A Bus Privacy Preserving Decentralized Power Flow Algorithm Considering Neighbor Partial Derivative Information
IEEE ACCESS(2024)
摘要
To ensure the privacy of power system users while maximizing the efficiency of decentralized computational resources, this paper presents a novel decentralized power flow algorithm. Its core focus lies in preserving bus privacy through the integration of neighboring partial derivative information. Initially, the algorithm utilizes partial derivative data from adjacent buses to formulate a bus-level voltage iterative function, rooted in the bus power balance equation. Each bus in the power network is treated as an individual computational unit managed by an agent. This process involves scanning the network's buses, computing estimated bus voltage values using a designated iteration formula, and iterating until convergence is achieved. Subsequent utilization of the latest neighbor information accelerates this iterative process. To fortify the algorithm's reliability, a strategy assessing local convergence, utilizing the Jacobian matrix at the solution's convergence, is proposed. The simulation results across various IEEE test systems unequivocally validate the proposed algorithm's efficacy in power flow computation, showcasing accelerated speed and robust anti-interference capabilities. Compared to the traditional decentralized power flow method at the bus level, this algorithm significantly optimizes the iterative process, slashing the number of iterations by a minimum of 1.36 times. Notably, this efficiency boost amplifies in larger system scales, boasting an impressive reduction of up to 9.21 times fewer iterations.
更多查看译文
关键词
Decentralized power flow,privacy preservation,voltage iterative function,local convergence
AI 理解论文
溯源树
样例
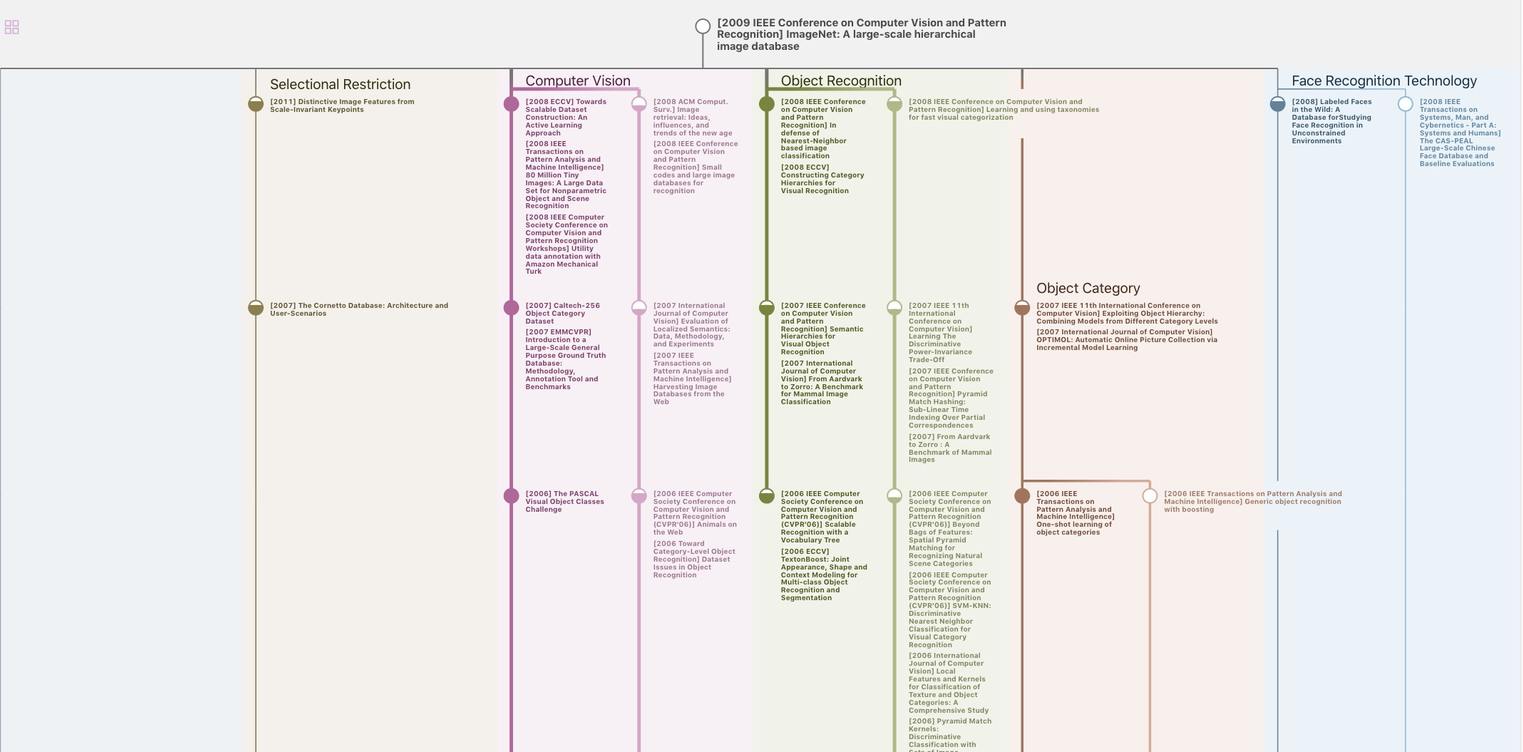
生成溯源树,研究论文发展脉络
Chat Paper
正在生成论文摘要