PQA: Zero-shot Protein Question Answering for Free-form Scientific Enquiry with Large Language Models
CoRR(2024)
摘要
We introduce the novel task of zero-shot Protein Question Answering (PQA) for
free-form scientific enquiry. Given a previously unseen protein sequence and a
natural language question, the task is to deliver a scientifically accurate
answer. This task not only supports future biological research, but could also
provide a test bed for assessing the scientific precision of large language
models (LLMs). We contribute the first specialized dataset for PQA model
training, containing 257K protein sequences annotated with 1.97M scientific
question-answer pairs. Additionally, we propose and study several novel
biologically relevant benchmarks for scientific PQA. Employing two robust
multi-modal architectures, we establish an initial state-of-the-art performance
for PQA and reveal key performance factors through ablation studies. Our
comprehensive PQA framework, named Pika, including dataset, code, model
checkpoints, and a user-friendly demo, is openly accessible on
github.com/EMCarrami/Pika, promoting wider research and application in the
field.
更多查看译文
AI 理解论文
溯源树
样例
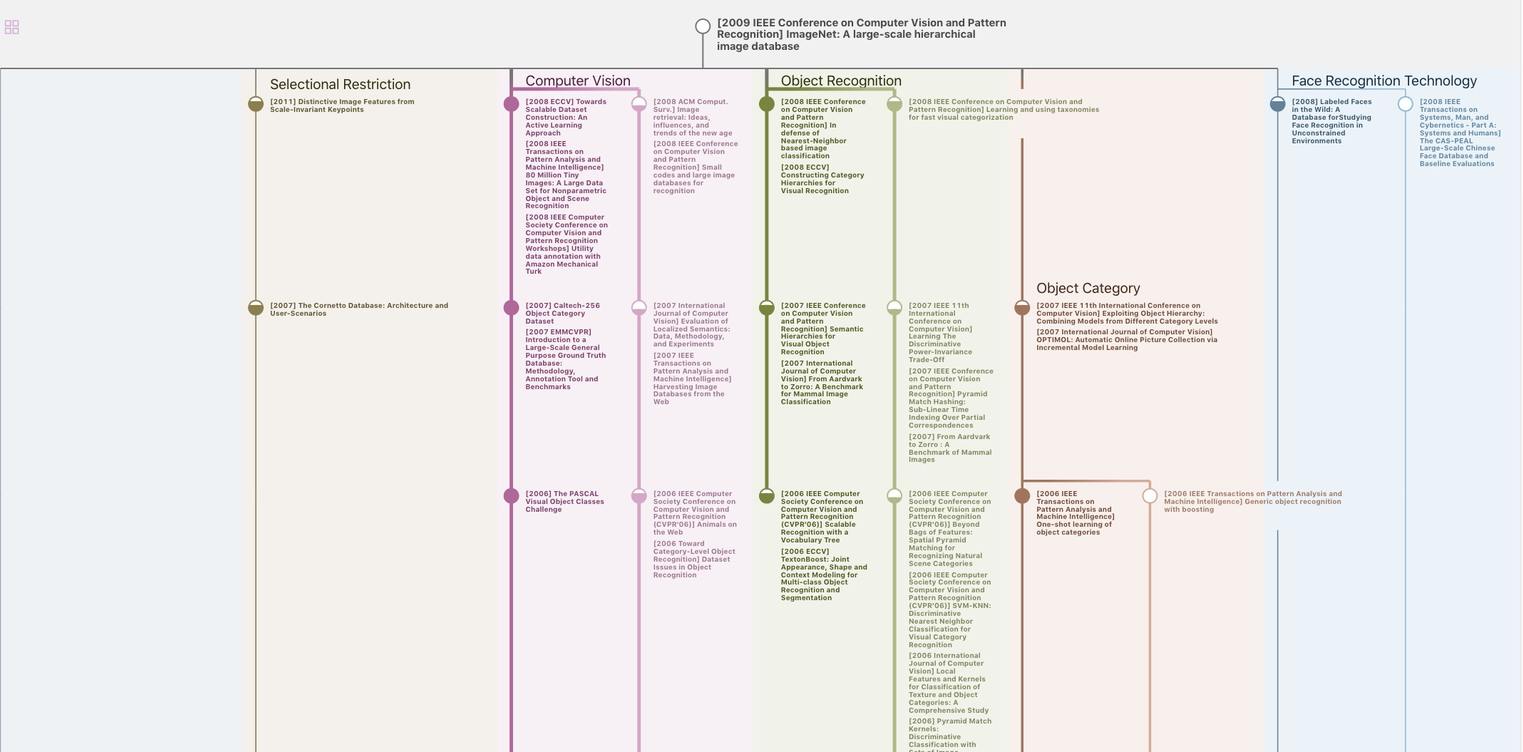
生成溯源树,研究论文发展脉络
Chat Paper
正在生成论文摘要