Approach for Automatic Defect Detection in Aluminum Casting X-Ray Images Using Deep Learning and Gain-Adaptive Multi-Scale Retinex
Journal of Nondestructive Evaluation(2024)
摘要
Nondestructive testing (NDT) plays a vital role in the production and quality control of the casting process. Due to the complexity of inspection procedures and the extensive scale of mass production, it becomes imperative to develop fast and precise automatic detection methods. This paper introduces a deep learning-based approach for detecting defects in X-ray images of aluminum castings. Firstly, we introduce the Gain-Adaptive Multi-Scale Retinex (GAMSR) algorithm, which is designed to enhance the low-contrast and noisy X-ray raw data. To address the problem of minor blowhole defects being overlooked during detections, we combine the Feature Pyramid Network (FPN) with the Convolutional Block Attention Module (CBAM) to extract high-level semantic information from the X-ray images. It can also promote the feature extraction network to focus more on the casting defect features. Furthermore, we employ Weighted Region of Interest pooling (W-RoI pooling) in place of RoIAlign. This strategy eliminates area misalignment and significantly enhances the precision of defect identification. Experiment results demonstrate that the proposed approaches can improve the performance of defect detection for aluminum casting DR images, with the accuracy increasing by 20.08%.
更多查看译文
关键词
Aluminum casting X-ray image,Defect detection,Image enhancement,Attention module,W-RoI pooling
AI 理解论文
溯源树
样例
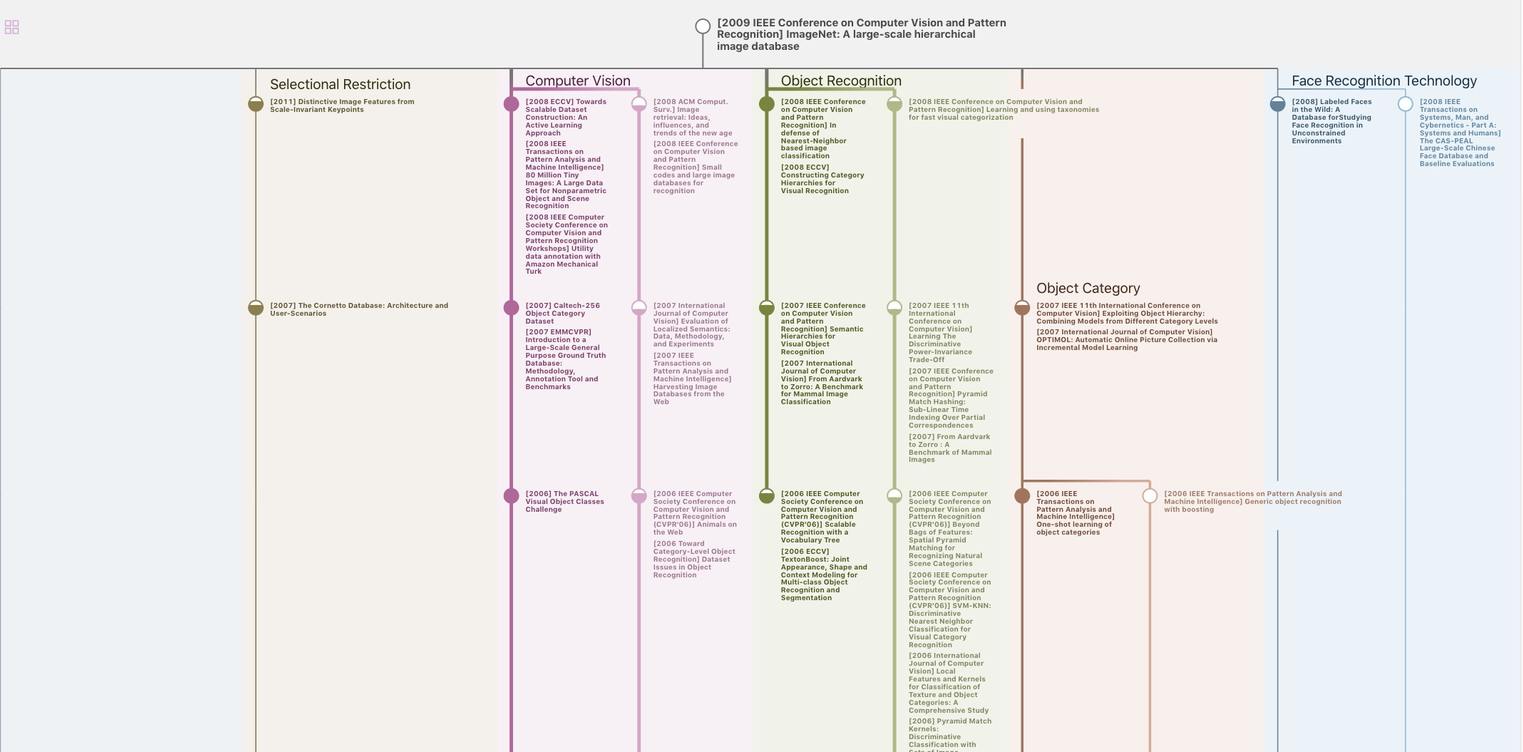
生成溯源树,研究论文发展脉络
Chat Paper
正在生成论文摘要