MAPE-PPI: Towards Effective and Efficient Protein-Protein Interaction Prediction Via Microenvironment-Aware Protein Embedding
ICLR 2024(2024)
摘要
Protein-Protein Interactions (PPIs) are fundamental in various biologicalprocesses and play a key role in life activities. The growing demand and costof experimental PPI assays require computational methods for efficient PPIprediction. While existing methods rely heavily on protein sequence for PPIprediction, it is the protein structure that is the key to determine theinteractions. To take both protein modalities into account, we define themicroenvironment of an amino acid residue by its sequence and structuralcontexts, which describe the surrounding chemical properties and geometricfeatures. In addition, microenvironments defined in previous work are largelybased on experimentally assayed physicochemical properties, for which the"vocabulary" is usually extremely small. This makes it difficult to cover thediversity and complexity of microenvironments. In this paper, we proposeMicroenvironment-Aware Protein Embedding for PPI prediction (MPAE-PPI), whichencodes microenvironments into chemically meaningful discrete codes via asufficiently large microenvironment "vocabulary" (i.e., codebook). Moreover, wepropose a novel pre-training strategy, namely Masked Codebook Modeling (MCM),to capture the dependencies between different microenvironments by randomlymasking the codebook and reconstructing the input. With the learnedmicroenvironment codebook, we can reuse it as an off-the-shelf tool toefficiently and effectively encode proteins of different sizes and functionsfor large-scale PPI prediction. Extensive experiments show that MAPE-PPI canscale to PPI prediction with millions of PPIs with superior trade-offs betweeneffectiveness and computational efficiency than the state-of-the-artcompetitors.
更多查看译文
关键词
Bioinformatics,Protein-Protein Interaction,Protein Sequence-Structure Co-Modeling
AI 理解论文
溯源树
样例
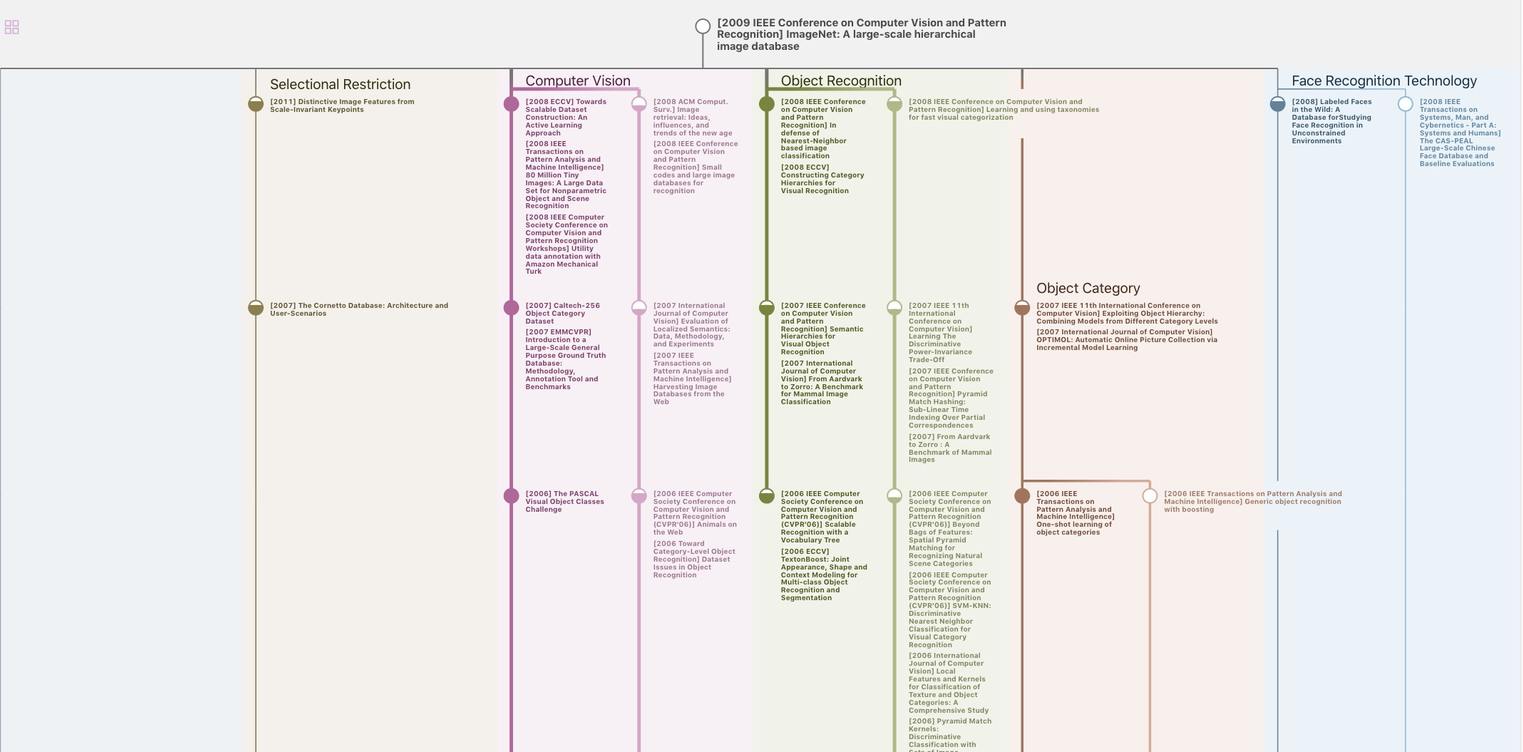
生成溯源树,研究论文发展脉络
Chat Paper
正在生成论文摘要