Automated Restarting Fast Proximal Gradient Descent Method for Single-View Cone-Beam X-ray Luminescence Computed Tomography Based on Depth Compensation
BIOENGINEERING-BASEL(2024)
摘要
Single-view cone-beam X-ray luminescence computed tomography (CB-XLCT) has recently gained attention as a highly promising imaging technique that allows for the efficient and rapid three-dimensional visualization of nanophosphor (NP) distributions in small animals. However, the reconstruction performance is hindered by the ill-posed nature of the inverse problem and the effects of depth variation as only a single view is acquired. To tackle this issue, we present a methodology that integrates an automated restarting strategy with depth compensation to achieve reconstruction. The present study employs a fast proximal gradient descent (FPGD) method, incorporating L0 norm regularization, to achieve efficient reconstruction with accelerated convergence. The proposed approach offers the benefit of retrieving neighboring multitarget distributions without the need for CT priors. Additionally, the automated restarting strategy ensures reliable reconstructions without the need for manual intervention. Numerical simulations and physical phantom experiments were conducted using a custom CB-XLCT system to demonstrate the accuracy of the proposed method in resolving adjacent NPs. The results showed that this method had the lowest relative error compared to other few-view techniques. This study signifies a significant progression in the development of practical single-view CB-XLCT for high-resolution 3-D biomedical imaging.
更多查看译文
关键词
image reconstruction,X-ray luminescence computed tomography,optical tomography,ill-posed
AI 理解论文
溯源树
样例
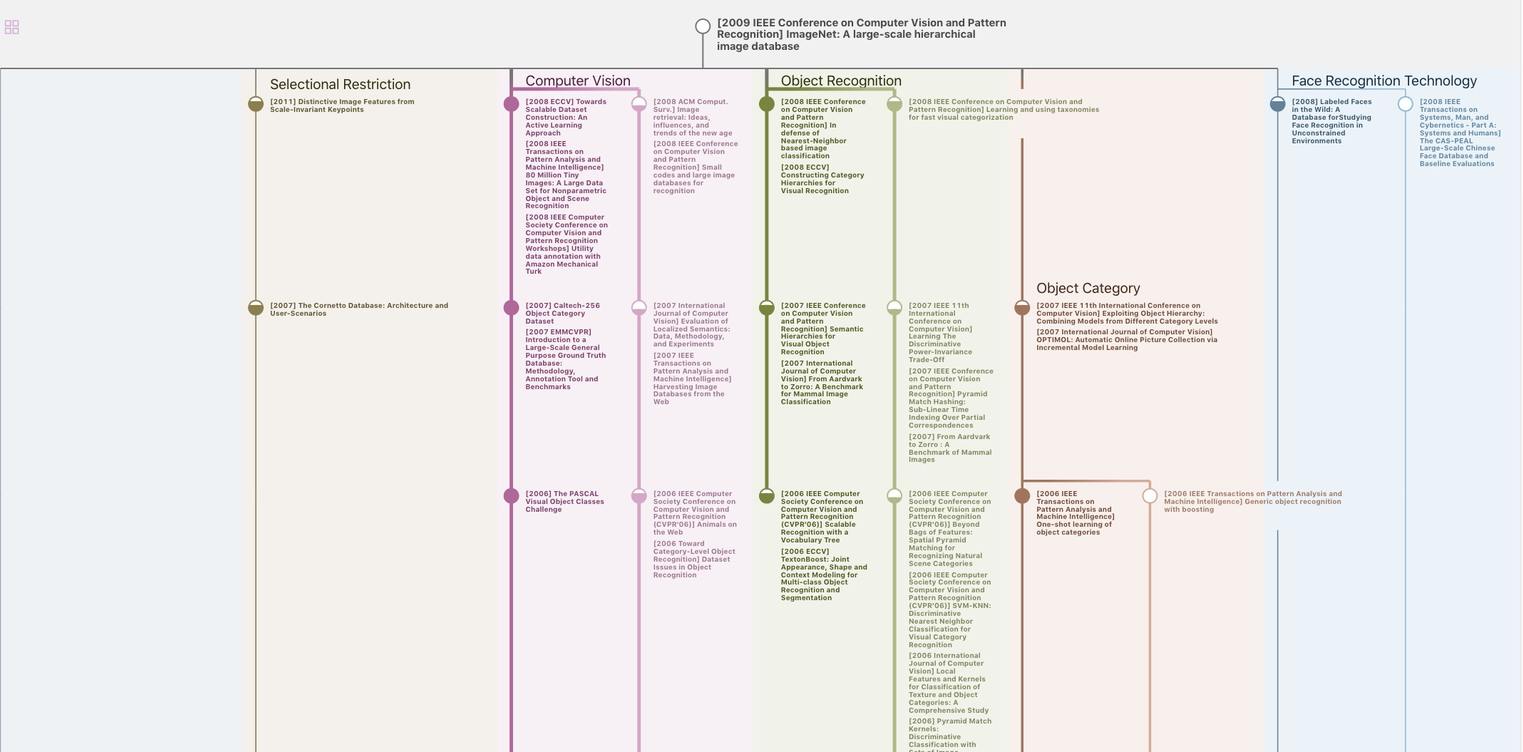
生成溯源树,研究论文发展脉络
Chat Paper
正在生成论文摘要