Facial micro-expression recognition using three-stream vision transformer network with sparse sampling and relabeling
Signal, Image and Video Processing(2024)
摘要
Most existing micro-expression recognition (MER) methods are based on convolutional neural networks (CNN) and could obtain better representations than conventional handcrafted-based methods. Nevertheless, the local receptive field of CNN leads to poor global feature extraction and thus limits the accuracy. In contrast, the vision transformer, an alternative technique, could capture global facial information and perform superiority over CNN in many vision tasks. However, directly applying it to MER may not be as effective as expected since the insufficient data and class-imbalanced characteristics of existing ME datasets could seriously restrict the accuracy. To address these problems, we propose a three-stream vision transformer-based network with sparse sampling and relabeling (SSRLTS-ViT). First, the network learns discriminative ME representations from three optical flow components. Second, a sparse sampling strategy is employed to add the optical flow components computed by the onset and images around the apex into training sets, which can expand the sample capacity and simultaneously guarantee the differences between data. Moreover, we introduce a relabeling mechanism to reassign the training data with correct labels to decrease the impact caused by subjectivity annotations, which can further improve recognition accuracy. Experimental results on two benchmarks show that SSRLTS-ViT outperforms other competing methods by obtaining the UF1 of 0.843 and UAR of 0.853 on the 3-class datasets and the UF1 of 0.795 and UAR of 0.801 on the 5-class datasets, respectively.
更多查看译文
关键词
Micro-expression recognition,Vision transformer,Multi-stream,Sparse sampling,Relabeling
AI 理解论文
溯源树
样例
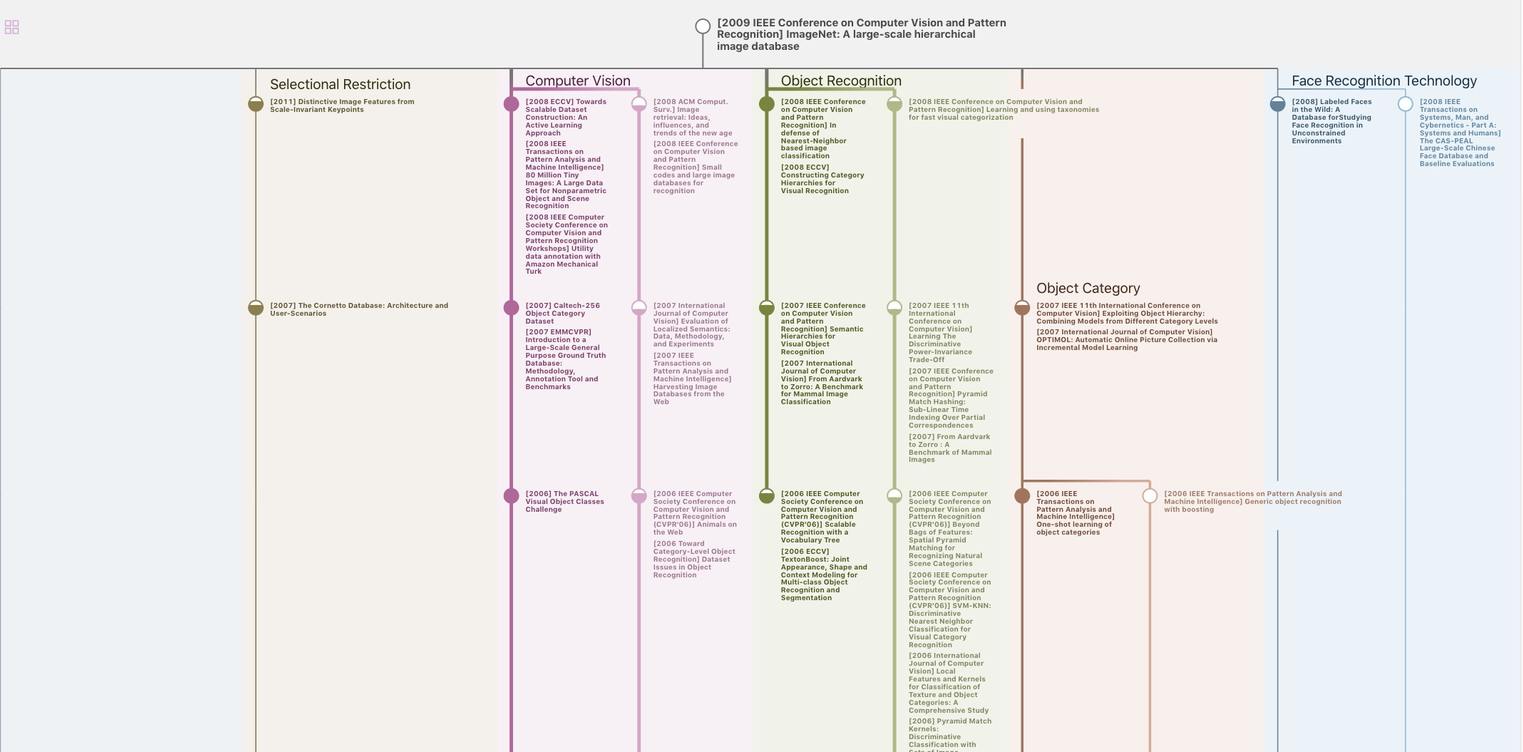
生成溯源树,研究论文发展脉络
Chat Paper
正在生成论文摘要