Suppressing HIFU Interference in Ultrasound Images Using 1D U-Net-based Neural Networks.
Physics in medicine & biology/Physics in medicine and biology(2024)
摘要
Objective.One big challenge with high-intensity focused ultrasound (HIFU) is that the intense acoustic interference generated by HIFU irradiation overwhelms the B-mode monitoring images, compromising monitoring effectiveness. This study aims to overcome this problem using a one-dimensional (1D) deep convolutional neural network.Approach. U-Net-based networks have been proven to be effective in image reconstruction and denoising, and the two-dimensional (2D) U-Net has already been investigated for suppressing HIFU interference in ultrasound monitoring images. In this study, we propose that the one-dimensional (1D) convolution in U-Net-based networks is more suitable for removing HIFU artifacts and can better recover the contaminated B-mode images compared to 2D convolution.Ex vivoandinvivoHIFU experiments were performed on a clinically equivalent ultrasound-guided HIFU platform to collect image data, and the 1D convolution in U-Net, Attention U-Net, U-Net++, and FUS-Net was applied to verify our proposal.Main results.All 1D U-Net-based networks were more effective in suppressing HIFU interference than their 2D counterparts, with over 30% improvement in terms of structural similarity (SSIM) to the uncontaminated B-mode images. Additionally, 1D U-Nets trained usingex vivodatasets demonstrated better generalization performance ininvivoexperiments.Significance.These findings indicate that the utilization of 1D convolution in U-Net-based networks offers great potential in addressing the challenges of monitoring in ultrasound-guided HIFU systems.
更多查看译文
关键词
high-intensity focused ultrasound (HIFU) interference,1D U-Net,HIFU therapy,US-guide HIFU,deep learning
AI 理解论文
溯源树
样例
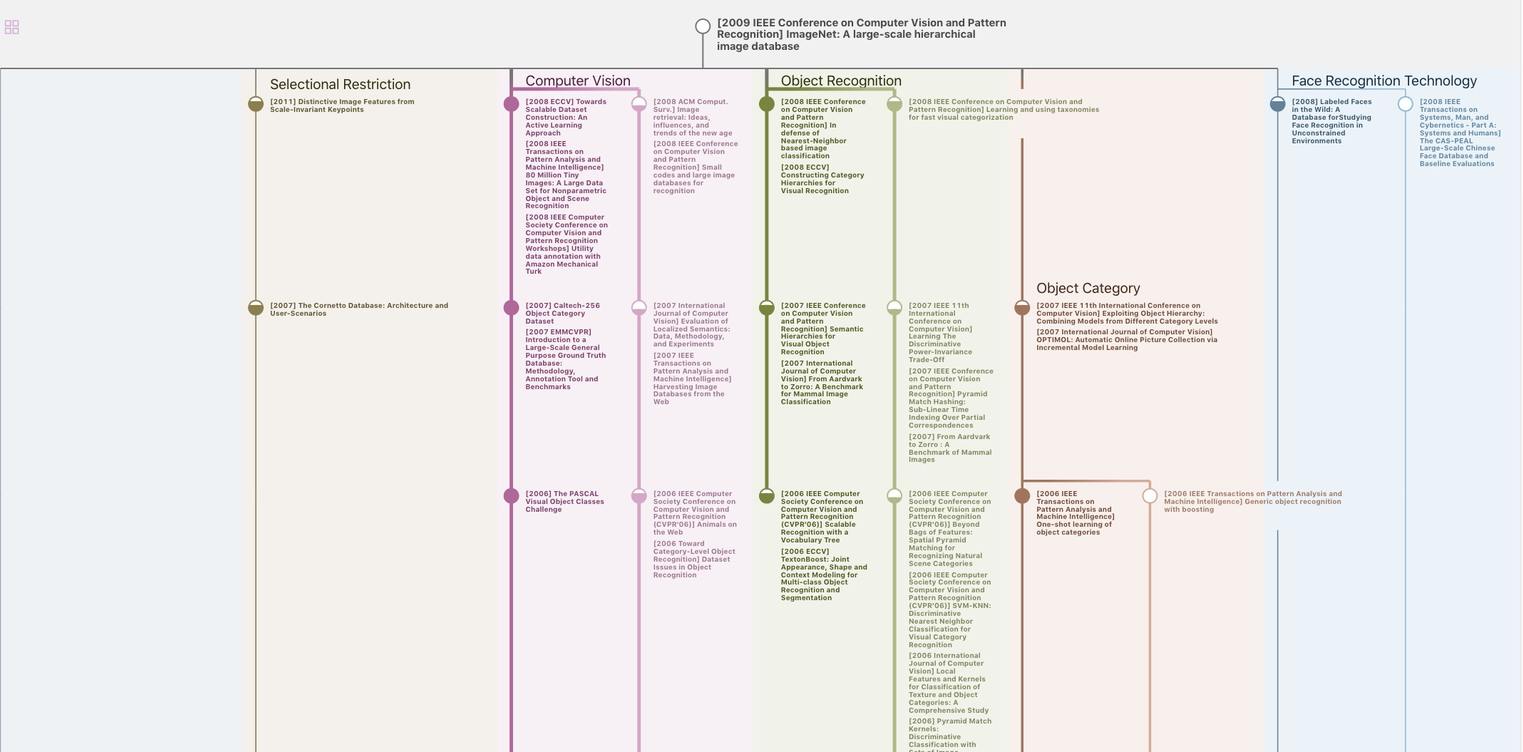
生成溯源树,研究论文发展脉络
Chat Paper
正在生成论文摘要