Water Quality Inversion of a Typical Rural Small River in Southeastern China Based on UAV Multispectral Imagery: A Comparison of Multiple Machine Learning Algorithms
WATER(2024)
摘要
Remote sensing technology applications for water quality inversion in large rivers are common. However, their application to medium/small-sized water bodies within rural areas is limited due to the low spatial resolution of remote sensing images. In this work, a typical small rural river was selected, and high-resolution unmanned aerial vehicle (UAV) multispectral images and ground monitoring data of the river were obtained. Then, a comparative analysis of three univariate regression models and nine machine learning models (Ridge Regression (RR), Support Vector Regression (SVR), Grid Search Support Vector Regression (GS-SVR), Random Forest (RF), Grid Search Random Forest (GS-RF), eXtreme Gradient Boosting (XGBoost), Deep Neural Networks (DNN), Convolutional Neural Networks (CNN), and Catboost Regression (CBR)) for their accuracy in the prediction of turbidity (TUB), total nitrogen (TN), and total phosphorus (TP) was performed. TUB can be achieved by simple statistical regression models. The CBR model exhibited the best performance for the three index inversions on the test set evaluation metrics: R-2 (0.90 similar to 0.92), RMSE (7.57 x 10(-3)similar to 1.59 mg/L), MAE (0.01 similar to 1.30 mg/L), RPD (3.21 similar to 3.56), and NSE (0.84 similar to 0.92). The water pollution of the study area was closely related to its land-use pattern, excessive and irrational fertilizer application, and distribution of pollutant outlets.
更多查看译文
关键词
UAV multispectral data,Catboost Regression,total nitrogen and total phosphorus,turbidity,medium/small-sized water bodies,small sample size
AI 理解论文
溯源树
样例
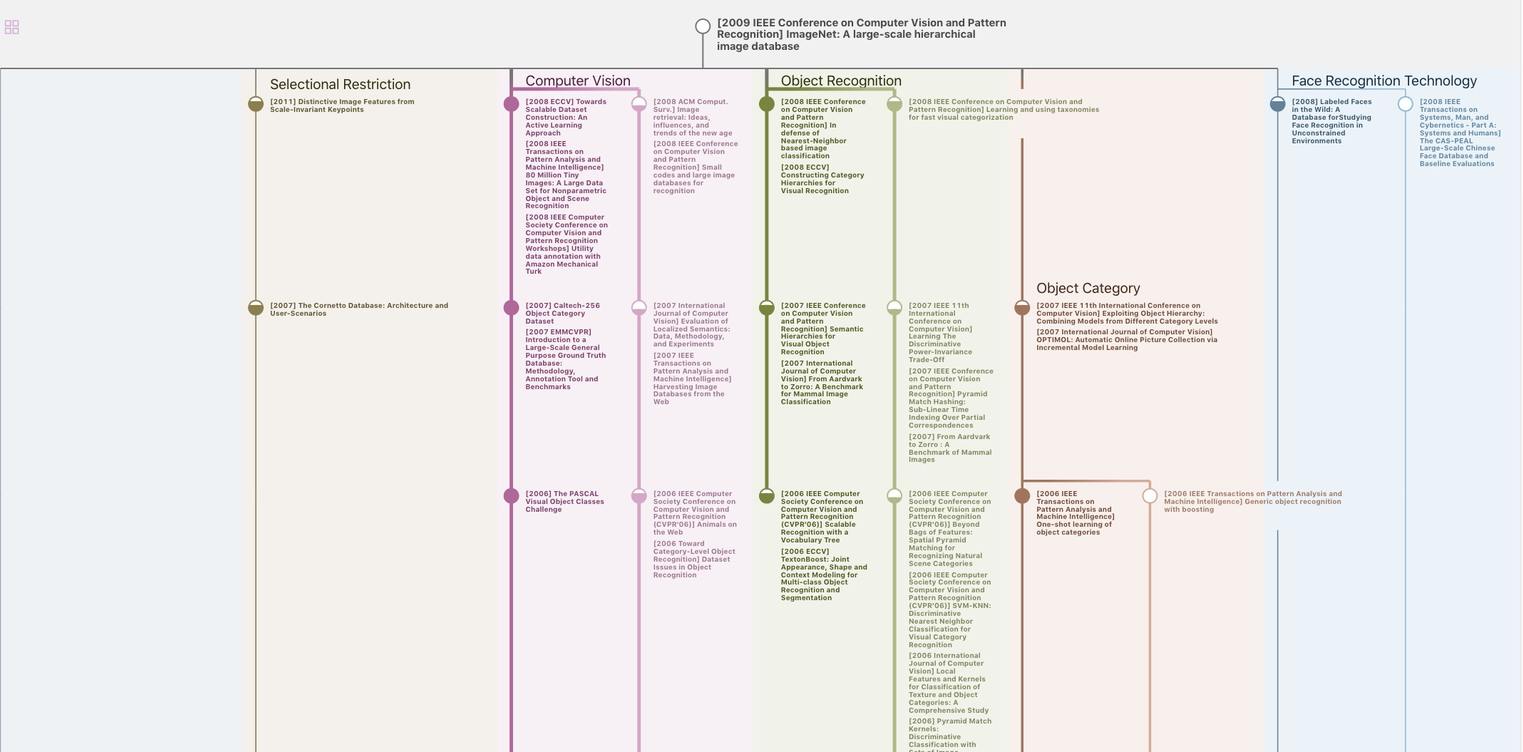
生成溯源树,研究论文发展脉络
Chat Paper
正在生成论文摘要