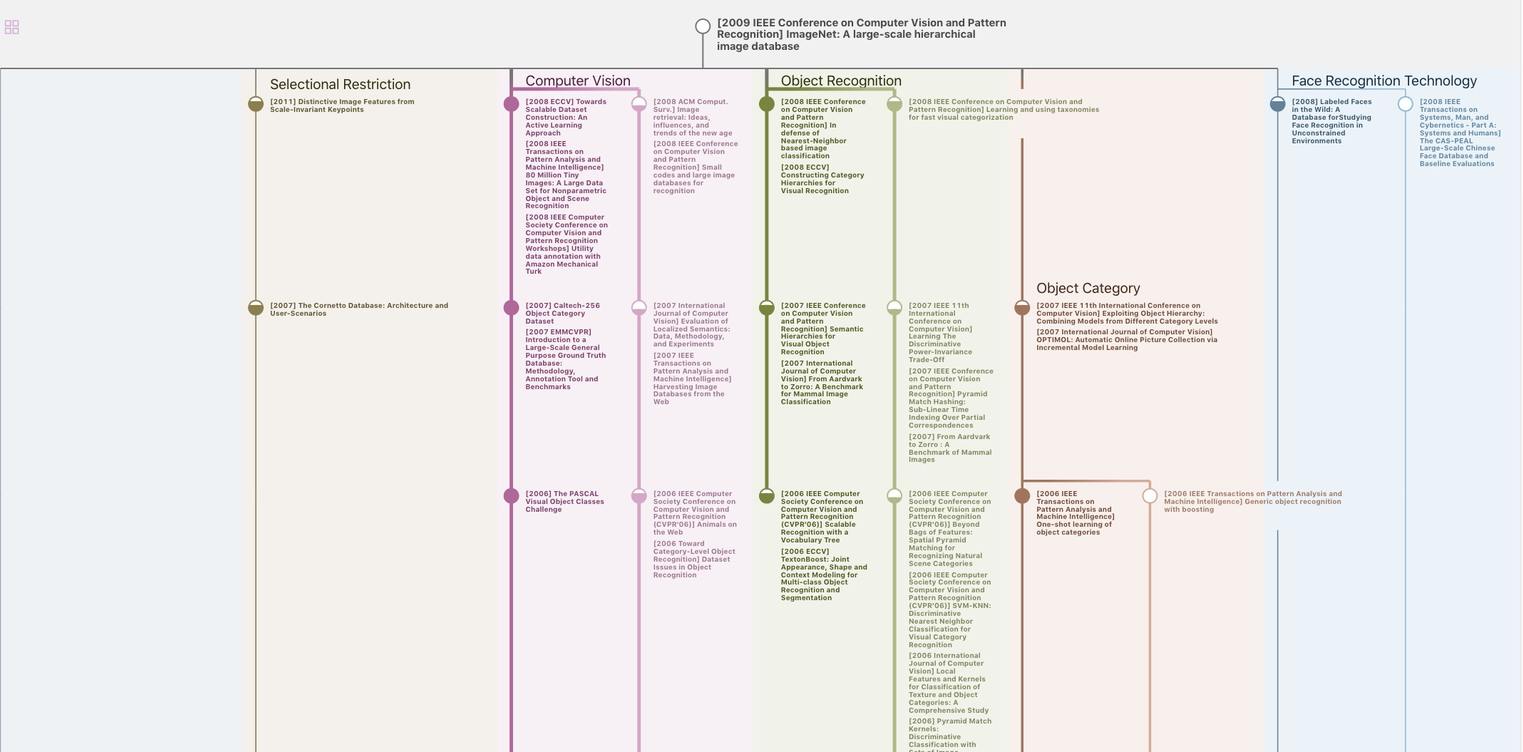
生成溯源树,研究论文发展脉络
Chat Paper
正在生成论文摘要