Adversarial Robustness with Partial Isometry
Entropy(2024)
Abstract
Despite their remarkable performance, deep learning models still lack robustness guarantees, particularly in the presence of adversarial examples. This significant vulnerability raises concerns about their trustworthiness and hinders their deployment in critical domains that require certified levels of robustness. In this paper, we introduce an information geometric framework to establish precise robustness criteria for l2 white-box attacks in a multi-class classification setting. We endow the output space with the Fisher information metric and derive criteria on the input–output Jacobian to ensure robustness. We show that model robustness can be achieved by constraining the model to be partially isometric around the training points. We evaluate our approach using MNIST and CIFAR-10 datasets against adversarial attacks, revealing its substantial improvements over defensive distillation and Jacobian regularization for medium-sized perturbations and its superior robustness performance to adversarial training for large perturbations, all while maintaining the desired accuracy.
MoreTranslated text
Key words
adversarial robustness,information geometry,fisher information metric,multi-class classification
AI Read Science
Must-Reading Tree
Example
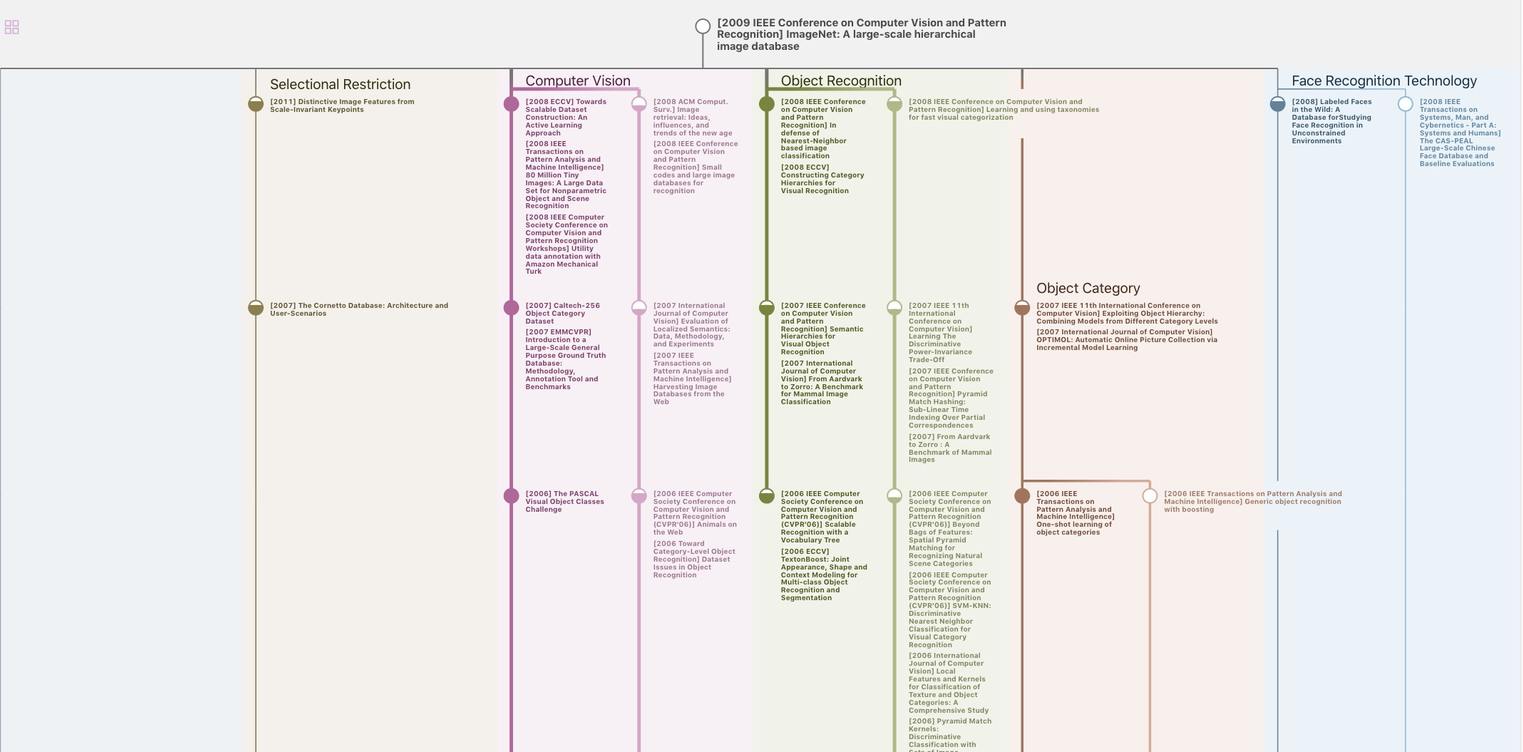
Generate MRT to find the research sequence of this paper
Chat Paper
Summary is being generated by the instructions you defined