One-vs-Rest vs. Voting Classifiers for Multi-Label Text Classification: An Empirical Study
E3S Web of Conferences(2024)
摘要
Inthispaper,weconductanempiricalstudytocompare the performance of two popular approaches for multi-label text classification, which is a challenging task in naturallanguageprocessingthatrequirespredictingmultiplelabelsforagiventext:One-vs-RestandVotingclassifiers.Weevaluatetheseclassifiersonadatasetoftoxiccommentsandmeasuretheir performance using accuracy and hamming loss evaluationmetrics.OurexperimentalresultsshowthattheOne-vs-RestclassifierwithXGBoutperformstheVotingclassifierandachievesanaccuracyof91.7%.Thestudy’sresultscanbeusedasabenchmarkforfutureresearchinthisarea,andtheinsightsgained can be used to improve the accuracy and robustness ofmulti-label text classification models. Furthermore, our findingssuggest that the One-vs-Rest classifier with XGB is a promisingapproachformulti-labeltextclassificationtasks,whichcanprovidebetterresultsthanotherpopularclassifiers
更多查看译文
AI 理解论文
溯源树
样例
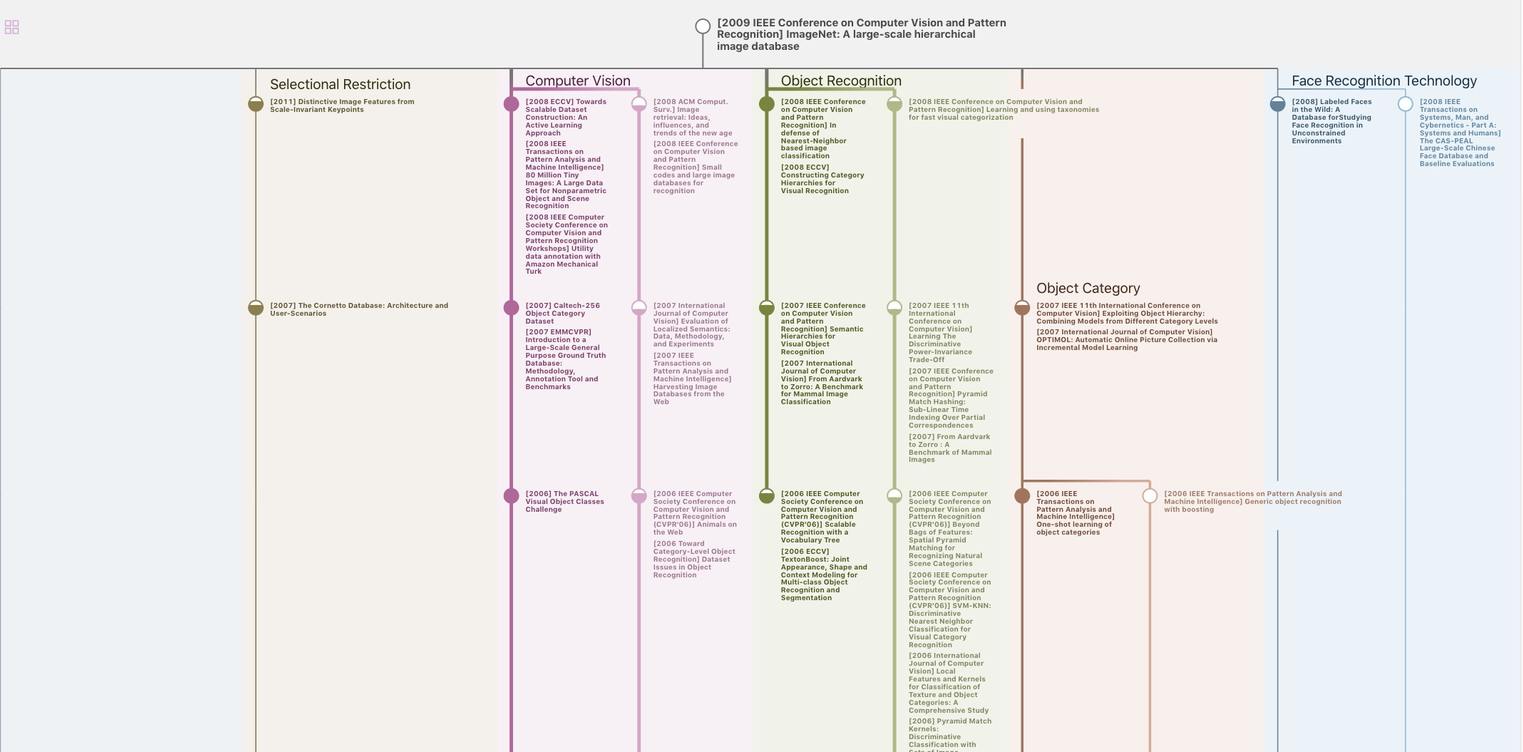
生成溯源树,研究论文发展脉络
Chat Paper
正在生成论文摘要