Evaluating the effectiveness of pre-operative diagnosis of ovarian cancer using minimally invasive liquid biopsies by combining serum human epididymis protein 4 and cell-free DNA in patients with an ovarian mass
INTERNATIONAL JOURNAL OF GYNECOLOGICAL CANCER(2024)
摘要
Objective To assess the feasibility of scalable, objective, and minimally invasive liquid biopsy-derived biomarkers such as cell-free DNA copy number profiles, human epididymis protein 4 (HE4), and cancer antigen 125 (CA125) for pre-operative risk assessment of early-stage ovarian cancer in a clinically representative and diagnostically challenging population and to compare the performance of these biomarkers with the Risk of Malignancy Index (RMI). Methods In this case-control study, we included 100 patients with an ovarian mass clinically suspected to be early-stage ovarian cancer. Of these 100 patients, 50 were confirmed to have a malignant mass (cases) and 50 had a benign mass (controls). Using WisecondorX, an algorithm used extensively in non-invasive prenatal testing, we calculated the benign-calibrated copy number profile abnormality score. This score represents how different a sample is from benign controls based on copy number profiles. We combined this score with HE4 serum concentration to separate cases and controls. Results Combining the benign-calibrated copy number profile abnormality score with HE4, we obtained a model with a significantly higher sensitivity (42% vs 0%; p<0.002) at 99% specificity as compared with the RMI that is currently employed in clinical practice. Investigating performance in subgroups, we observed especially large differences in the advanced stage and non-high-grade serous ovarian cancer groups. Conclusion This study demonstrates that cell-free DNA can be successfully employed to perform pre-operative risk of malignancy assessment for ovarian masses; however, results warrant validation in a more extensive clinical study.
更多查看译文
关键词
Area Under Curve,Carcinoma, Ovarian Epithelial,Ovarian Cancer,Ovarian Neoplasms
AI 理解论文
溯源树
样例
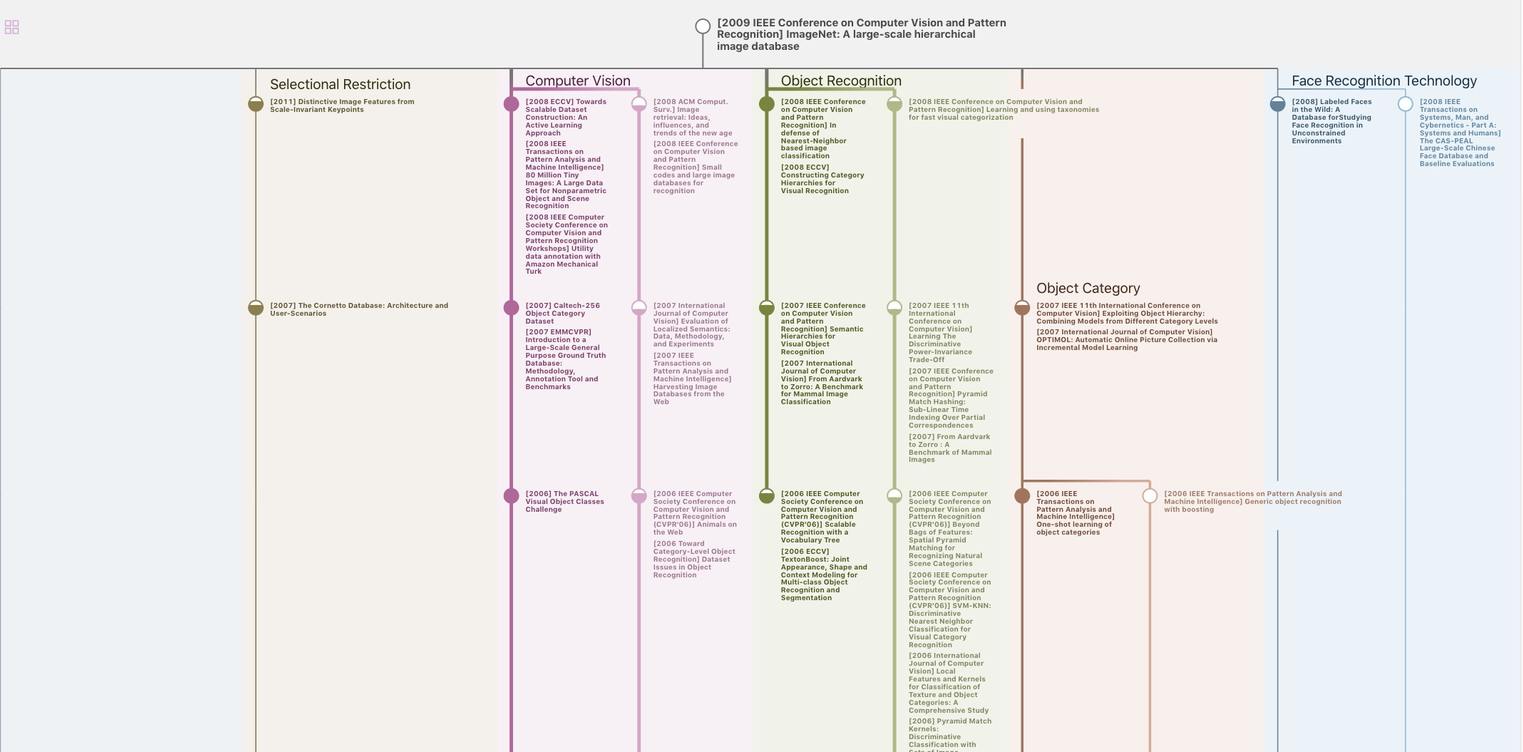
生成溯源树,研究论文发展脉络
Chat Paper
正在生成论文摘要