On the Prediction of Kidney Stone Formers
2023 IEEE International Conference on Technology Management, Operations and Decisions (ICTMOD)(2023)
Abstract
The early detection of kidney stone formers will significantly reduce treatment costs as well as the discomfort and inconvenience experienced by patients. We collected demographic data, health data and kidney stone information from 1220 individuals. Our objective was to determine how precisely we can predict if an individual will develop a kidney stone in the near future given their demographic and health data. We applied traditional machine learning methods, specifically Decision Tree, Random Forest, Naive Bayes, Logistic Regression Support Vector Machine and Perceptron, to perform this task and obtained reasonable results. We then applied a newly developed regression approach that works well on small data sets and found that it outperformed the traditional machine learning approaches. We believe that this new approach can be used to better predict kidney stone formers.
MoreTranslated text
Key words
Machine Learning,Data Science,Kidney Stone Formers
AI Read Science
Must-Reading Tree
Example
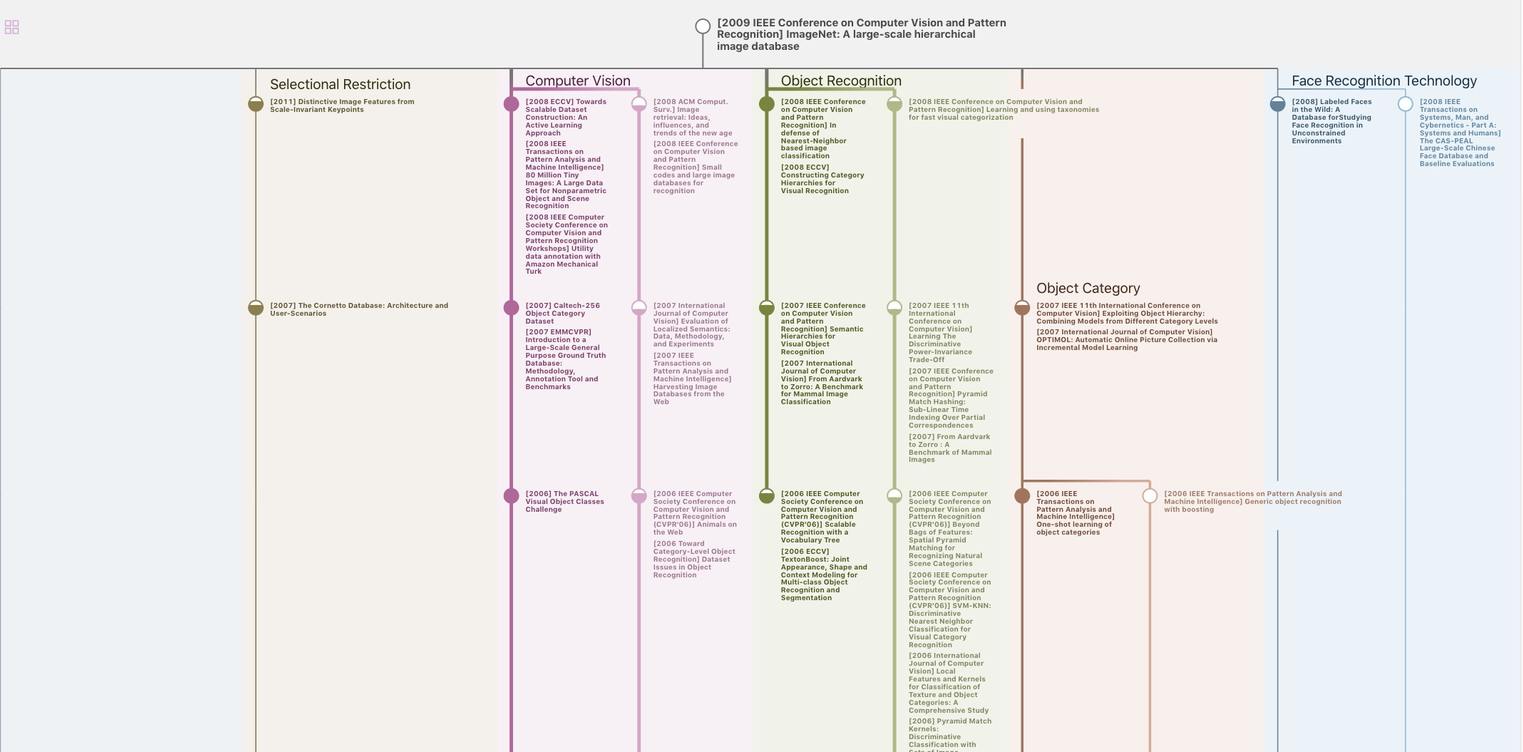
Generate MRT to find the research sequence of this paper
Chat Paper
Summary is being generated by the instructions you defined