Dual Optical Design for VIS-NIR Classification based on Single Pixel and Diffractive Cameras
2023 IEEE Colombian Caribbean Conference (C3)(2023)
摘要
Spectral images are used in classification tasks from visible (VIS) and near-infrared (NIR) information of the underlying scene. However, traditional optical architectures that include spectral imaging sensors, require a high economic investment. Then, single pixel cameras (SPCs) with spectrometers have been included in spectral systems because of their low cost. These optical systems allow the acquisition of high-resolution spectral information and low-resolution spatial information. Additionally, diffractive optical cameras (DOCs) based on diffractive optical elements (DOEs) can acquire spectral and spatial information including phase modulation in the wavefront. The DOE is easily able to extract features from a scene for performing computational tasks. Then, this element can be designed from an end-to-end (E2E) optimization approach for classification. Traditional classification approaches have not simultaneously incorporated SPCs and DOCs, ignoring either spatial or spectral features. This work proposes a dual optical architecture for VIS-NIR acquisition in classification tasks: the first branch acquires the spectral information by an SPC, and the second branch acquires the spatial information by a DOC. Specifically, the height map in the DOE and the deep neural network (DNN) parameters are jointly learned from an E2E optimization. The proposed method contains an optical layer that describes the spectral system, a spectral imaging fusion layer that recovers the spatial-spectral information, and a classification neural network that labels the materials over spectral datasets. The proposed method obtained up to 4% in terms of accuracy, precision, recall, and F1 score above traditional optical setups including only CCD cameras.
更多查看译文
关键词
Spectral classification,single pixel camera,diffractive optical camera,diffractive optical element,end-to-end approach,deep neural networks
AI 理解论文
溯源树
样例
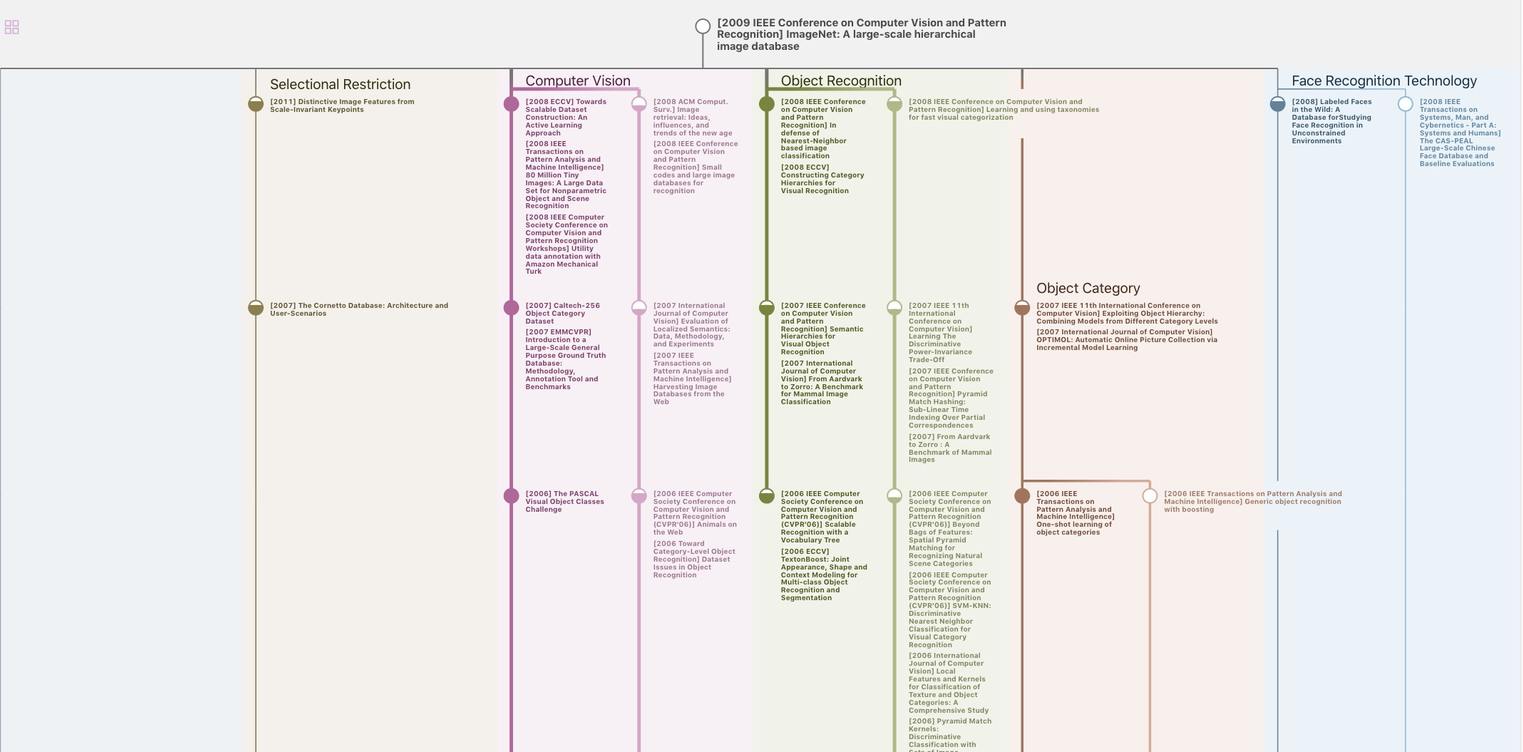
生成溯源树,研究论文发展脉络
Chat Paper
正在生成论文摘要