Grounding from an AI and Cognitive Science Lens
IEEE Intelligent Systems(2024)
关键词
Grounding,Collaboration,Machine learning,Cognitive science,Task analysis,Intelligent systems,Artificial intelligence,Neural engineering,Symbols
AI 理解论文
溯源树
样例
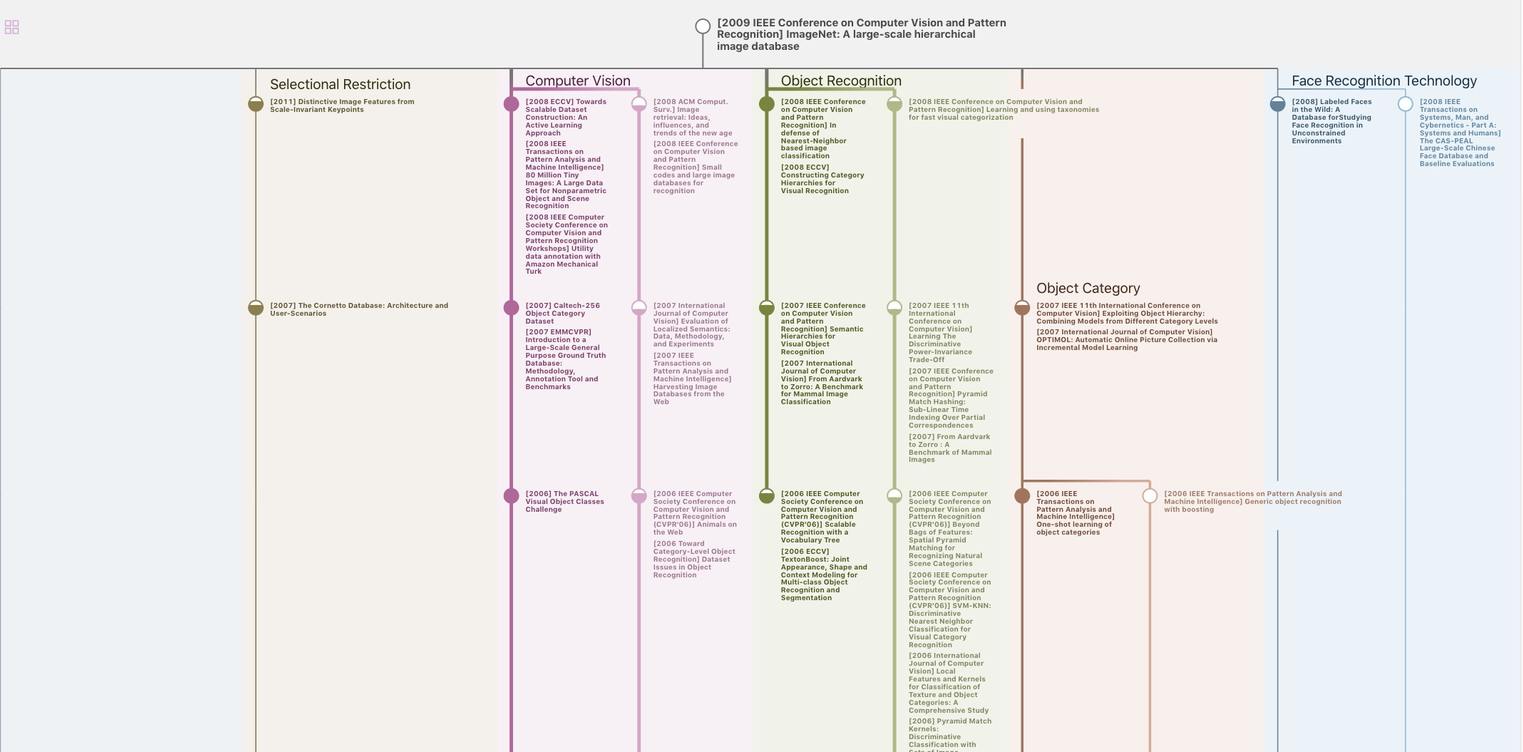
生成溯源树,研究论文发展脉络
Chat Paper
正在生成论文摘要