An Efficient and Resilient Technique for Malware Detection
2023 International Conference on the Confluence of Advancements in Robotics, Vision and Interdisciplinary Technology Management (IC-RVITM)(2023)
摘要
The escalating complexity and rising volume of malware threats have necessitated the development of sophisticated and efficient techniques for analyzing malicious software. Machine learning algorithms have exhibited considerable promise in this realm, automating the process of malware analysis and un-covering previously unidentified malware samples. This research paper delves into the utilization of the random forest algorithm in malware analysis, utilizing a numerical dataset comprising a diverse set of malware attributes to train and assess the model. We employed a pre-processed dataset and implemented feature selection to enhance the model’s performance. Our findings demonstrate that the random forest algorithm achieved exceptional accuracy in the identification of malware samples. Furthermore, we conducted a comparison of the efficacy of the random forest algorithm with other widely used machine learning techniques in the field of malware analysis. The results suggest that the random forest algorithm proves to be a robust and effective method for detecting malware, with potential for further refinement through the incorporation of deep learning methodologies. This research holds significant implications for advancing automated tools for malware analysis and can substantially contribute to the ongoing efforts to combat malware threats.
更多查看译文
关键词
Malware analysis,Feature selection,Feature extraction,Decision trees,Machine learning
AI 理解论文
溯源树
样例
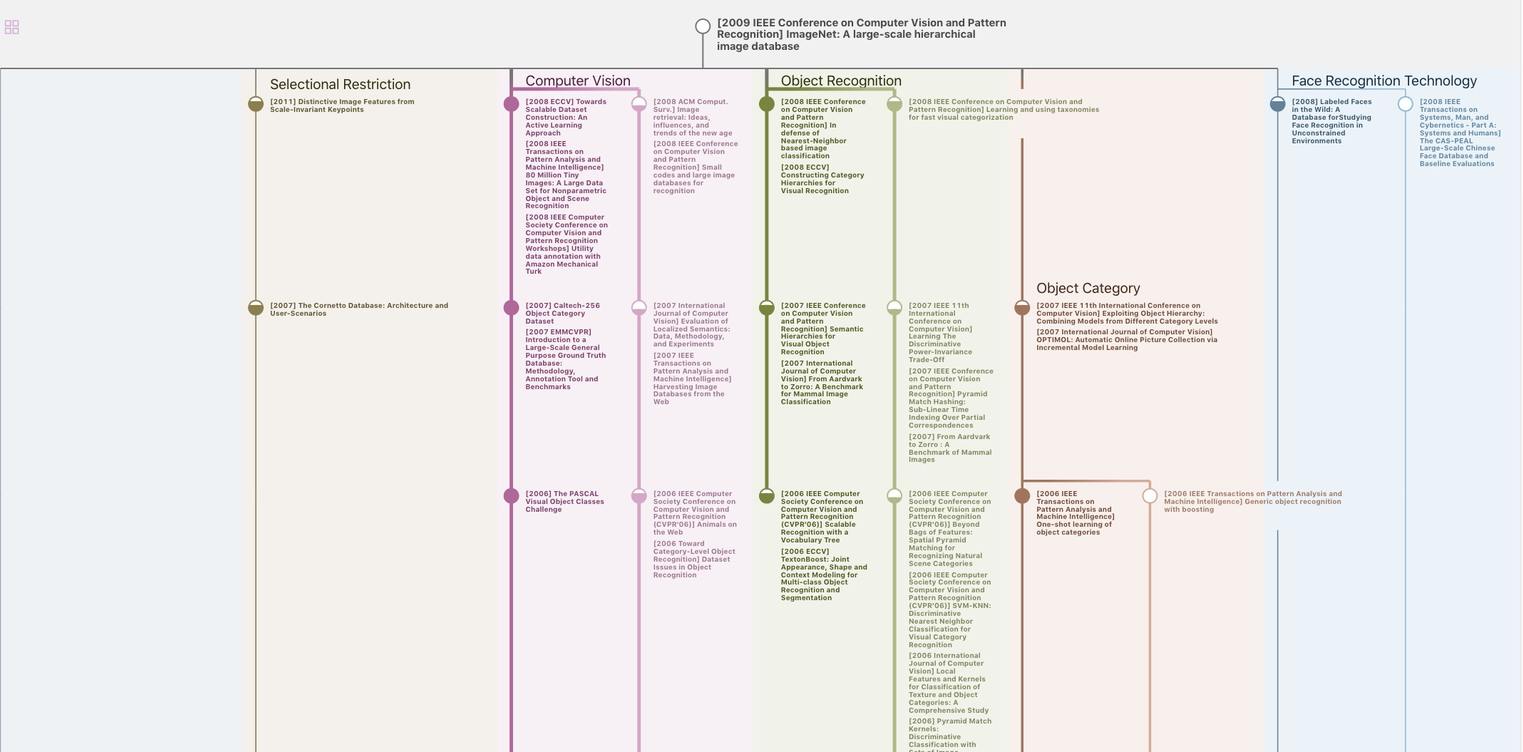
生成溯源树,研究论文发展脉络
Chat Paper
正在生成论文摘要