CriticBench: Benchmarking LLMs for Critique-Correct Reasoning
Findings of the Association for Computational Linguistics ACL 2024(2024)
摘要
The ability of Large Language Models (LLMs) to critique and refine theirreasoning is crucial for their application in evaluation, feedback provision,and self-improvement. This paper introduces CriticBench, a comprehensivebenchmark designed to assess LLMs' abilities to critique and rectify theirreasoning across a variety of tasks. CriticBench encompasses five reasoningdomains: mathematical, commonsense, symbolic, coding, and algorithmic. Itcompiles 15 datasets and incorporates responses from three LLM families.Utilizing CriticBench, we evaluate and dissect the performance of 17 LLMs ingeneration, critique, and correction reasoning, i.e., GQC reasoning. Ourfindings reveal: (1) a linear relationship in GQC capabilities, withcritique-focused training markedly enhancing performance; (2) a task-dependentvariation in correction effectiveness, with logic-oriented tasks being moreamenable to correction; (3) GQC knowledge inconsistencies that decrease asmodel size increases; and (4) an intriguing inter-model critiquing dynamic,where stronger models are better at critiquing weaker ones, while weaker modelscan surprisingly surpass stronger ones in their self-critique. We hope theseinsights into the nuanced critique-correct reasoning of LLMs will fosterfurther research in LLM critique and self-improvement.
更多查看译文
AI 理解论文
溯源树
样例
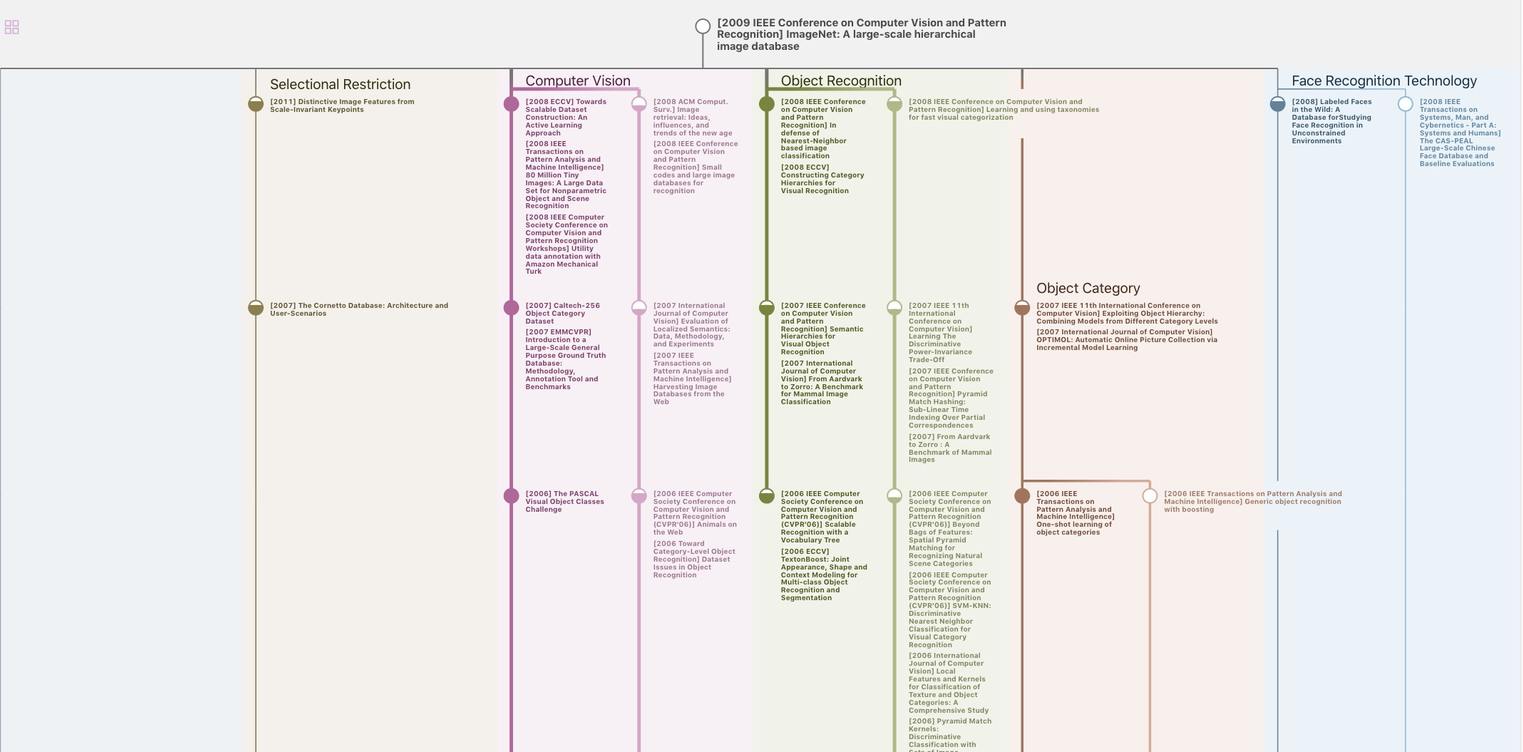
生成溯源树,研究论文发展脉络
Chat Paper
正在生成论文摘要