Deep learning model for the detection of prostate cancer and classification of clinically significant disease using multiparametric MRI in comparison to PI-RADs score.
Urologic oncology(2024)
摘要
BACKGROUND:The Prostate Imaging Reporting and Data System (PI-RADS) is an established reporting scheme for multiparametric magnetic resonance imaging (mpMRI) to distinguish clinically significant prostate cancer (csPCa). Deep learning (DL) holds great potential for automating csPCa classification on mpMRI.
METHOD:To compare the performance between a DL algorithm and PI-RADS categorization in PCa detection and csPCa classification, we included 1,729 consecutive patients who underwent radical prostatectomy or biopsy in Tongji hospital. We developed DL models by integrating individual mpMRI sequences and employing an ensemble approach for distinguishing between csPCa and CiSPCa (specifically defined as PCa with a Gleason group 1 or benign prostate disease, training cohort: 1,285 patients vs. external testing cohort: 315 patients).
RESULTS:DL-based models exhibited higher csPCa detection rates than PI-RADS categorization (area under the curve [AUC]: 0.902; sensitivity: 0.728; specificity: 0.906 vs. AUC: 0.759; sensitivity: 0.761; specificity: 0.756) (P < 0.001) Notably, DL networks exhibited significant strength in the prostate-specific antigen (PSA) arm < 10 ng/ml compared with PI-RADS assessment (AUC: 0.788; sensitivity: 0.588; specificity: 0.883 vs. AUC: 0.618; sensitivity: 0.379; specificity: 0.763) (P = 0.041).
CONCLUSIONS:We developed DL-based mpMRI ensemble models for csPCa classification with improved sensitivity, specificity, and accuracy compared with clinical PI-RADS assessment. In the PSA-stratified condition, the DL ensemble model performed better than PI-RADS in the detection of csPCa in both the high PSA group and the low PSA group.
更多查看译文
AI 理解论文
溯源树
样例
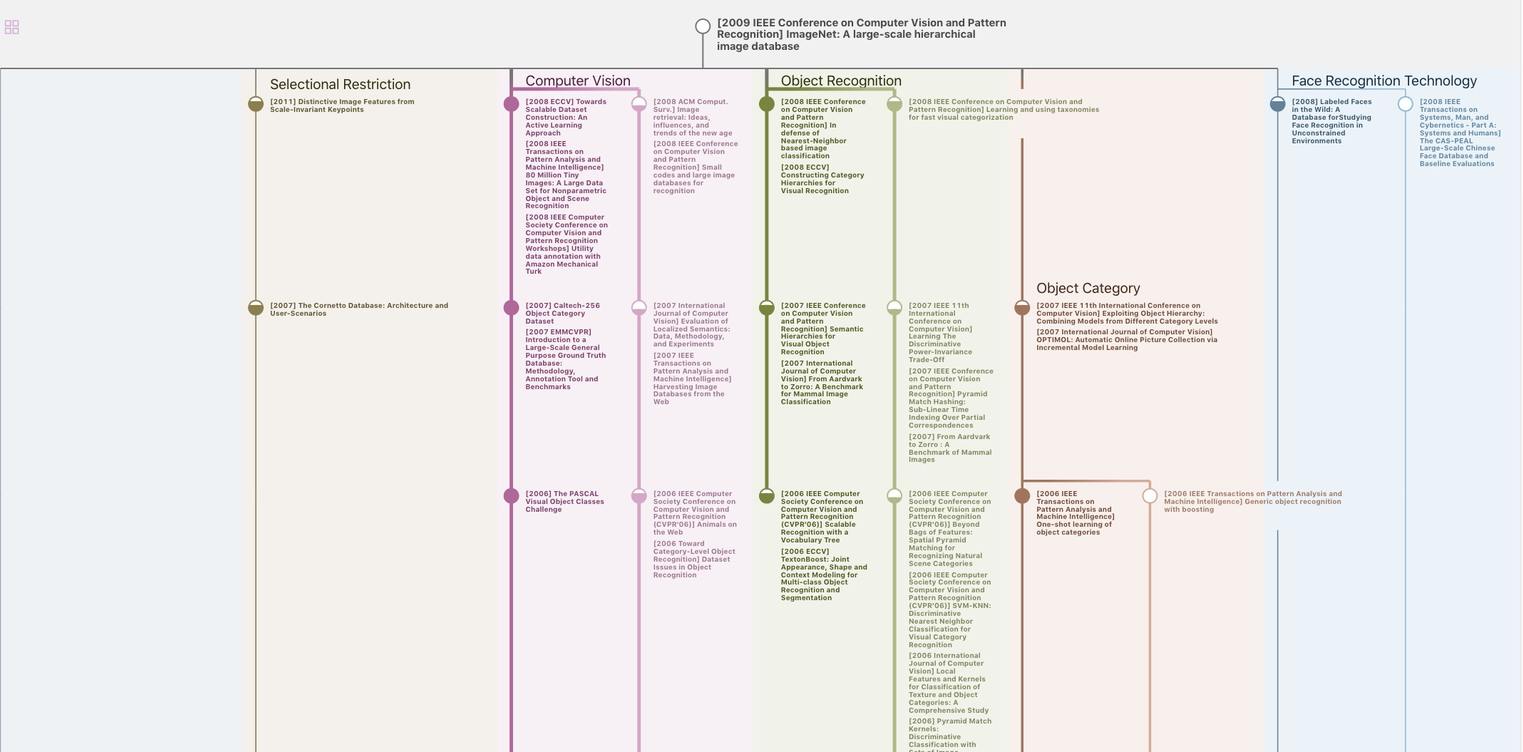
生成溯源树,研究论文发展脉络
Chat Paper
正在生成论文摘要