Explainable Classification Techniques for Quantum Dot Device Measurements
CoRR(2024)
摘要
In the physical sciences, there is an increased need for robust feature
representations of image data: image acquisition, in the generalized sense of
two-dimensional data, is now widespread across a large number of fields,
including quantum information science, which we consider here. While
traditional image features are widely utilized in such cases, their use is
rapidly being supplanted by Neural Network-based techniques that often
sacrifice explainability in exchange for high accuracy. To ameliorate this
trade-off, we propose a synthetic data-based technique that results in
explainable features. We show, using Explainable Boosting Machines (EBMs), that
this method offers superior explainability without sacrificing accuracy.
Specifically, we show that there is a meaningful benefit to this technique in
the context of quantum dot tuning, where human intervention is necessary at the
current stage of development.
更多查看译文
AI 理解论文
溯源树
样例
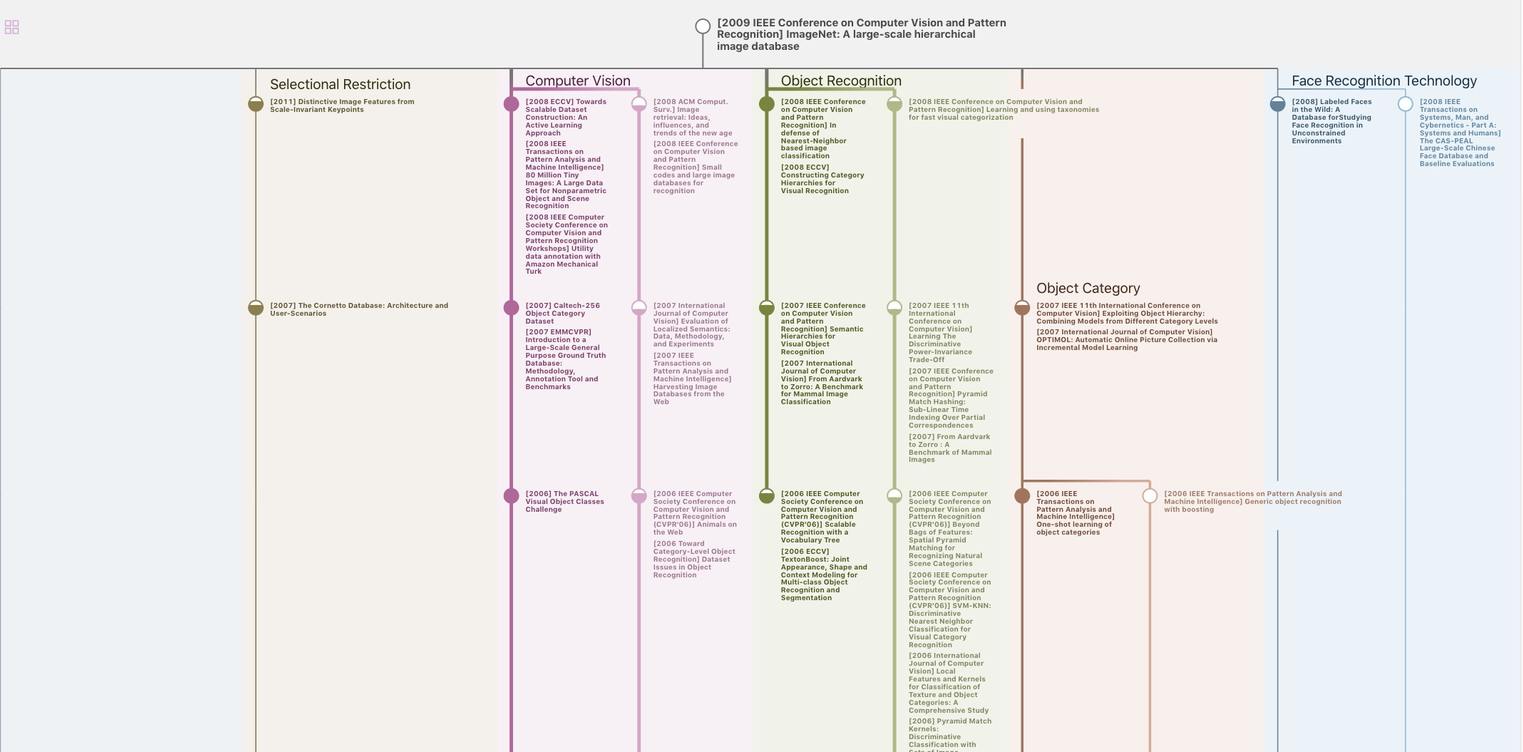
生成溯源树,研究论文发展脉络
Chat Paper
正在生成论文摘要