FrameNeRF: A Simple and Efficient Framework for Few-shot Novel View Synthesis
CoRR(2024)
摘要
We present a novel framework, called FrameNeRF, designed to apply
off-the-shelf fast high-fidelity NeRF models with fast training speed and high
rendering quality for few-shot novel view synthesis tasks. The training
stability of fast high-fidelity models is typically constrained to dense views,
making them unsuitable for few-shot novel view synthesis tasks. To address this
limitation, we utilize a regularization model as a data generator to produce
dense views from sparse inputs, facilitating subsequent training of fast
high-fidelity models. Since these dense views are pseudo ground truth generated
by the regularization model, original sparse images are then used to fine-tune
the fast high-fidelity model. This process helps the model learn realistic
details and correct artifacts introduced in earlier stages. By leveraging an
off-the-shelf regularization model and a fast high-fidelity model, our approach
achieves state-of-the-art performance across various benchmark datasets.
更多查看译文
AI 理解论文
溯源树
样例
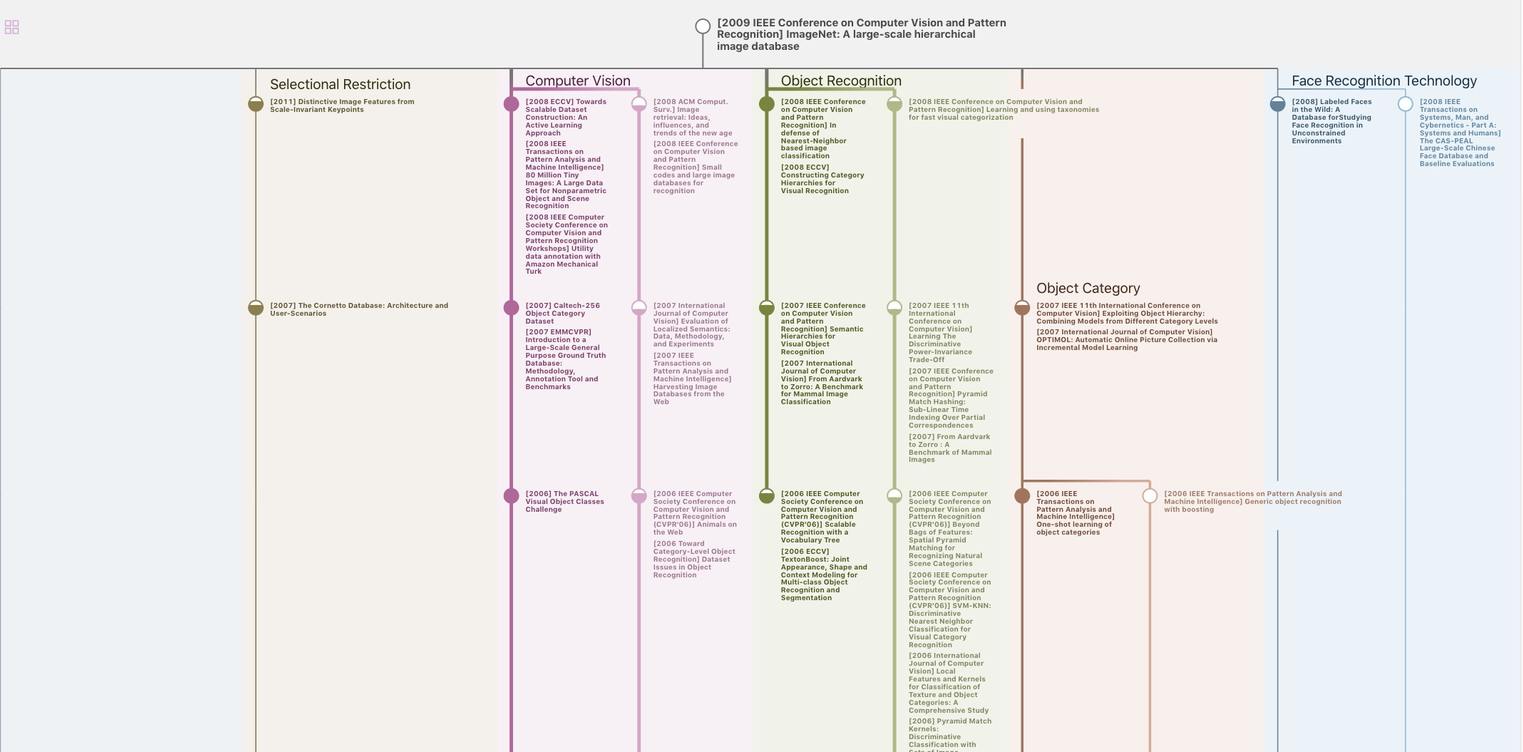
生成溯源树,研究论文发展脉络
Chat Paper
正在生成论文摘要