Quantum Markov Decision Processes Part II: Optimal Solutions and Algorithms
CoRR(2024)
摘要
This two-part article aims to introduce a quantum analogue to classical
Markov decision processes (MDPs). In Part II, building on the formulation of
q-MDPs presented in Part I, our focus shifts to the development of algorithms
for computing optimal policies and value functions of both open-loop and
closed-loop policies. First, by using the duality between the dynamic
programming and the semi-definite programming formulations of any q-MDP with
open-loop policies, we establish an algorithm that enables us to efficiently
compute optimal open-loop quantum policies and value functions. Then, dynamic
programming and semi-definite programming formulations for closed-loop policies
is established, where duality of these two formulations similarly enables the
efficient computation of optimal closed-loop policies and value functions.
Finally, given that any q-MDP can be approximated by q-MDPs with classical
policies–potentially with higher-dimensional underlying Hilbert spaces than
the original model–and since any classical policy is an element of the set of
closed-loop policies, we conclude that any q-MDP can be approximated by q-MDPs
with closed-loop policies having higher-dimensional Hilbert spaces.
更多查看译文
AI 理解论文
溯源树
样例
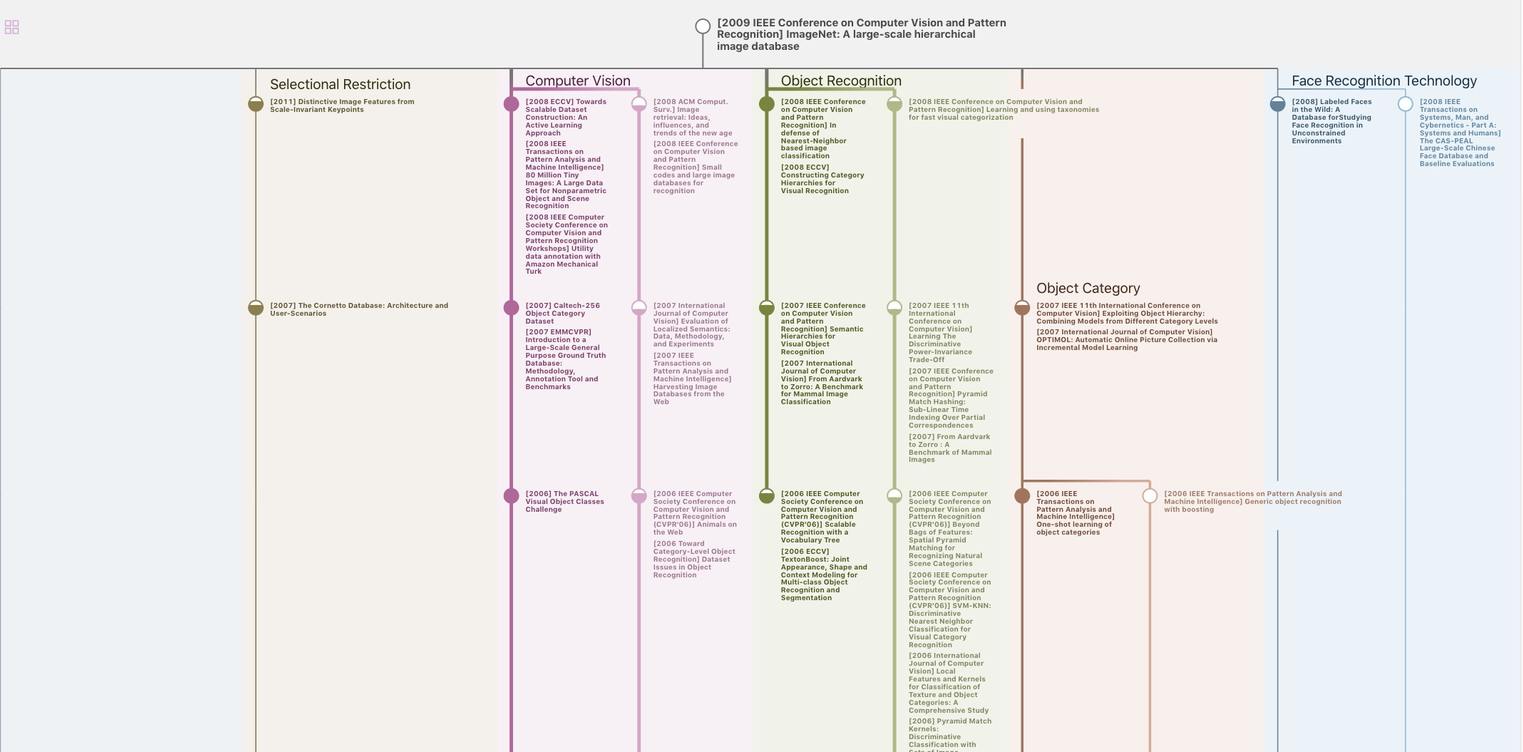
生成溯源树,研究论文发展脉络
Chat Paper
正在生成论文摘要