Creating semi-Quanta multi-layer synthetic CNT images using CycleGAN.
Applied Imagery Pattern Recognition Workshop(2023)
摘要
We address the challenge of creating semi-Quanta images of vertically-oriented carbon nanotubes (CNT). Our previous method, CNTNeXt, successfully predicted the mechanical properties for semi-Scanning Electron Microscopy (SEM) images of CNT forests. However, for Quanta images, a more sophisticated approach is required. We propose a novel pipeline that modifies multi-layer synthetic (MLS) CNT images, which are synthetic representations of CNT structures, to resemble Quanta images. This is achieved using CycleGAN, a deep learning model designed for image-to-image translation tasks, which can learn mappings between two different domains without paired training data. By training the CycleGAN on both Quanta and MLS images, we are able to transfer the texture of Quanta images to the MLS images. The resulting ’semi-Quanta’ images are ready to train CNTNeXt, which effectively predicts the mechanical properties, including buckling load and stiffness of synthetic MLS images. Our method represents an advancement towards our ultimate goal of creating an automated CNT growth process, and opens up possibilities for further improvements and applications.
更多查看译文
关键词
CNTNeXt,CycleGAN,Semi-Quanta multi-layer images,Mechanical Properties
AI 理解论文
溯源树
样例
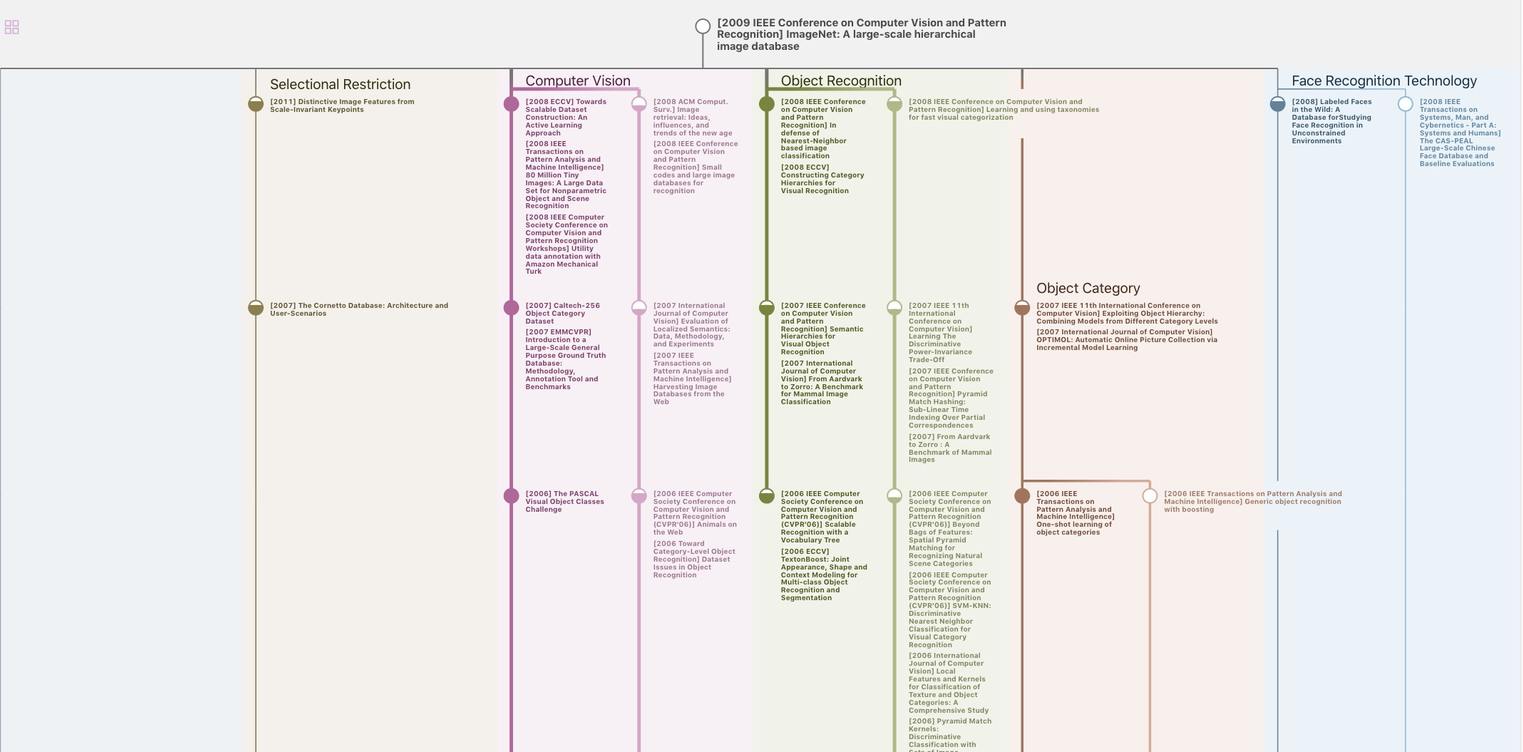
生成溯源树,研究论文发展脉络
Chat Paper
正在生成论文摘要