A Hybrid Approach for Improving the Classification Performance in Imbalanced Dataset
2023 3rd International Conference on Mobile Networks and Wireless Communications (ICMNWC)(2023)
摘要
Imbalanced data classification plays a vital role in reality. Various approaches have been applied to improve the efficiency of this classification problem. In this paper, we illustrate a hybrid approach MASI based on resampling samples idea to deal with the imbalanced data classification. Furthermore, in our proposal, the data density distribution and the nearest k-nearest neighbor (k-NN) in each subset are the important criteria in the selecting the number of samples in the majority class need to be relabeled into the minority sample's label. So, we use the k-NN classifier to evaluate the classification performance. The experimental results on ten imbalanced datasets from UCI repository illustrated that our algorithm achieves significantly in G-mean and F-measure values than other approaches like original, Random Over-sampling (ROS), Random Under-sampling (RUS), SMOTE, and Borderline-Smote (BSMOTE1).
更多查看译文
关键词
Density distribution,Imbalanced data,k-nearest neighbor,classification performance,UCI repository
AI 理解论文
溯源树
样例
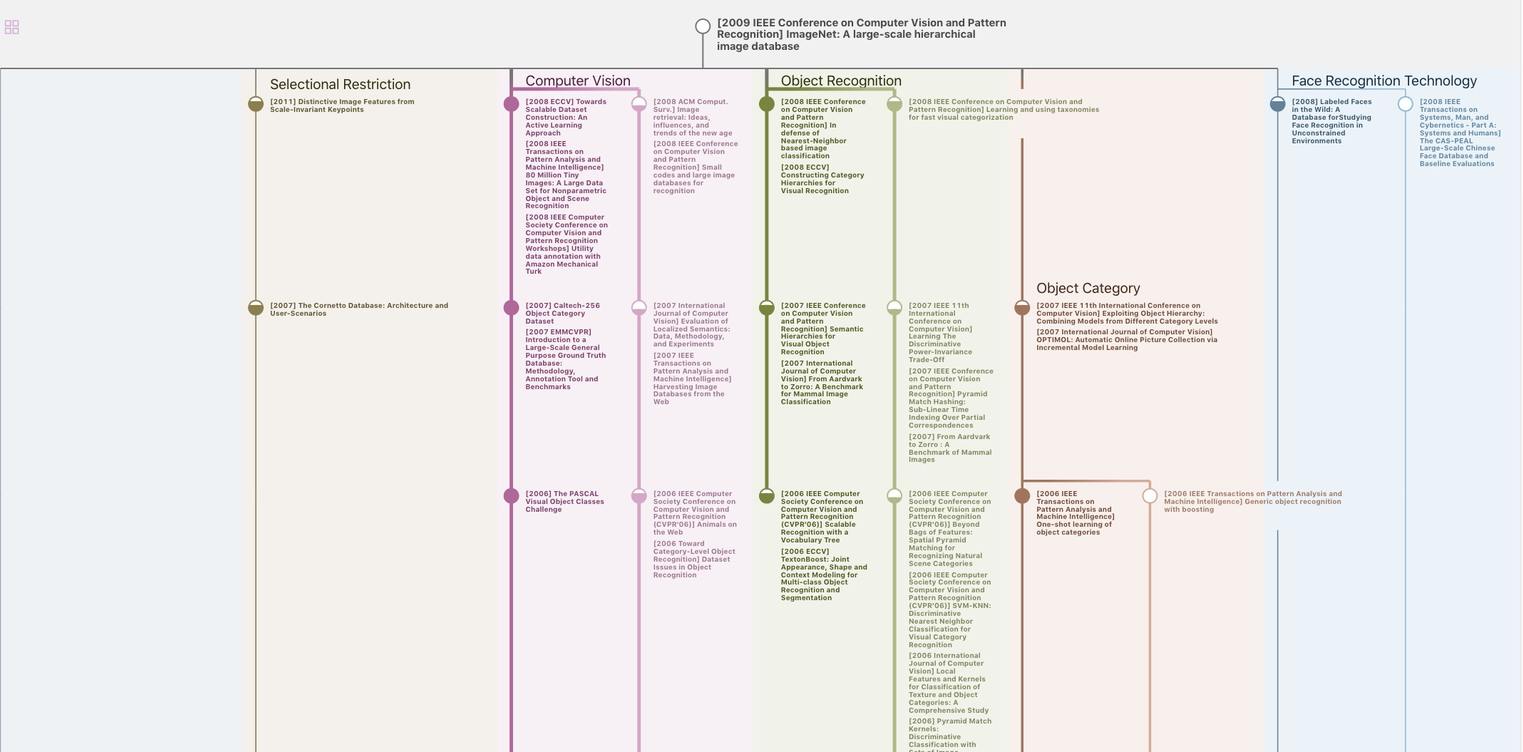
生成溯源树,研究论文发展脉络
Chat Paper
正在生成论文摘要