Land-Use Change Prediction in Dam Catchment Using Logistic Regression-CA, ANN-CA and Random Forest Regression and Implications for Sustainable Land-Water Nexus
SUSTAINABILITY(2024)
摘要
For sustainable water resource management within dam catchments, accurate knowledge of land-use and land-cover change (LULCC) and the relationships with dam water variability is necessary. To improve LULCC prediction, this study proposes the use of a random forest regression (RFR) model, in comparison with logistic regression-cellular automata (LR-CA) and artificial neural network-cellular automata (ANN-CA), for the prediction of LULCC (2019-2030) in the Gaborone dam catchment (Botswana). RFR is proposed as it is able to capture the existing and potential interactions between the LULC intensity and their nonlinear interactions with the change-driving factors. For LULCC forecasting, the driving factors comprised physiographic variables (elevation, slope and aspect) and proximity-neighborhood factors (distances to water bodies, roads and urban areas). In simulating the historical LULC (1986-2019) at 5-year time steps, RFR outperformed ANN-CA and LR-CA models with respective percentage accuracies of 84.9%, 62.1% and 60.7%. Using the RFR model, the predicted LULCCs were determined as vegetation (-8.9%), bare soil (+8.9%), built-up (+2.49%) and cropland (-2.8%), with water bodies exhibiting insignificant change. The correlation between land use (built-up areas) and water depicted an increasing population against decreasing dam water capacity. The study approach has the potential for deriving the catchment land-water nexus, which can aid in the formulation of sustainable catchment monitoring and development strategies.
更多查看译文
关键词
land-use land-cover (LULC) change,logistic regression,artificial neural network,cellular automata,random forest regression,sustainable land-water nexus
AI 理解论文
溯源树
样例
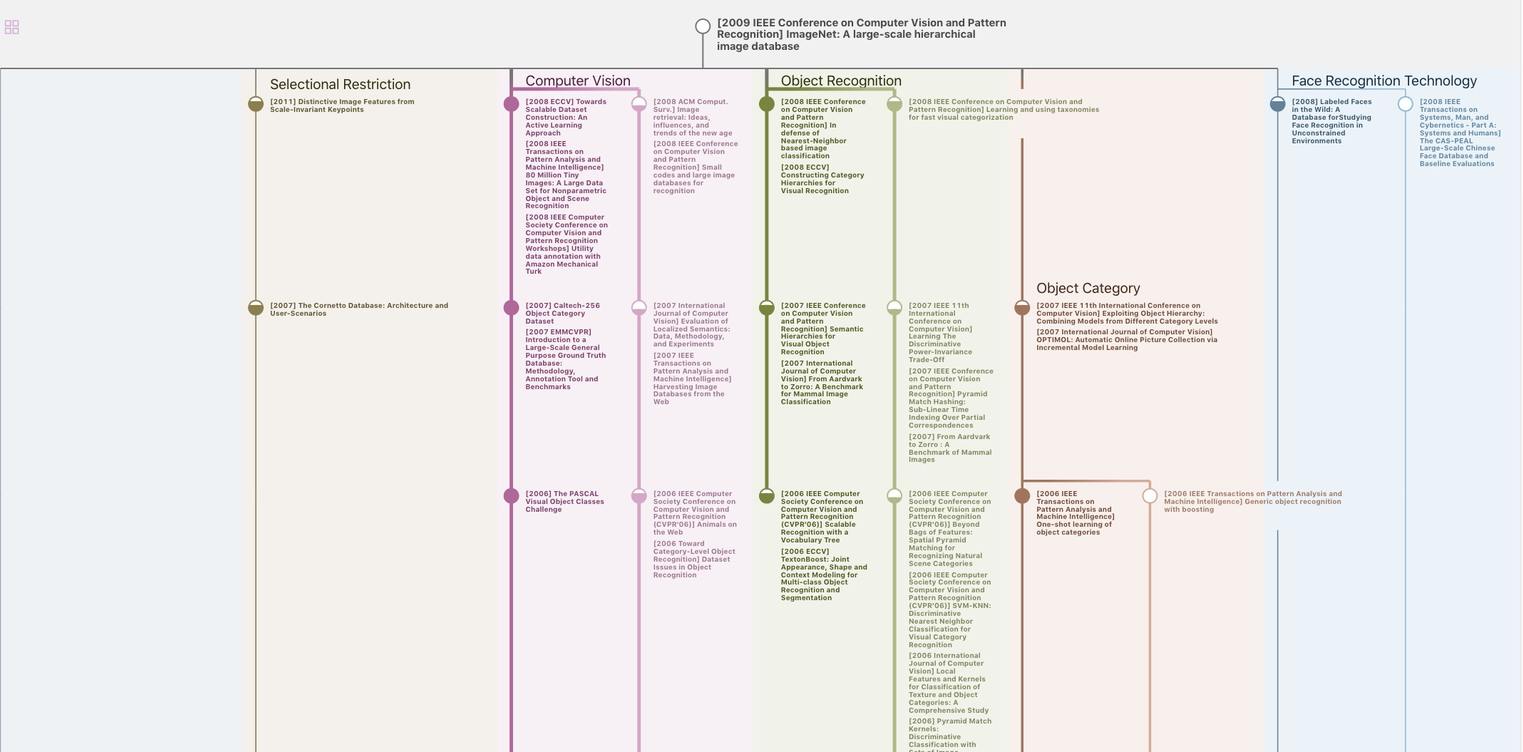
生成溯源树,研究论文发展脉络
Chat Paper
正在生成论文摘要