Property-Aware Relation Networks for Few-Shot Molecular Property Prediction.
IEEE transactions on pattern analysis and machine intelligence(2024)
摘要
Molecular property prediction plays a fundamental role in AI-aided drug discovery to identify candidate molecules, which is also essentially a few-shot problem due to lack of labeled data. In this paper, we propose Property-Aware Relation networks (PAR) to handle this problem. We first introduce a property-aware molecular encoder to transform the generic molecular embeddings to property-aware ones. Then, we design a query-dependent relation graph learning module to estimate molecular relation graph and refine molecular embeddings w.r.t. the target property. Thus, the facts that both property-related information and relationships among molecules change across different properties are utilized to better learn and propagate molecular embeddings. Generally, PAR can be regarded as a combination of metric-based and optimization-based few-shot learning method. We further extend PAR to Transferable PAR (T-PAR) to handle the distribution shift, which is common in drug discovery. The keys are joint sampling and relation graph learning schemes, which simultaneously learn molecular embeddings from both source and target domains. Extensive results on benchmark datasets show that PAR and T-PAR consistently outperform existing methods on few-shot and transferable few-shot molecular property prediction tasks, respectively. Besides, ablation and case studies are conducted to validate the rationality of our designs in PAR and T-PAR.
更多查看译文
关键词
Few-Shot Learning,Molecular Property Prediction,Meta-Learning,Transfer Learning
AI 理解论文
溯源树
样例
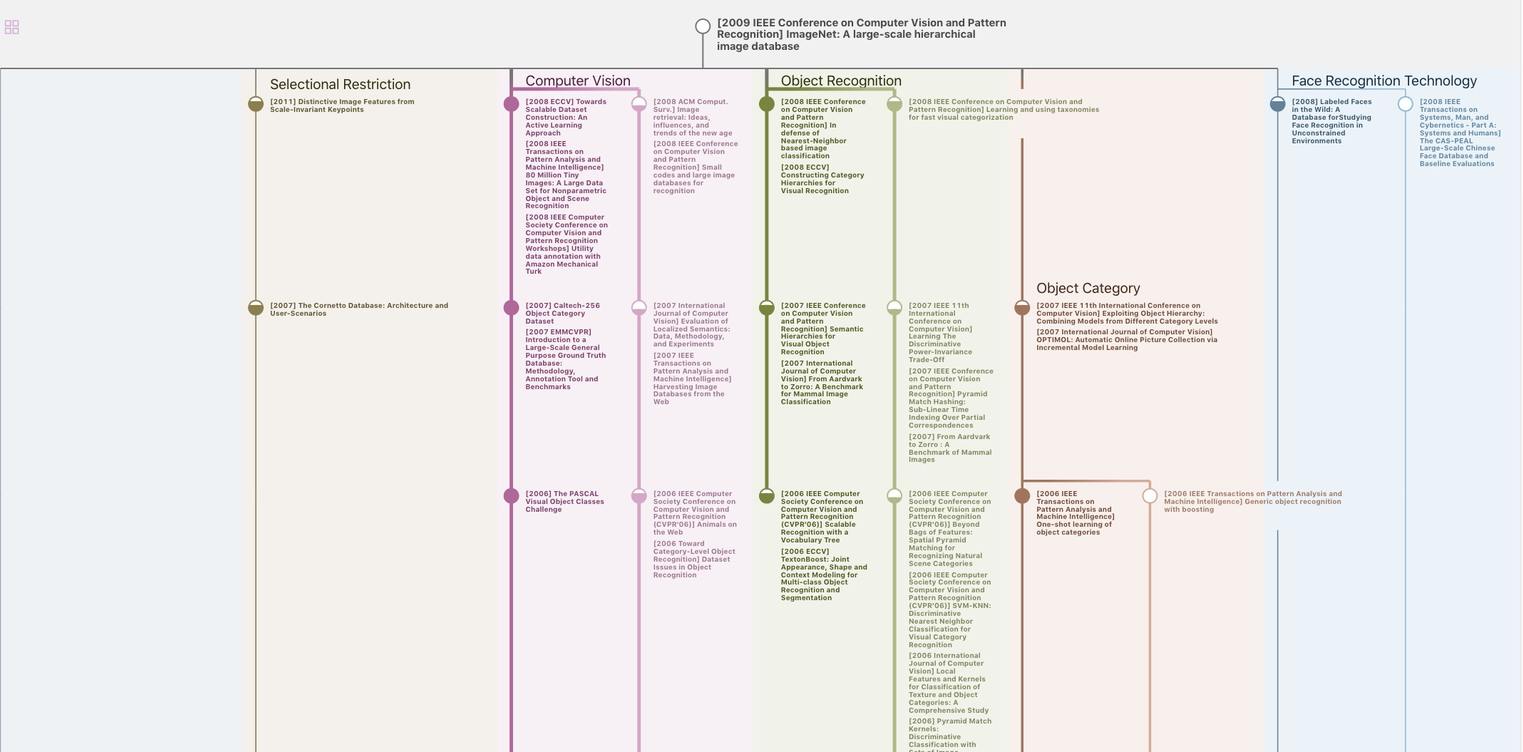
生成溯源树,研究论文发展脉络
Chat Paper
正在生成论文摘要