Predictive models of electric vehicle adoption in the United States: Charging ahead with renewable energy
TRANSPORTATION RESEARCH INTERDISCIPLINARY PERSPECTIVES(2024)
摘要
In this paper, we develop several predictive models pertaining to Electric Vehicles in the United States. We set out to understand three phenomena: Public Charging Units, Electric Vehicle Jobs, and Electric Vehicle Registrations. To model them, we include variables from various categories - demographics, economics, education, environment, finance, geographics, public health, and technographics - in the timespan of 2010-2020. We integrate data from multiple data sources and use them to understand, explain, and predict the Electric Vehicle phenomena combining state and county level data. We obtain three random effects linear regression models having variance explained of 51.5-62.4%. We also fit several machine learning models to improve the accuracy of the models, highlighting nonlinearities, to provide additional insights. The overall predictive accuracy of each Gradient Boosted Tree model is far superior to that of each linear regression model and significantly better than the other machine learning models. Our core contribution is that, spanning all three phenomena, the three most important predictor variables are solar generation of electricity, high school graduation rates, and air quality. We interpret the models and discuss their implications for research and practice.
更多查看译文
关键词
Electric vehicles,Education,Solar power,Air quality,Gradient boosted trees,Regression
AI 理解论文
溯源树
样例
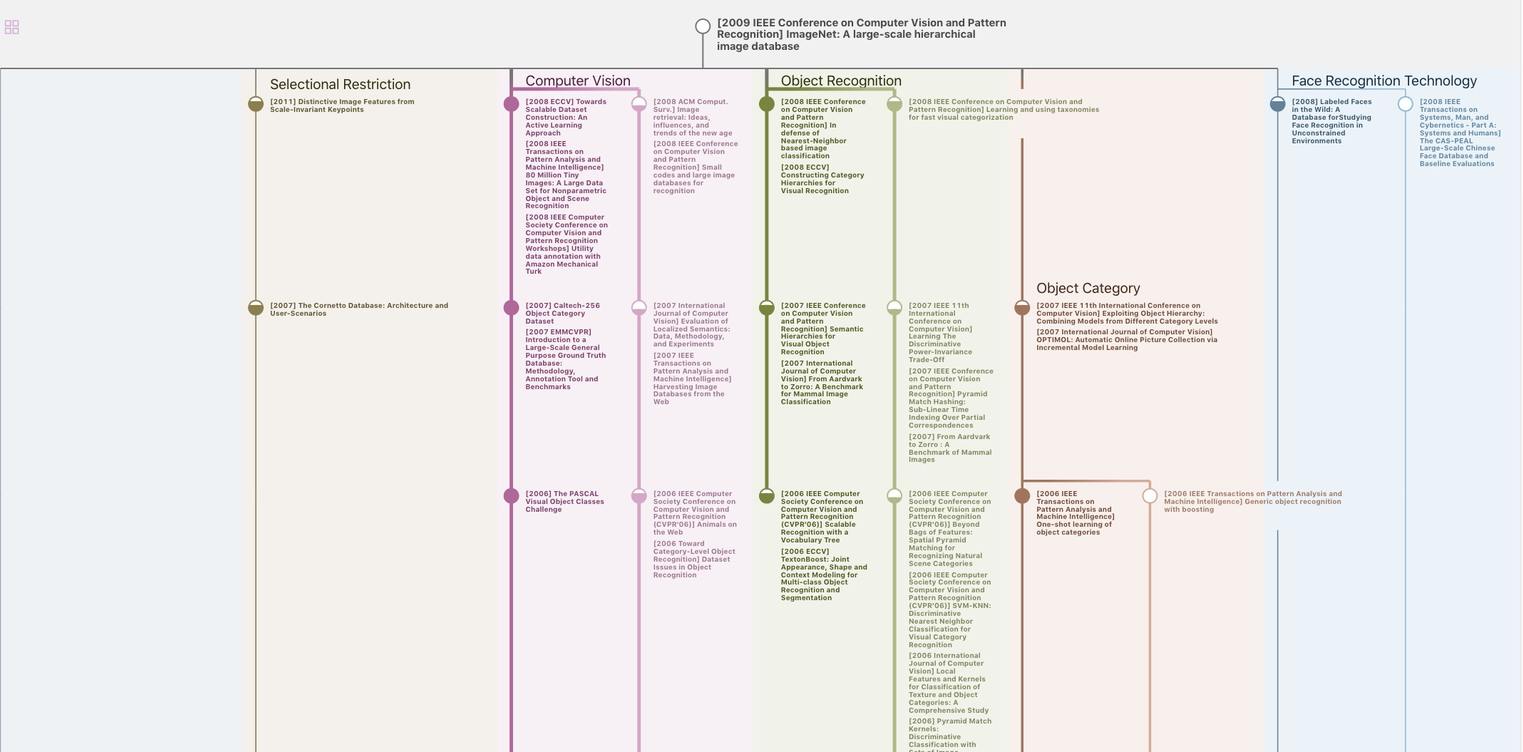
生成溯源树,研究论文发展脉络
Chat Paper
正在生成论文摘要