A Bayesian sampling optimisation strategy for finite element model updating
Journal of Civil Structural Health Monitoring(2024)
摘要
Model Updating (MU) aims to estimate the unknown properties of a physical system of interest from experimental observations. In Finite Element (FE) models, these unknowns are the elements’ parameters. Typically, besides model calibration purposes, MU and FEMU procedures are employed for the Non-Destructive Evaluation (NDE) and damage assessment of structures. In this framework, damage can be located and quantified by updating the parameters related to stiffness. However, these procedures require the minimisation of a cost function, defined according to the difference between the model and the experimental data. Sophisticated FE models can generate expensive and non-convex cost functions, which minimization is a non-trivial task. To deal with this challenging optimization problem, this work makes use of a Bayesian sampling optimisation technique. This approach consists of generating a statistical surrogate model of the underlying cost function (in this case, a Gaussian Process is used) and applying an acquisition function that drives the intelligent selection of the next sampling point, considering both exploitation and exploration needs. This results in a very efficient yet very powerful optimization technique, necessitating of minimal sampling volume. The performance of this proposed scheme is then compared to three well-established global optimisation algorithms. This investigation is performed on numerical and experimental case studies based on the famous Mirandola bell tower.
更多查看译文
关键词
Bayesian optimisation,Bayesian expected improvement,Finite Element Model Updating,Bell Tower,Masonry structures
AI 理解论文
溯源树
样例
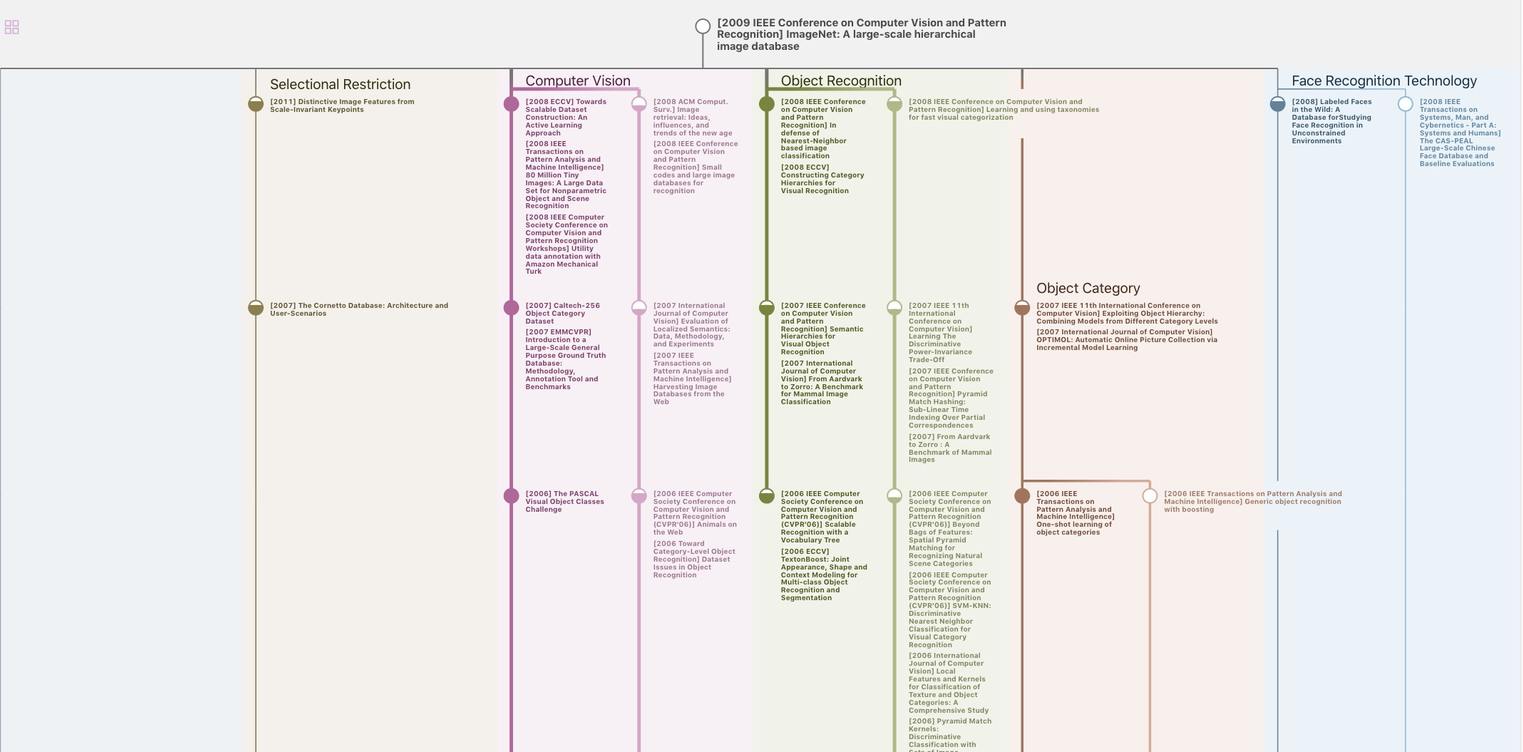
生成溯源树,研究论文发展脉络
Chat Paper
正在生成论文摘要