Spectral Data Migration of Seawater COD Using Generative Adversarial Networks to Address Differences in Spectral Features and Insufficient Samples Across Regions
2023 3rd International Conference on Electronic Information Engineering and Computer Science (EIECS)(2023)
摘要
Chemical oxygen demand (COD) is an indicator of organic matter content in water, and its sensitive spectral regions depend on the species and concentrations of organics present. In recent years, spectroscopic analysis methods using feature variable modeling have become important for seawater COD detection. However, due to differences in COD compositions across regions and insufficient single-region samples, issues like varying spectral features and inadequate samples make data processing with deep learning difficult. To address this, we propose a seawater COD spectral data migration method using generative adversarial networks, aiming to resolve problems caused by varying COD compositions and insufficient samples across regions. Our method employs WGAN-GP for network training to achieve spectral data migration between regions. The improved WGAN-GP generator produces simulated data highly similar to real data, thereby enabling COD spectral data migration. This method can effectively address issues of varying spectral features and inadequate samples across regions, improving the accuracy and reliability of seawater COD spectral data analysis.
更多查看译文
关键词
Seawater COD,Spectral Data Migration,Generative Adversarial Networks,Varying Spectral Features
AI 理解论文
溯源树
样例
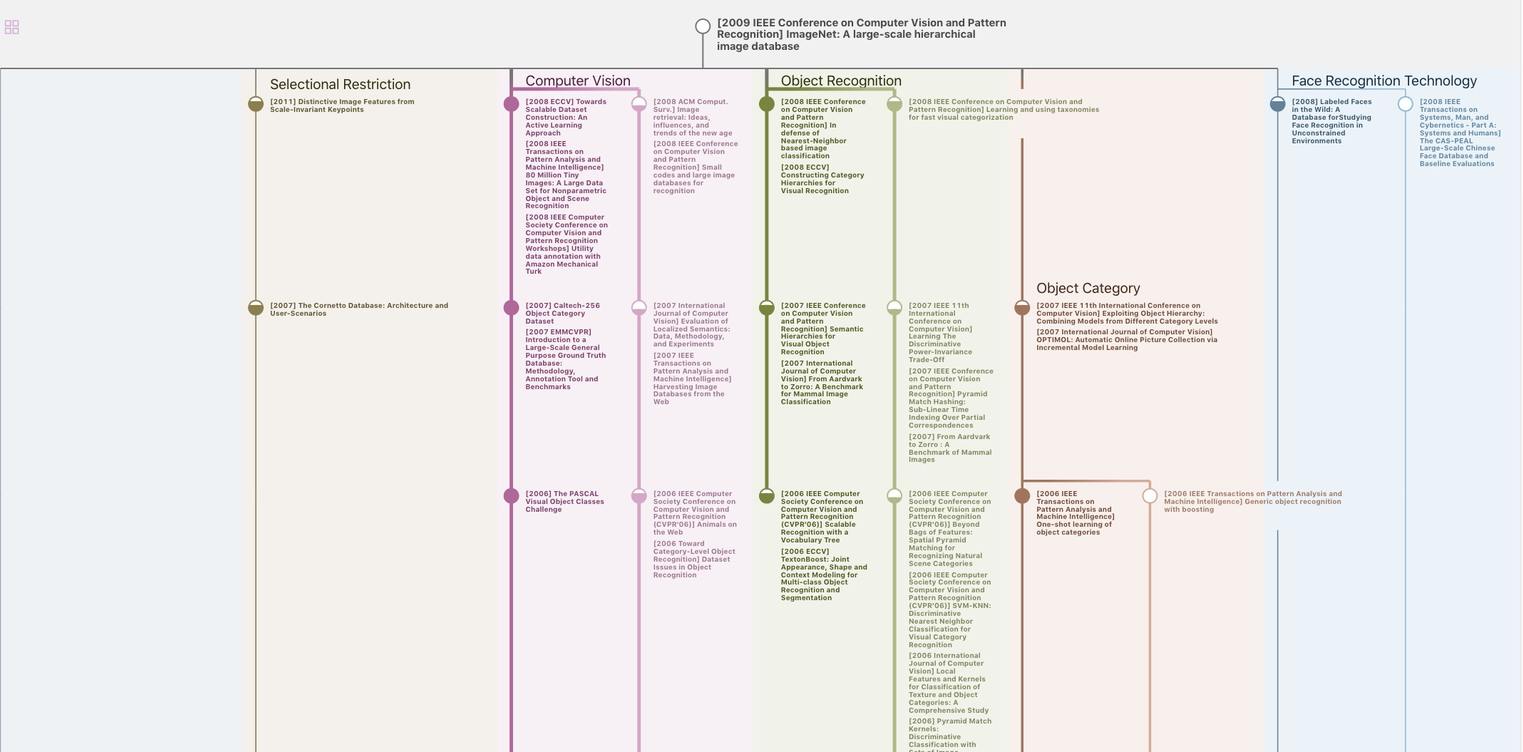
生成溯源树,研究论文发展脉络
Chat Paper
正在生成论文摘要