CGF: A Category Guidance Based PM$_{2.5}$ Sequence Forecasting Training Framework
IEEE Transactions on Knowledge and Data Engineering(2023)
Abstract
PM
$_{2.5}$
concentration forecasting is important yet challenging. First, complicated local fluctuations in PM
$_{2.5}$
concentrations disturb modeling global trends. Second, forecasting errors are often accumulated through an autoregressive process. To contend with the two challenges, we propose a
C
ategory
G
uidance based PM
${_{2.5}}$
sequence
F
orecasting training framework (CGF) to enhance the performance of existing PM
${_{2.5}}$
concentration forecasting models. CGF contains a Category based Representation Learning (CRL) module and a Category based Self-paced Learning (CSL) module, both of which utilize PM
${_{2.5}}$
category information that is easily obtained and publicly available. First, CRL employs category information to guide forecasting models to produce more robust hidden representations that are insensitive to local fluctuations, thus alleviating the negative impact of local fluctuations. Second, CSL adaptively selects real PM
${_{2.5}}$
concentration values versus autoregressive PM
${_{2.5}}$
forecast values when training forecasting models, helping alleviate error accumulations. The CGF framework is applied to existing PM
${_{2.5}}$
forecasting models, and the experimental results on two real-world datasets demonstrate that CGF is able to consistently improve the accuracy of existing forecasting models. Furthermore, to validate the generality of CGF, we conduct extensional experiments in two other time-series prediction tasks, including exchange rate forecasting and electricity forecasting. The experimental results also verify the effectiveness of CGF.
MoreTranslated text
Key words
Category guidance,PM $_{2.5}$ 2 . 5 concentration forecasting,spatio-temporal,temporal
AI Read Science
Must-Reading Tree
Example
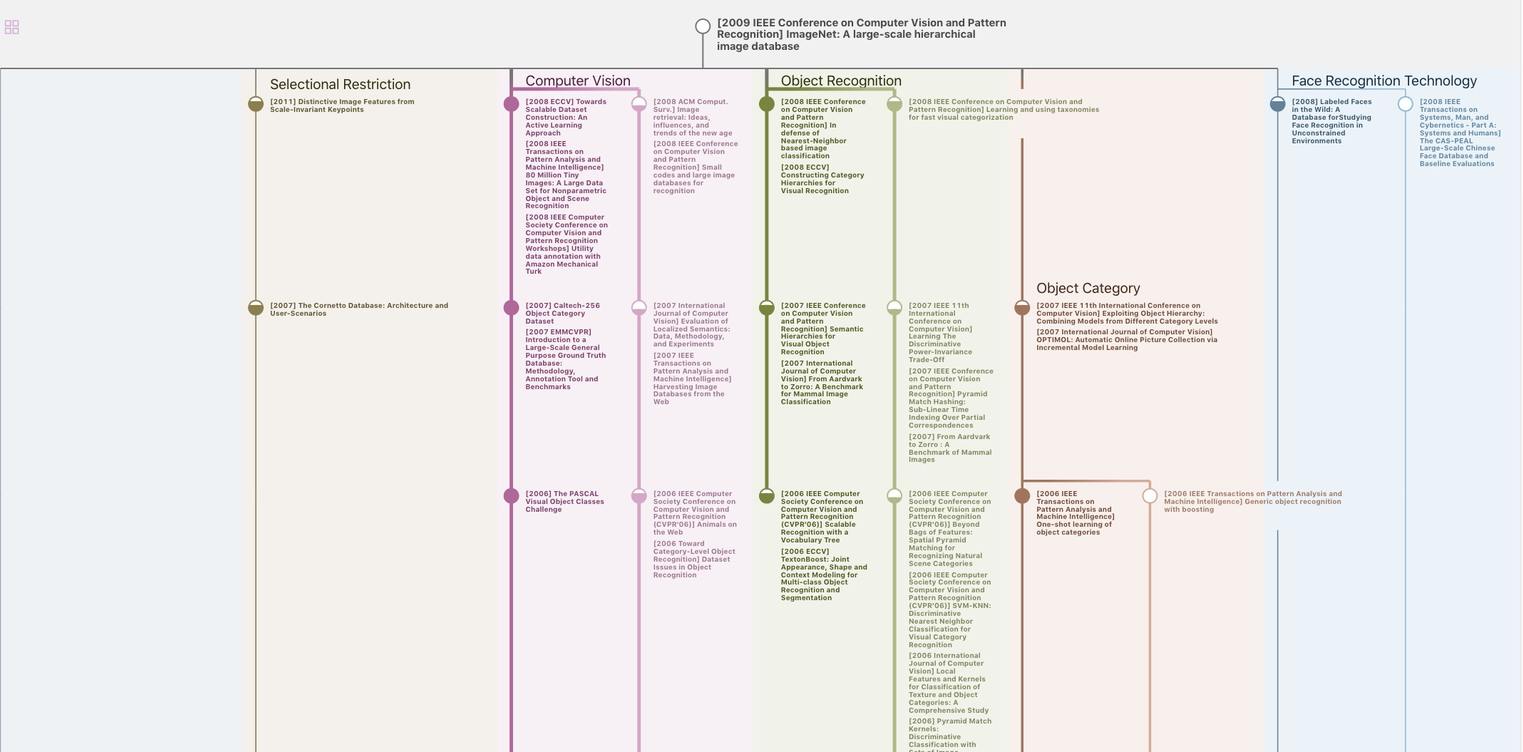
Generate MRT to find the research sequence of this paper
Chat Paper
Summary is being generated by the instructions you defined