Machine Learning Approaches in Label-Free Small Extracellular Vesicles Analysis with Surface-Enhanced Raman Scattering (SERS) for Cancer Diagnostics
biorxiv(2024)
摘要
Early diagnosis remains of pivotal importance in reducing patient morbidity and mortality in cancer. To this end, liquid biopsy is emerging as a tool to perform broad cancer screenings. Small extracellular vesicles (sEVs) found in bodily fluids can serve as important cancer biomarkers in these screenings. Our group has recently developed a nanopipette dielectrophoretic approach to extract sEVs from blood. Herein, we demonstrate the feasibility to integrate this approach with surface-enhanced Raman scattering (SERS) analysis. SERS can be used to characterized extracted exosomes through their vibrational fingerprint that changes depending on the origin of sEVs. While these changes are not easily identified in spectra, they can be modeled with machine learning (ML) approaches. Common ML approaches in the field of spectral analysis use dimensionality reduction method that often function as a black box. To avoid this pitfall, we used Shapley additive explanations (SHAP) is a type of explainable AI (XAI) that bridges ML models and human comprehension by calculating the specific contribution of individual features to a model predictions, directly correlating model/decisions with the original data. Using these approaches we demonstrated a proof-of-concept model predictive of cancer from isolated exosomes, integrating nanopipette dielectrophoresis and SERS. This work demonstrates the use of explainable AI to perform diagnostic analysis on complex SERS data of clinical samples, while reporting interpretable biochemical information.
### Competing Interest Statement
The authors have declared no competing interest.
更多查看译文
AI 理解论文
溯源树
样例
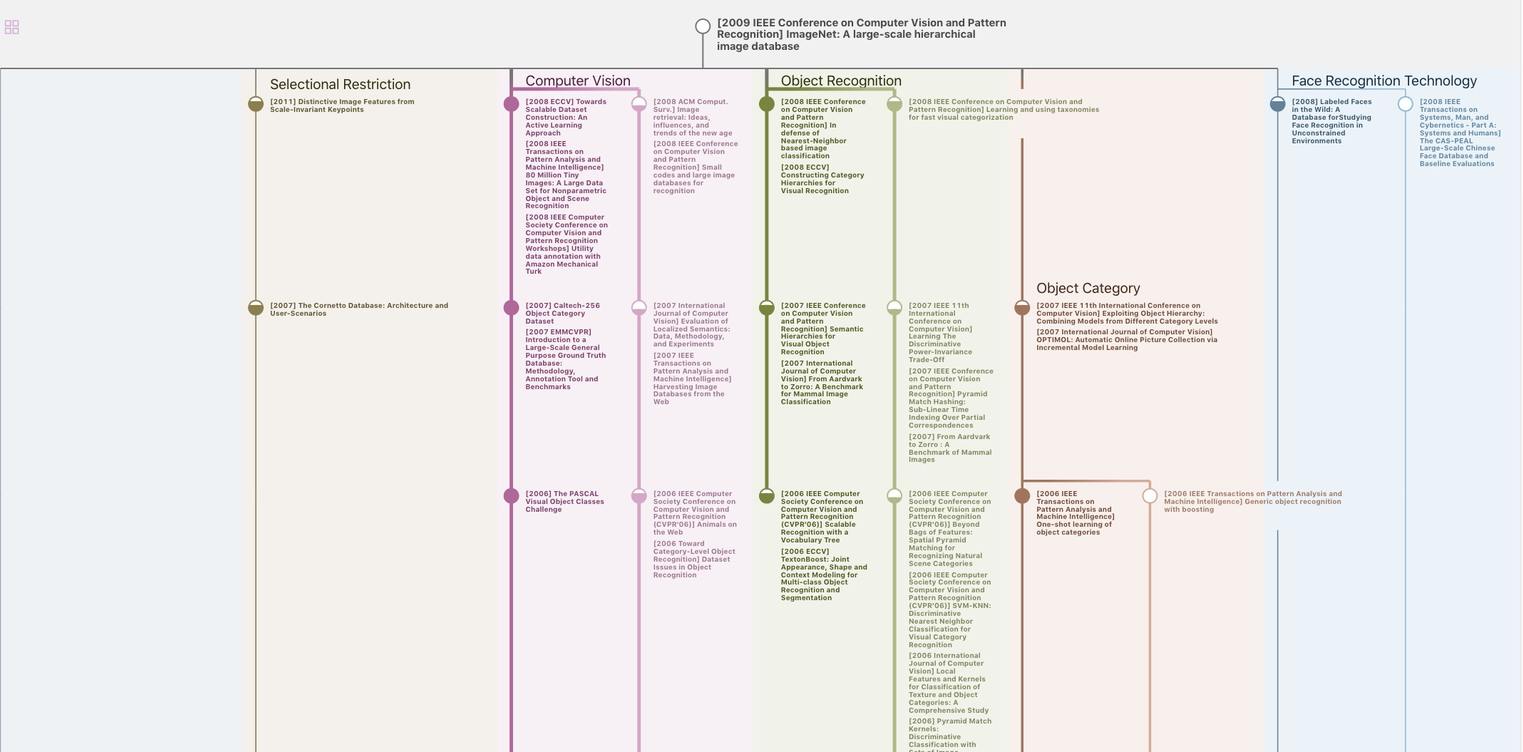
生成溯源树,研究论文发展脉络
Chat Paper
正在生成论文摘要