Compositional Design of Compounds with Elements Not in Training Data Using Supervised Learning
Journal of Materiomics(2024)
摘要
An issue of current interest in the use of machine learning models to predict compositions of materials is their reliability in predicting outcomes with elements not included in the training data. We show that the phase diagram of the ceramic (Ba1−x−yCaxSry)(Ti1−u−v−wZruSnvHfw)O3 can be accurately predicted if the feature values of unknown elements do not exceed the range of values for existing elements in the training data. In particular, we employ physical features as descriptors and compositions as weights to show that by excluding an element, such as Zr, Sn or Hf from the training set and treating it as an unknown element, the machine learning model accurately predicts the property only if the feature values of the unknown element does not exceed the range of values of existing elements in the training set. By adding a small amount of data for the unknown element restores the prediction accuracy. We demonstrate this for BaTiO3 ceramics doped with rare earth elements where the prediction accuracy is restored if the physical feature space is suitably enlarged with training data. The prediction error increases with the Euclidean distance of the testing sample relative to the nearest training sample in the physical feature space. Our work provides an effective strategy for extending machine learning models for material compositions beyond the scope of available data.
更多查看译文
关键词
Machine learning,Compositional design,BaTiO3,Phase diagram
AI 理解论文
溯源树
样例
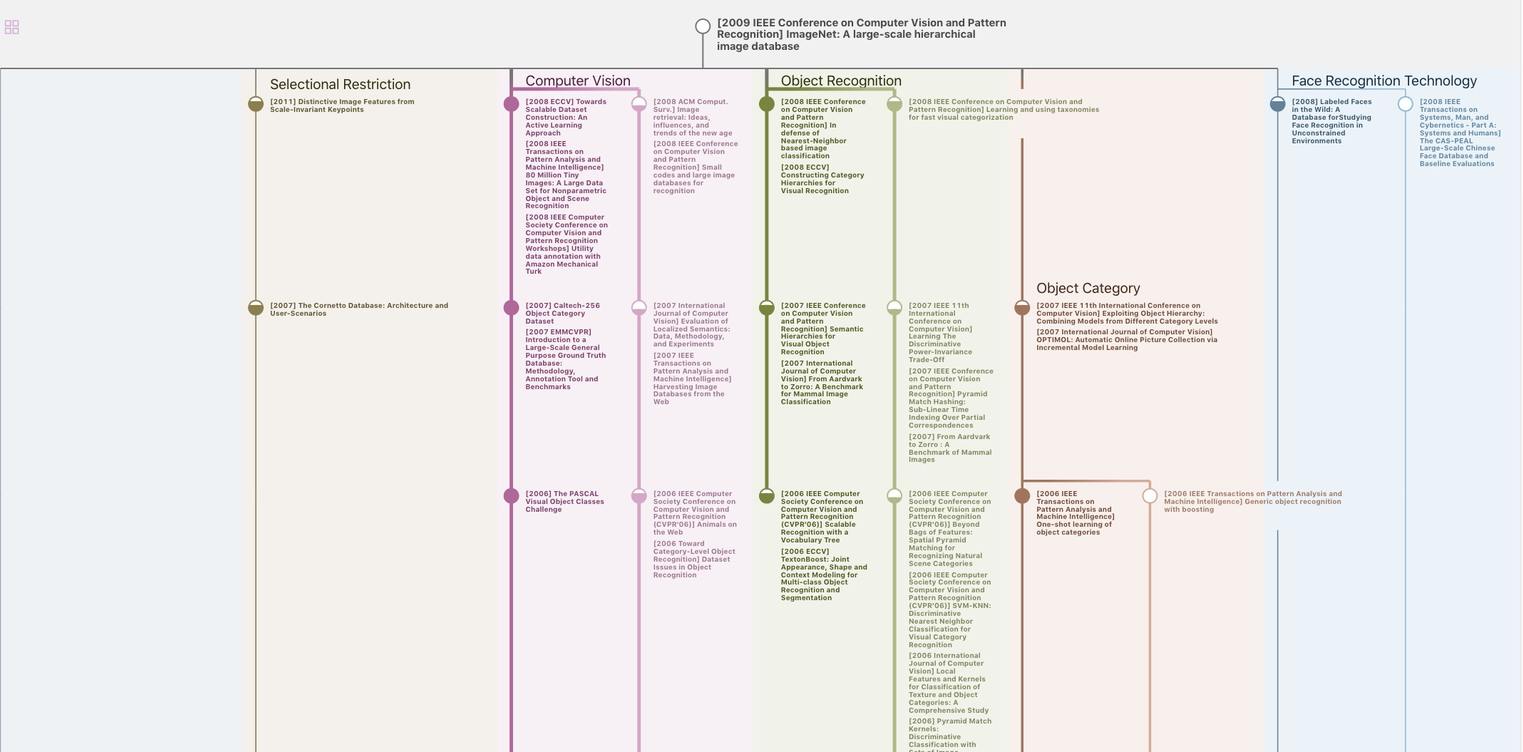
生成溯源树,研究论文发展脉络
Chat Paper
正在生成论文摘要