Detecting fake information with knowledge-enhanced AutoPrompt
Neural Computing and Applications(2024)
摘要
The rapid growth of fake news on the Internet poses a challenge to accessing authentic information. Fake news detection plays a crucial role in filtering out false information and improving information accuracy. However, practical implementation faces challenges like high annotation costs, limited samples, poor training results, and weak model generalization. To tackle these issues, we propose knowledge-enhanced AutoPrompt (KEAP) for fake news detection. This method leverages prompt templates generated by the T5 model to transform the fake news detection task into a prompt learning-based task. We also incorporate external entity knowledge to enhance detection capabilities. Carefully designed prompts activate the model’s latent knowledge, improving performance in low-resource scenarios and model generalization. Experiments on GossipCop and PolitiFact datasets demonstrate the superiority of prompt learning over existing methods without extra text during testing. KEAP achieves an average F1 score improvement of 2.79 % compared to state-of-the-art methods in few-shot settings.
更多查看译文
关键词
Fake news detection,Few-shot,Knowledge,Prompt
AI 理解论文
溯源树
样例
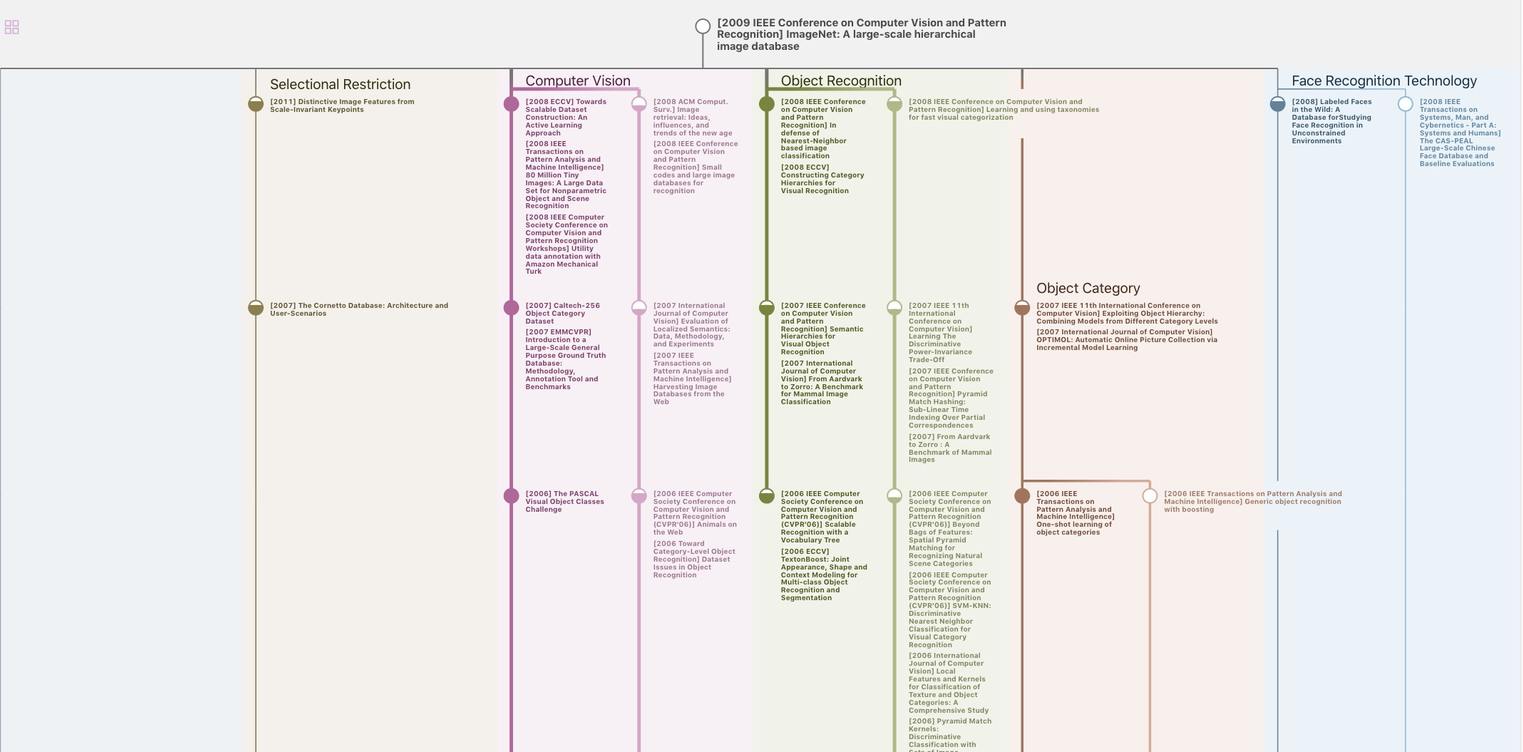
生成溯源树,研究论文发展脉络
Chat Paper
正在生成论文摘要